Query Twice: Dual Mixture Attention Meta Learning for Video Summarization
MM '20: The 28th ACM International Conference on Multimedia Seattle WA USA October, 2020(2020)
摘要
Video summarization aims to select representative frames to retain high-level information, which is usually solved by predicting the segment-wise importance score via a softmax function. However, softmax function suffers in retaining high-rank representations for complex visual or sequential information, which is known as the Softmax Bottleneck problem. In this paper, we propose a novel framework named Dual Mixture Attention (DMASum) model with Meta Learning for video summarization that tackles the softmax bottleneck problem, where the Mixture of Attention layer (MoA) effectively increases the model capacity by employing twice self-query attention that can capture the second-order changes in addition to the initial query-key attention, and a novel Single Frame Meta Learning rule is then introduced to achieve more generalization to small datasets with limited training sources. Furthermore, the DMASum significantly exploits both visual and sequential attention that connects local key-frame and global attention in an accumulative way. We adopt the new evaluation protocol on two public datasets, SumMe, and TVSum. Both qualitative and quantitative experiments manifest significant improvements over the state-of-the-art methods.
更多查看译文
关键词
video summarization, attention network, meta learning
AI 理解论文
溯源树
样例
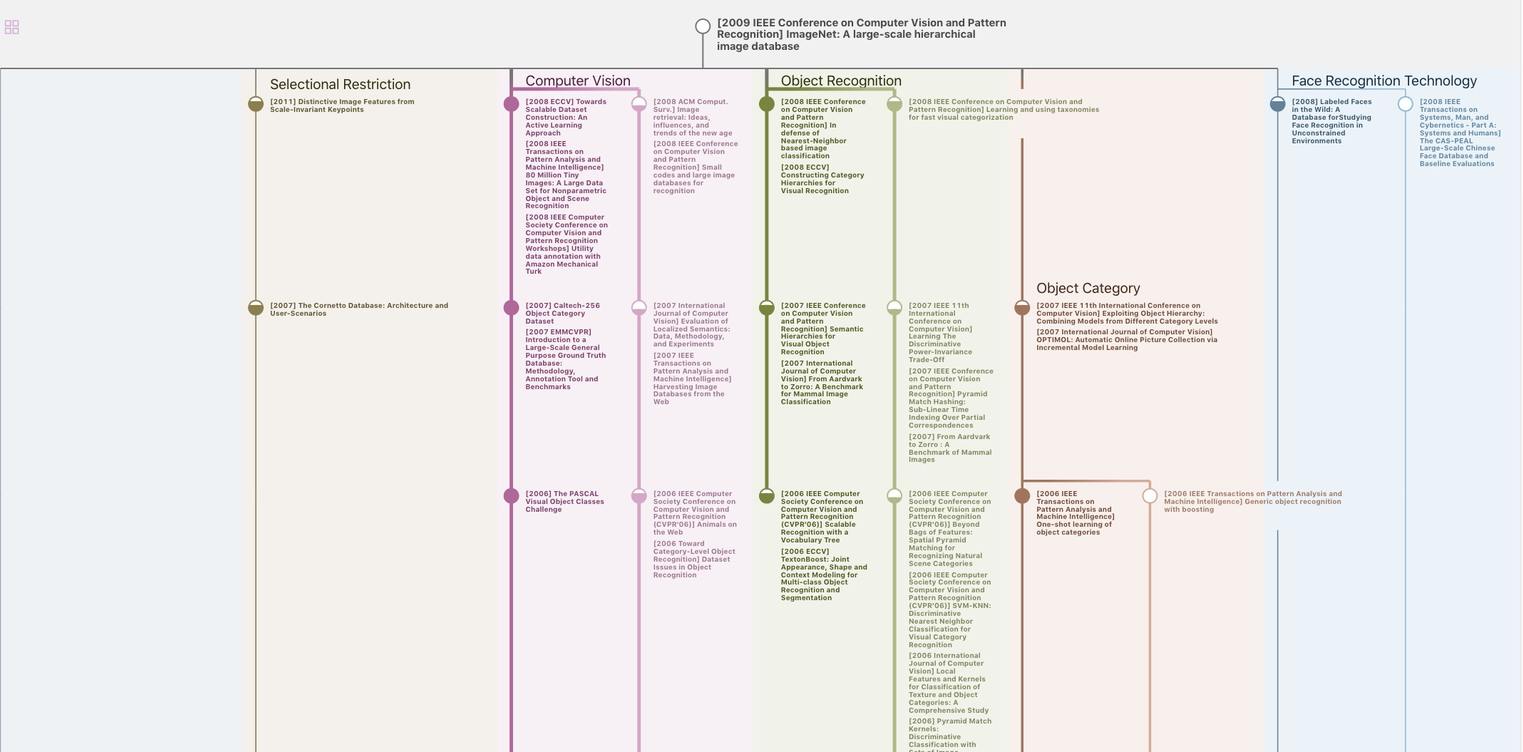
生成溯源树,研究论文发展脉络
Chat Paper
正在生成论文摘要