Particle Filters with Nudging in Multiscale Chaotic Systems: With Application to the Lorenz ’96 Atmospheric Model
Journal of Nonlinear Science(2020)
摘要
This paper presents reduced-order nonlinear filtering schemes based on a theoretical framework that combines stochastic dimensional reduction and nonlinear filtering. Here, dimensional reduction is achieved for estimating the slow-scale process in a multiscale environment by constructing a filter using stochastic averaging results. The nonlinear filter is approximated numerically using the ensemble Kalman filter and particle filter. The particle filter is further adapted to the complexities of inherently chaotic signals. In particle filters, an ensemble of particles is used to represent the distribution of the state of the hidden signal. The ensemble is updated using observation data to obtain the best representation of the conditional density of the true state variables given observations. Particle methods suffer from the “curse of dimensionality,” an issue of particle degeneracy within a sample, which increases exponentially with system dimension. Hence, particle filtering in high dimensions can benefit from some form of dimensional reduction. A control is superimposed on particle dynamics to drive particles to locations most representative of observations, in other words, to construct a better prior density. The control is determined by solving a classical stochastic optimization problem and implemented in the particle filter using importance sampling techniques.
更多查看译文
关键词
Data assimilation,Nonlinear filtering,Homogenization,Particle filter,Ensemble Kalman filter,Nudging,Stochastic optimal control,Lorenz ’96
AI 理解论文
溯源树
样例
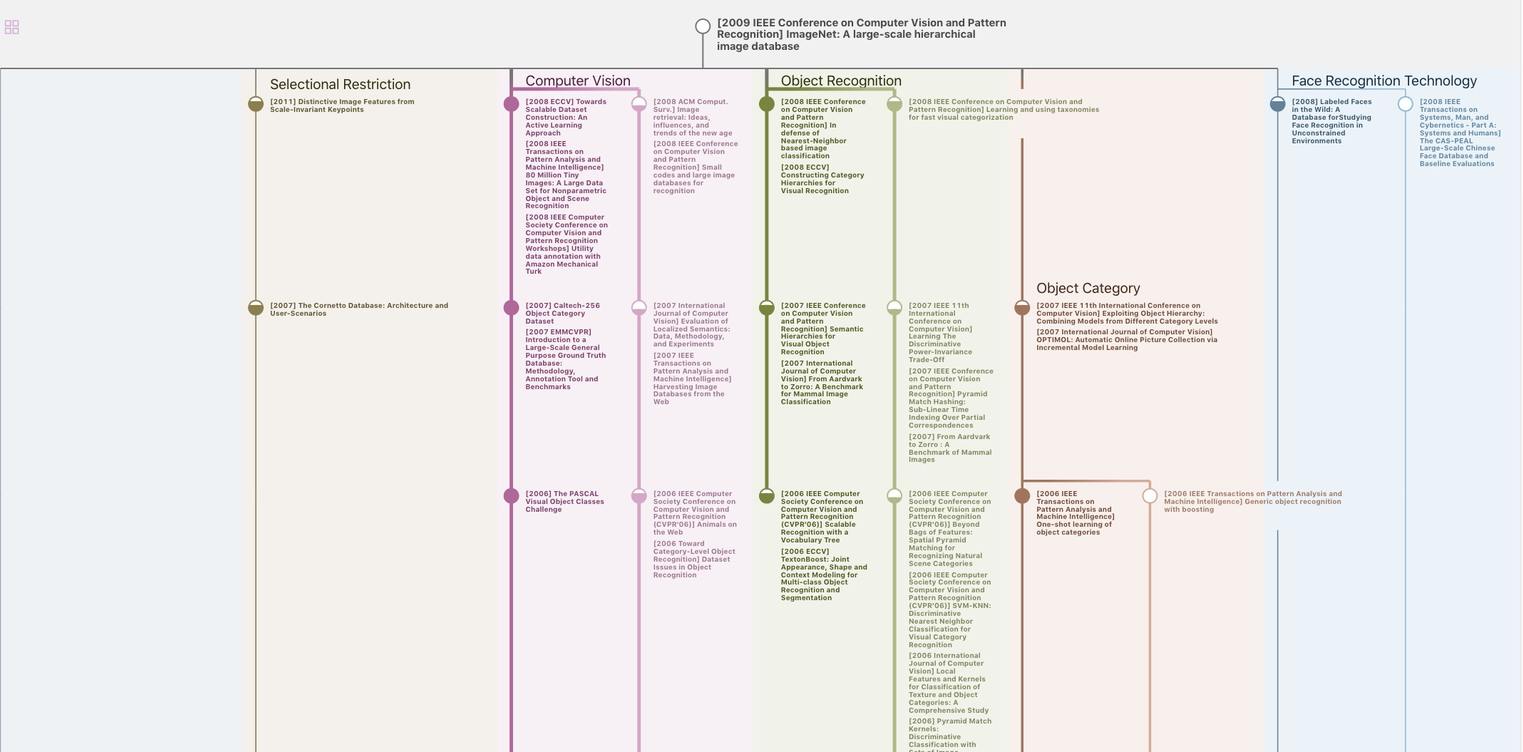
生成溯源树,研究论文发展脉络
Chat Paper
正在生成论文摘要