Real-Time Burn Depth Assessment Using Artificial Networks: A Large-Scale, Multicentre Study
BURNS(2020)
摘要
Introduction: Early judgment of the depth of burns is very important for the accurate formulation of treatment plans. In medical imaging the application of Artificial Intelligence has the potential for serving as a very experienced assistant to improve early clinical diagnosis. Due to lack of large volume of a particular feature, there has been almost no progress in burn field.Methods: 484 early wound images are collected on patients who discharged home after a burn injury in 48 h, from five different levels of hospitals in Hunan Province China. According to actual healing time, all images are manually annotated by five professional burn surgeons and divided into three sets which are shallow(0-10 days), moderate(11-20 days) and deep (more than 21 days or skin graft healing). These ROIs were further divided into 5637 patches sizes 224 x 224 pixels, of which 1733 shallow, 1804 moderate, and 2100 deep. We used transfer learning suing a Pre-trained ResNet50 model and the ratio of all images is 7:1.5:1.5 for training:validation:test.Results: A novel artificial burn depth recognition model based on convolutional neural network was established and the diagnostic accuracy of the three types of burns is about 80%.Discussion: The actual healing time can be used to deduce the depth of burn involvement. The artificial burn depth recognition model can accurately infer healing time and burn depth of the patient, which is expected to be used for auxiliary diagnosis improvement. (C) 2020 Elsevier Ltd and ISBI. All rights reserved.
更多查看译文
关键词
Burn depth assessment, Convolutional neural networks, Classification, Medical imaging
AI 理解论文
溯源树
样例
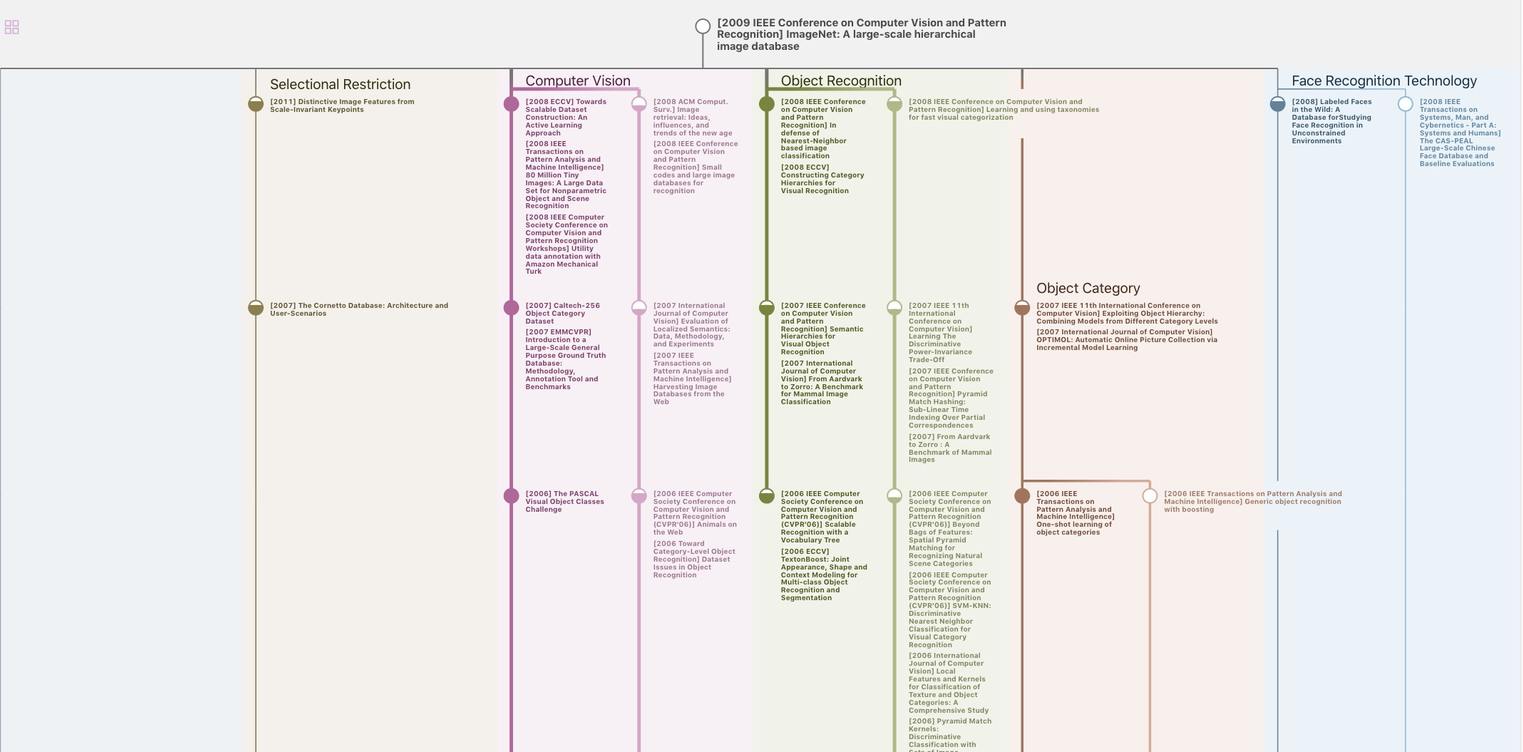
生成溯源树,研究论文发展脉络
Chat Paper
正在生成论文摘要