Variational Autoencoder for Anti-Cancer Drug Response Prediction
arxiv(2020)
摘要
There is remarkable progress in identifying the perplexity of genomic landscape of cancer over the past two decades, providing us with huge information resources on anti-cancer drugs. In this work, we seek to predict the response and efficacy for different anti-cancer drugs on breast cancer and generalize it on pan-cancer by using deep learning models. In order to get the result without dealing with enormous amount of unlabeled data, we propose unsupervised variational autoencoder models geneVAE and rectified junction tree variational autoencoder(JTVAE) to extract important features of the data as input to our drug prediction network, which is a multi-layer perceptron(MLP). We take support vector machine regressor(SVR) and single MLP model as our baseline models. The result show that geneVAE and JTVAE are robust and has an average coefficient of determination ($R^{2}$ > 0.84) when predicting drug response on breast cancer cell lines and pan-cancer cell lines while baseline models have an average ($R^{2}$ < 0.7). We discuss about our models' robustness. We use latent vectors to showcase similarity of drug structures by functional groups and assume its uniqueness by mutual prediction between similar drugs.
更多查看译文
AI 理解论文
溯源树
样例
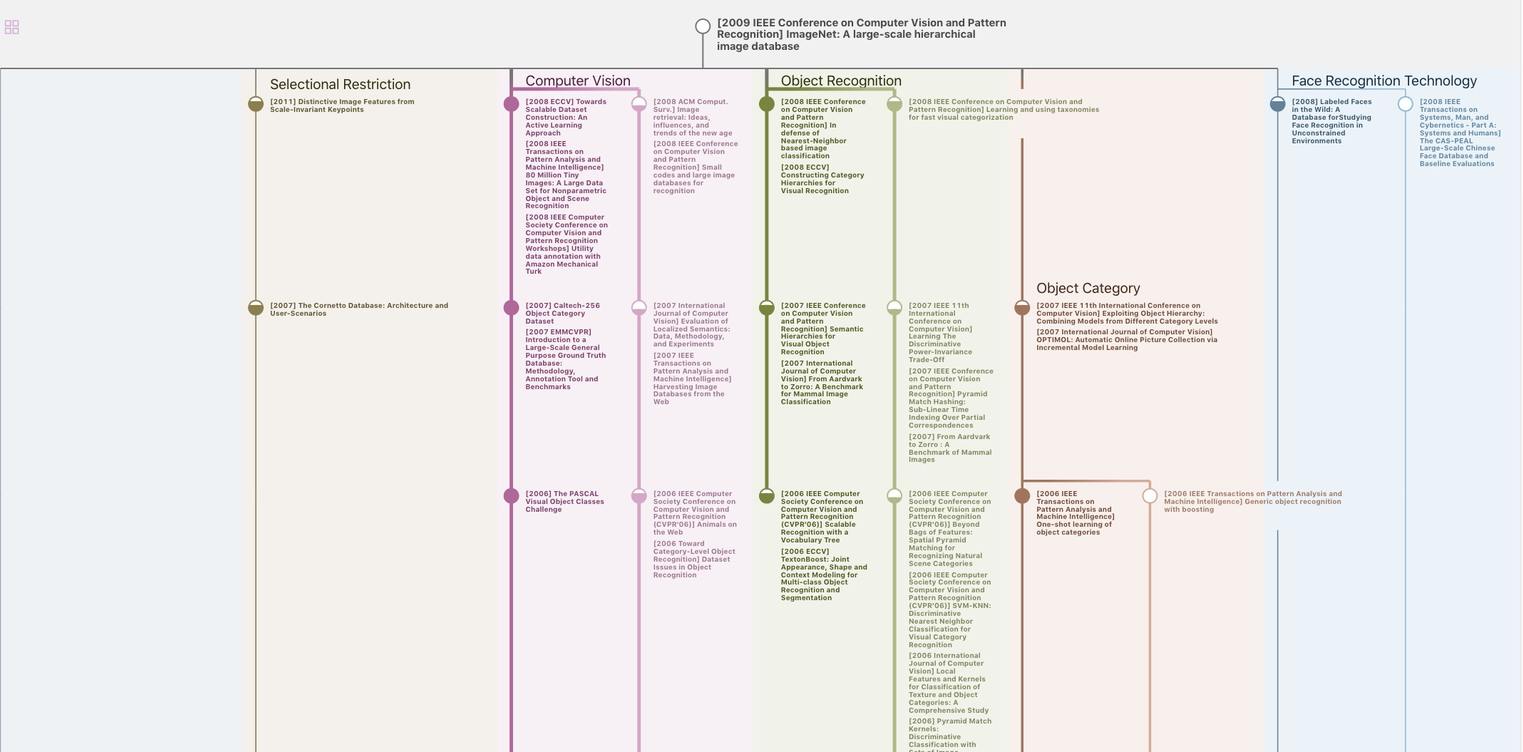
生成溯源树,研究论文发展脉络
Chat Paper
正在生成论文摘要