One Weight Bitwidth to Rule Them All
ECCV Workshops(2020)
摘要
Weight quantization for deep ConvNets has shown promising results for applications such as image classification and semantic segmentation and is especially important for applications where memory storage is limited. However, when aiming for quantization without accuracy degradation, different tasks may end up with different bitwidths. This creates complexity for software and hardware support and the complexity accumulates when one considers mixed-precision quantization, in which case each layer's weights use a different bitwidth. Our key insight is that optimizing for the least bitwidth subject to no accuracy degradation is not necessarily an optimal strategy. This is because one cannot decide optimality between two bitwidths if one has a smaller model size while the other has better accuracy. In this work, we take the first step to understand if some weight bitwidth is better than others by aligning all to the same model size using a width-multiplier. Under this setting, somewhat surprisingly, we show that using a single bitwidth for the whole network can achieve better accuracy compared to mixed-precision quantization targeting zero accuracy degradation when both have the same model size. In particular, our results suggest that when the number of channels becomes a target hyperparameter, a single weight bitwidth throughout the network shows superior results for model compression.
更多查看译文
关键词
Model compression,Deep learning architectures,Quantization,ConvNets,Image classification
AI 理解论文
溯源树
样例
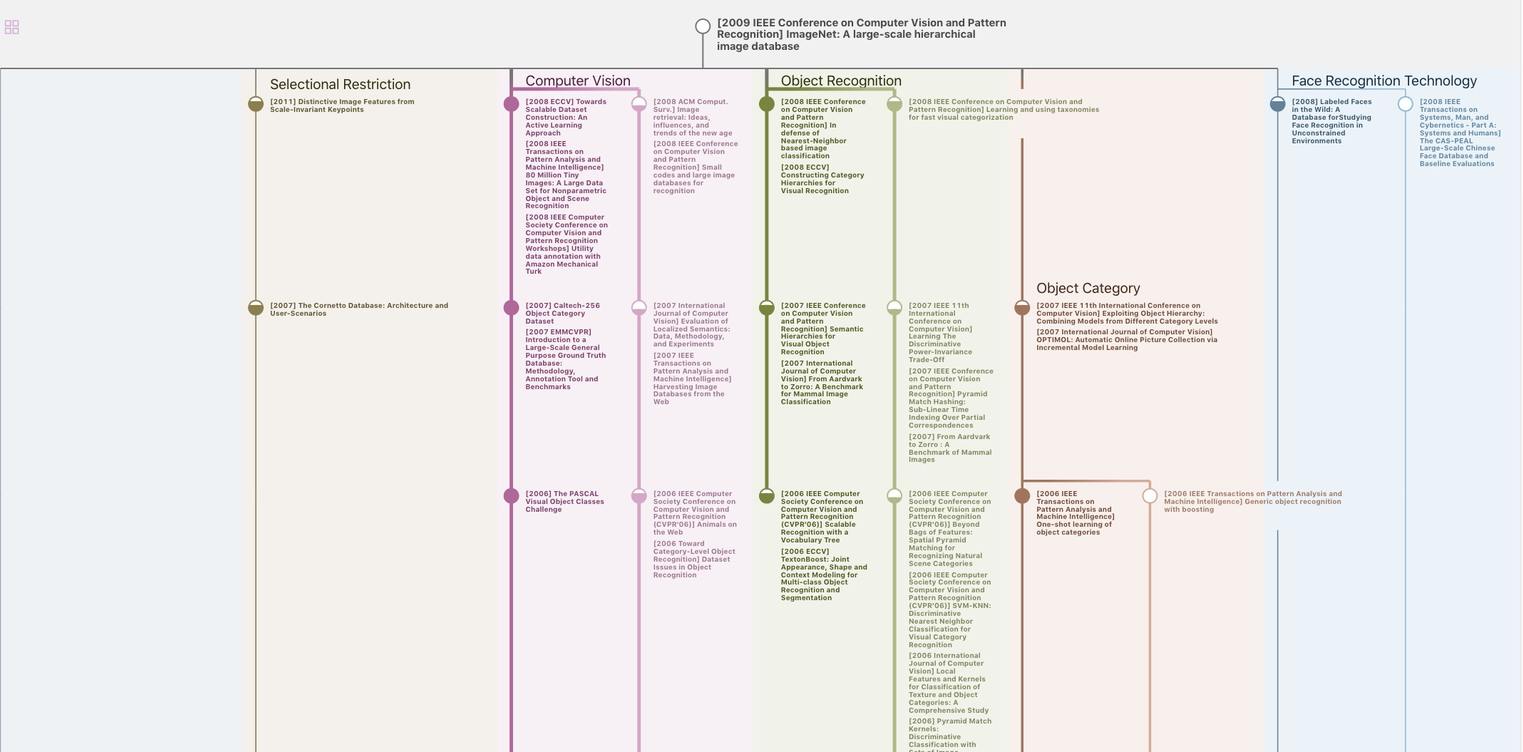
生成溯源树,研究论文发展脉络
Chat Paper
正在生成论文摘要