Learning Domain-invariant Graph for Adaptive Semi-supervised Domain Adaptation with Few Labeled Source Samples.
CoRR(2020)
摘要
Domain adaptation aims to generalize a model from a source domain to tackle tasks in a related but different target domain. Traditional domain adaptation algorithms assume that enough labeled data, which are treated as the prior knowledge are available in the source domain. However, these algorithms will be infeasible when only a few labeled data exist in the source domain, and thus the performance decreases significantly. To address this challenge, we propose a Domain-invariant Graph Learning (DGL) approach for domain adaptation with only a few labeled source samples. Firstly, DGL introduces the Nystrom method to construct a plastic graph that shares similar geometric property as the target domain. And then, DGL flexibly employs the Nystrom approximation error to measure the divergence between plastic graph and source graph to formalize the distribution mismatch from the geometric perspective. Through minimizing the approximation error, DGL learns a domain-invariant geometric graph to bridge source and target domains. Finally, we integrate the learned domain-invariant graph with the semi-supervised learning and further propose an adaptive semi-supervised model to handle the cross-domain problems. The results of extensive experiments on popular datasets verify the superiority of DGL, especially when only a few labeled source samples are available.
更多查看译文
关键词
adaptation,learning,domain-invariant,semi-supervised
AI 理解论文
溯源树
样例
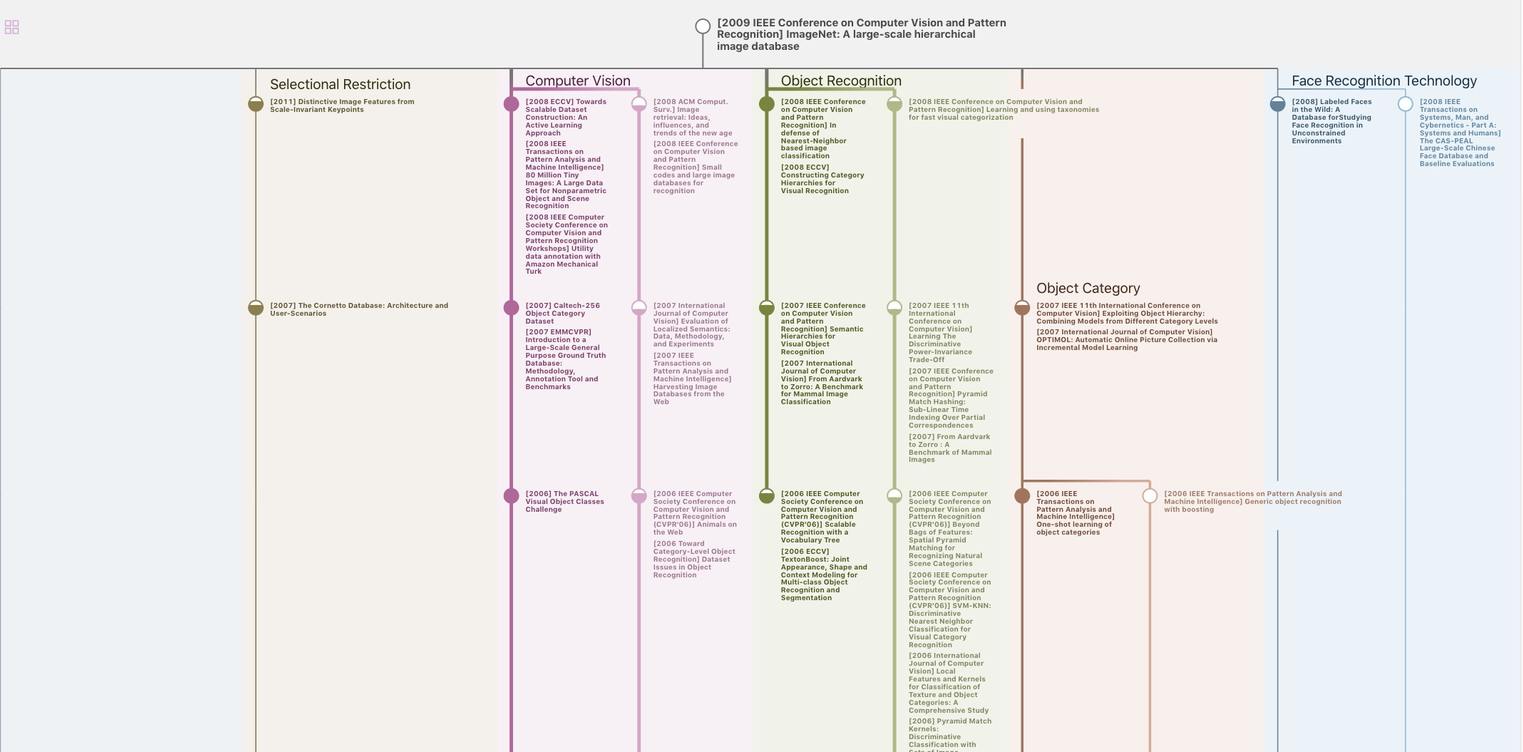
生成溯源树,研究论文发展脉络
Chat Paper
正在生成论文摘要