On Gap Based Lower Bounding Techniques for Best Arm Identification
Entropy(2020)
摘要
In this paper, we consider techniques for establishing lower bounds on the number of arm pulls for best-arm identification in the multi-armed bandit problem. While a recent divergence-based approach was shown to provide improvements over an older gap-based approach, we show that the latter can be refined to match the former (up to constant factors) in many cases of interest under Bernoulli rewards, including the case that the rewards are bounded away from zero and one. Together with existing upper bounds, this indicates that the divergence-based and gap-based approaches are both effective for establishing sample complexity lower bounds for best-arm identification.
更多查看译文
关键词
multi-armed bandits,best-arm identification,information-theoretic lower bounds,PAC learning
AI 理解论文
溯源树
样例
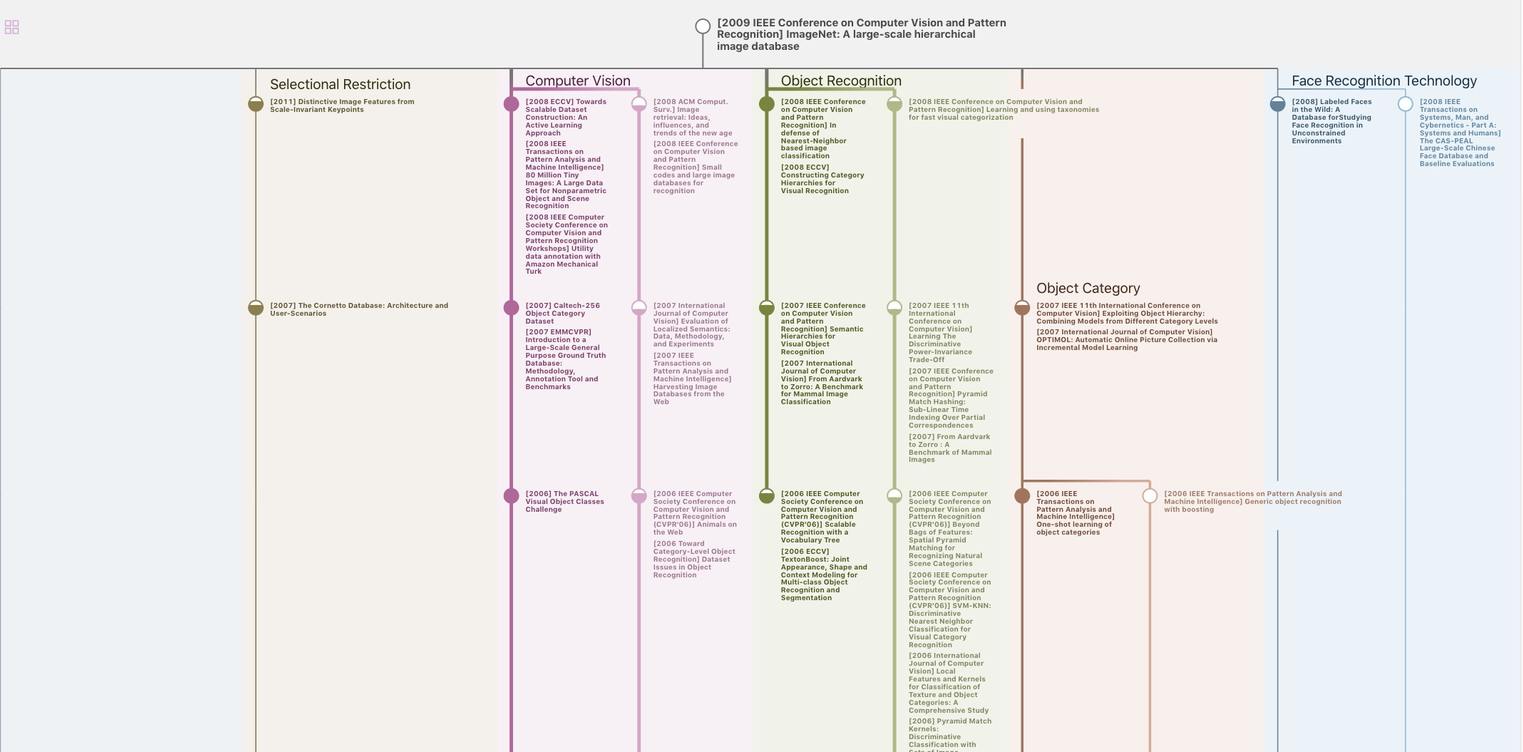
生成溯源树,研究论文发展脉络
Chat Paper
正在生成论文摘要