Quantification of Myocardial Blood Flow by Machine Learning Analysis of Modified Dual Bolus MRI Examination
Annals of biomedical engineering(2020)
摘要
Contrast-enhanced magnetic resonance imaging (MRI) is a promising method for estimating myocardial blood flow (MBF). However, it is often affected by noise from imaging artefacts, such as dark rim artefact obscuring relevant features. Machine learning enables extracting important features from such noisy data and is increasingly applied in areas where traditional approaches are limited. In this study, we investigate the capacity of machine learning, particularly support vector machines (SVM) and random forests (RF), for estimating MBF from tissue impulse response signal in an animal model. Domestic pigs (n = 5) were subjected to contrast enhanced first pass MRI (MRI-FP) and the impulse response at different regions of the myocardium (n = 24/pig) were evaluated at rest (n = 120) and stress (n = 96). Reference MBF was then measured using positron emission tomography (PET). Since the impulse response may include artefacts, classification models based on SVM and RF were developed to discriminate noisy signal. In addition, regression models based on SVM, RF and linear regression (for comparison) were developed for estimating MBF from the impulse response at rest and stress. The classification and regression models were trained on data from 4 pigs (n = 168) and tested on 1 pig (n = 48). Models based on SVM and RF outperformed linear regression, with higher correlation (R SVM 2 = 0.81, R RF 2 = 0.74, R linear_regression 2 = 0.60; ρSVM = 0.76, ρRF = 0.76, ρlinear_regression = 0.71) and lower error (RMSESVM = 0.67 mL/g/min, RMSERF = 0.77 mL/g/min, RMSElinear_regression = 0.96 mL/g/min) for predicting MBF from MRI impulse response signal. Classifier based on SVM was optimal for detecting impulse response signals with artefacts (accuracy = 92%). Modified dual bolus MRI signal, combined with machine learning, has potential for accurately estimating MBF at rest and stress states, even from signals with dark rim artefacts. This could provide a protocol for reliable and easy estimation of MBF, although further research is needed to clinically validate the approach.
更多查看译文
关键词
Magnetic resonance imaging,Myocardial perfusion imaging,Modified dual bolus method,Machine learning,Random forest,Support vector machine
AI 理解论文
溯源树
样例
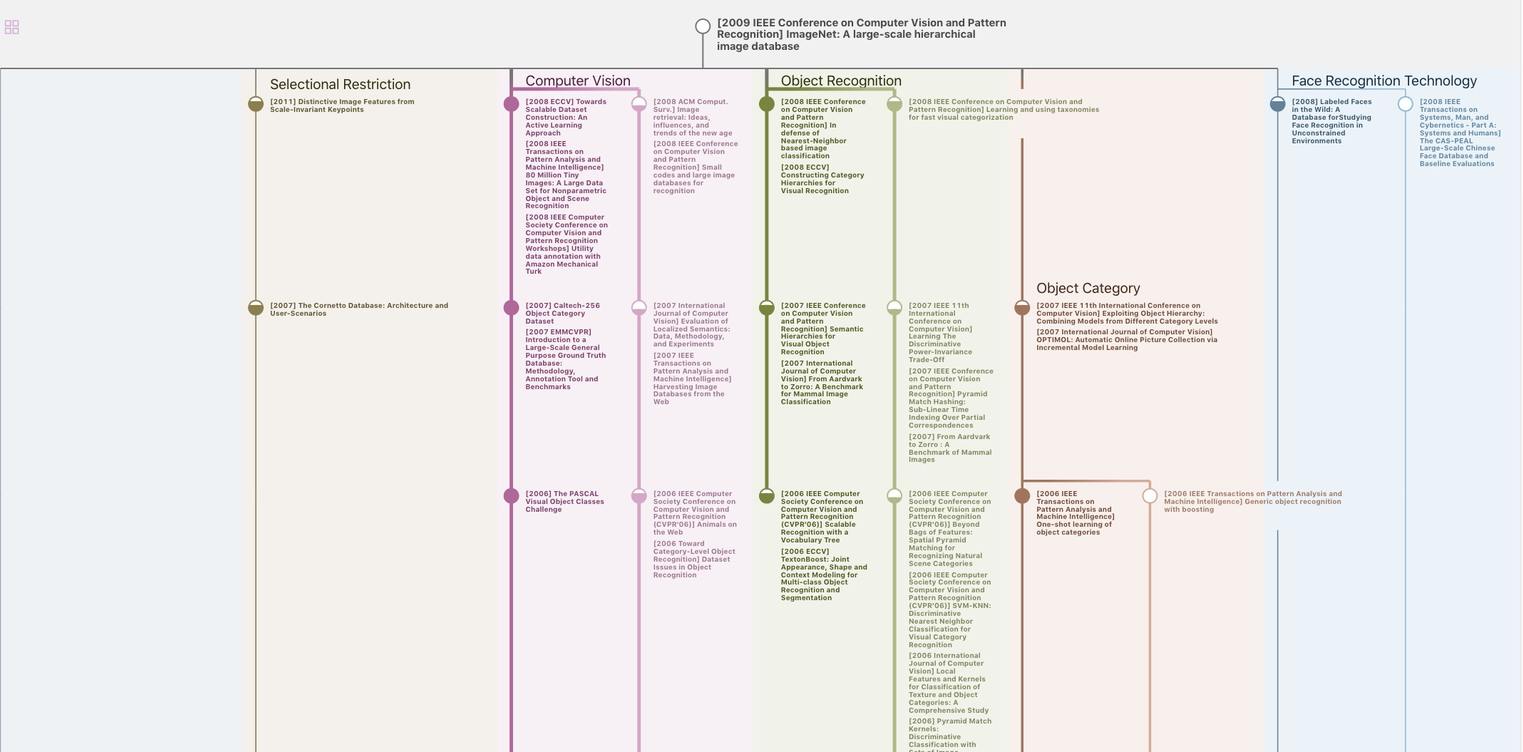
生成溯源树,研究论文发展脉络
Chat Paper
正在生成论文摘要