Unifying framework for strong and fragile liquids via machine learning: a study of liquid silica
arxiv(2020)
摘要
The fragility of a glassforming liquid characterizes how rapidly its relaxation dynamics slow down with cooling. The viscosity of strong liquids follows an Arrhenius law with a temperature-independent barrier height to rearrangements responsible for relaxation, whereas fragile liquids experience a much faster increase in their dynamics, suggesting a barrier height that increases with decreasing temperature. Strong glassformers are typically network glasses, while fragile glassformers are typically molecular or hard-sphere-like. As a result of these differences at the microscopic level, strong and fragile glassformers are usually treated separately from a theoretical point of view. Silica is the archetypal strong glassformer at low temperatures, but also exhibits a mysterious strong-to-fragile crossover at higher temperatures. Here we show that softness, a structure-based machine learned parameter that has previously been applied to fragile glassformers provides a useful description of model liquid silica in the strong and fragile regimes, and through the strong-to-fragile crossover. Just as for fragile glassformers, the relationship between softness and dynamics is invariant and Arrhenius in all regimes, but the average softness changes with temperature. The strong-to-fragile crossover in silica is not due to a sudden, qualitative change in structure, but can be explained by a simple Arrhenius form with a continuously and linearly changing local structure. Our results unify the study of liquid silica under a single simple conceptual picture.
更多查看译文
关键词
fragile liquids,silica,machine learning
AI 理解论文
溯源树
样例
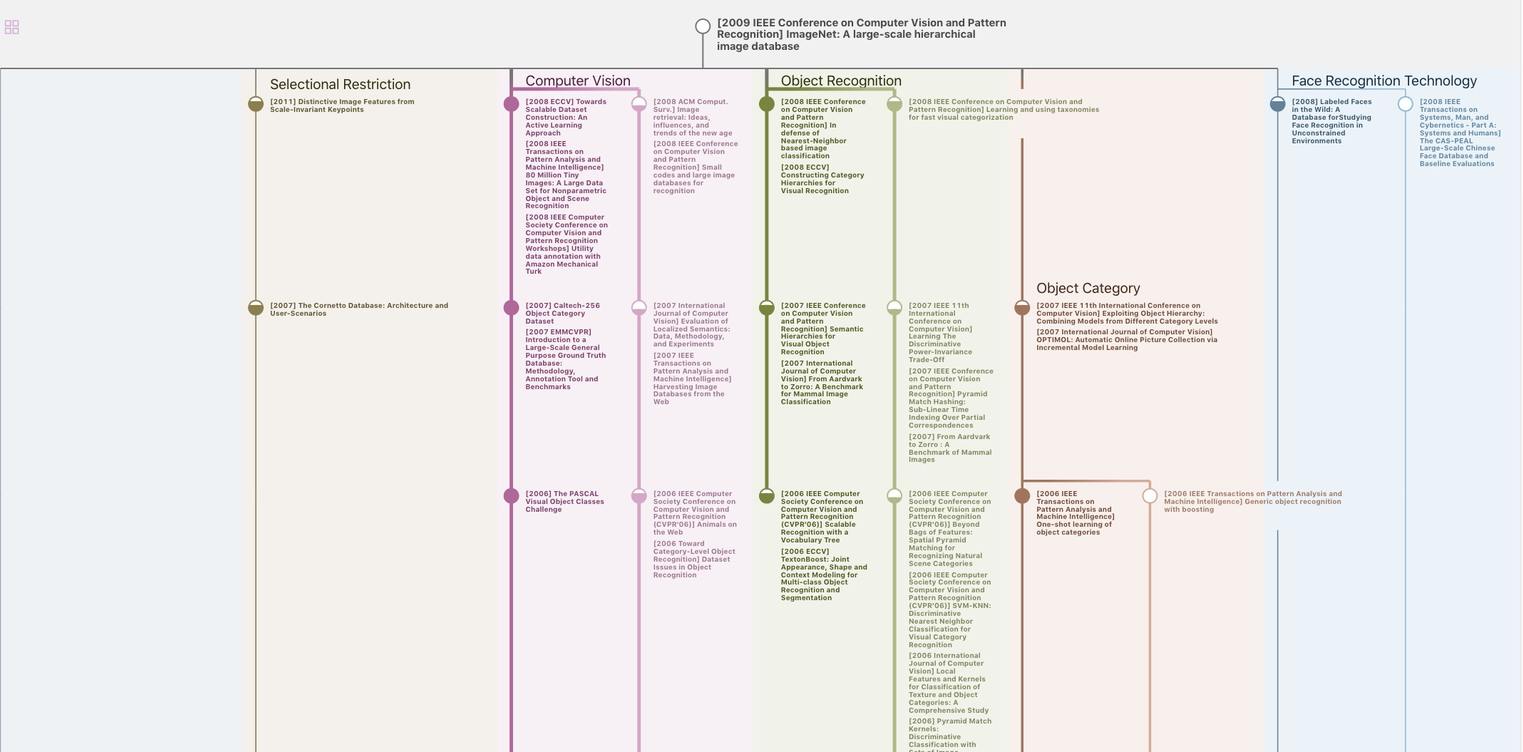
生成溯源树,研究论文发展脉络
Chat Paper
正在生成论文摘要