A Multimodal Framework for Large-Scale Emotion Recognition by Fusing Music and Electrodermal Activity Signals
ACM Transactions on Multimedia Computing, Communications, and Applications(2022)
摘要
AbstractConsiderable attention has been paid to physiological signal-based emotion recognition in the field of affective computing. For reliability and user-friendly acquisition, electrodermal activity (EDA) has a great advantage in practical applications. However, EDA-based emotion recognition with large-scale subjects is still a tough problem. The traditional well-designed classifiers with hand-crafted features produce poorer results because of their limited representation abilities. And the deep learning models with auto feature extraction suffer the overfitting drop-off because of large-scale individual differences. Since music has a strong correlation with human emotion, static music can be involved as the external benchmark to constrain various dynamic EDA signals. In this article, we make an attempt by fusing the subject’s individual EDA features and the external evoked music features. And we propose an end-to-end multimodal framework, the one-dimensional residual temporal and channel attention network (RTCAN-1D). For EDA features, the channel-temporal attention mechanism for EDA-based emotion recognition is first involved in mine the temporal and channel-wise dynamic and steady features. The comparisons with single EDA-based SOTA models on DEAP and AMIGOS datasets prove the effectiveness of RTCAN-1D to mine EDA features. For music features, we simply process the music signal with the open-source toolkit openSMILE to obtain external feature vectors. We conducted systematic and extensive evaluations. The experiments on the current largest music emotion dataset PMEmo validate that the fusion of EDA and music is a reliable and efficient solution for large-scale emotion recognition.
更多查看译文
关键词
Multimodal fusion, large-scale emotion recognition, attention mechanism
AI 理解论文
溯源树
样例
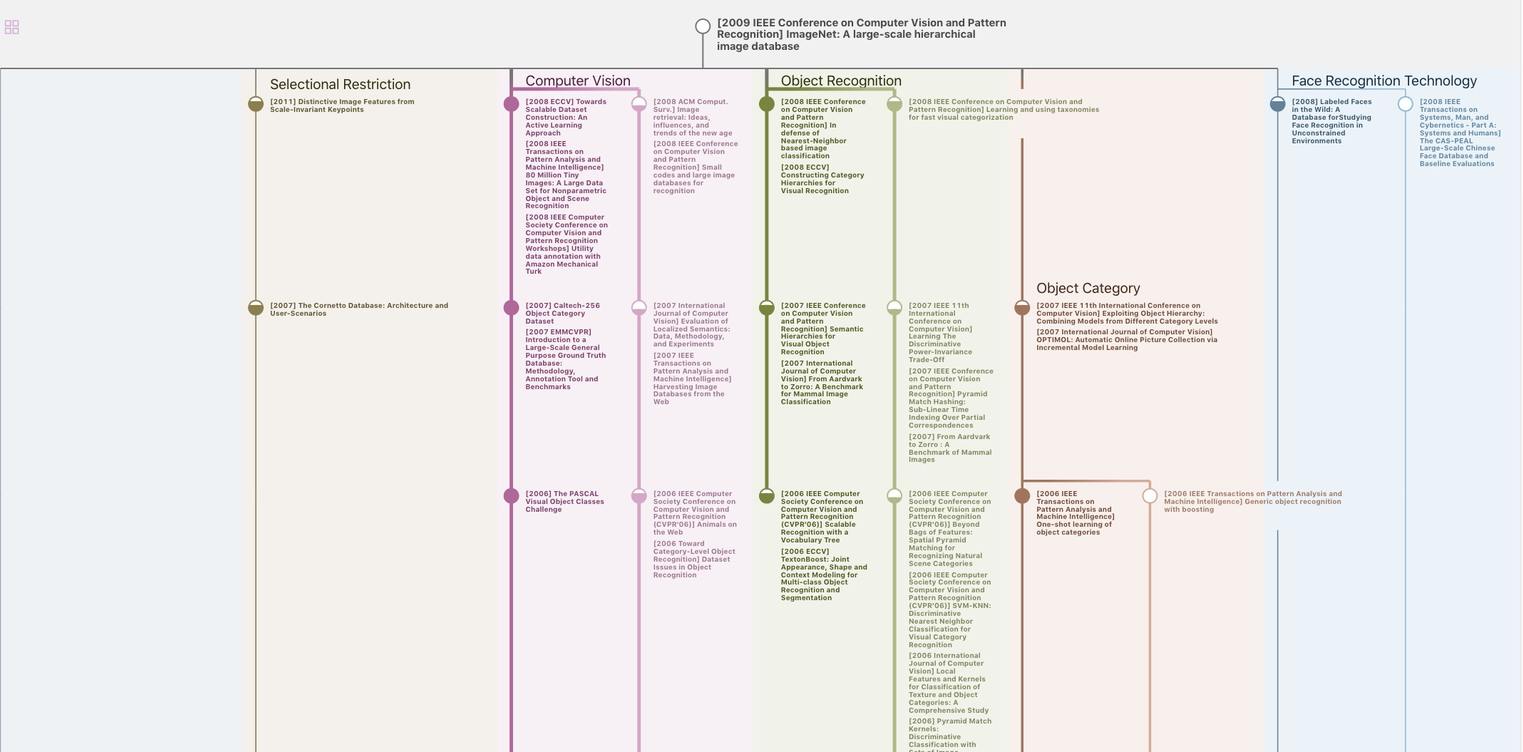
生成溯源树,研究论文发展脉络
Chat Paper
正在生成论文摘要