Single-Timescale Stochastic Nonconvex-Concave Optimization for Smooth Nonlinear TD Learning
Unknown Journal(2020)
摘要
Temporal-Difference (TD) learning with nonlinear smooth function approximation for policy evaluation has achieved great success in modern reinforcement learning. It is shown that such a problem can be reformulated as a stochastic nonconvex-strongly-concave optimization problem, which is challenging as naive stochastic gradient descent-ascent algorithm suffers from slow convergence. Existing approaches for this problem are based on two-timescale or double-loop stochastic gradient algorithms, which may also require sampling large-batch data. However, in practice, a single-timescale single-loop stochastic algorithm is preferred due to its simplicity and also because its step-size is easier to tune. In this paper, we propose two single-timescale single-loop algorithms which require only one data point each step. Our first algorithm implements momentum updates on both primal and dual variables achieving an $O(\varepsilon^{-4})$ sample complexity, which shows the important role of momentum in obtaining a single-timescale algorithm. Our second algorithm improves upon the first one by applying variance reduction on top of momentum, which matches the best known $O(\varepsilon^{-3})$ sample complexity in existing works. Furthermore, our variance-reduction algorithm does not require a large-batch checkpoint. Moreover, our theoretical results for both algorithms are expressed in a tighter form of simultaneous primal and dual side convergence.
更多查看译文
关键词
nonlinear td learning,optimization,single-timescale,nonconvex-concave
AI 理解论文
溯源树
样例
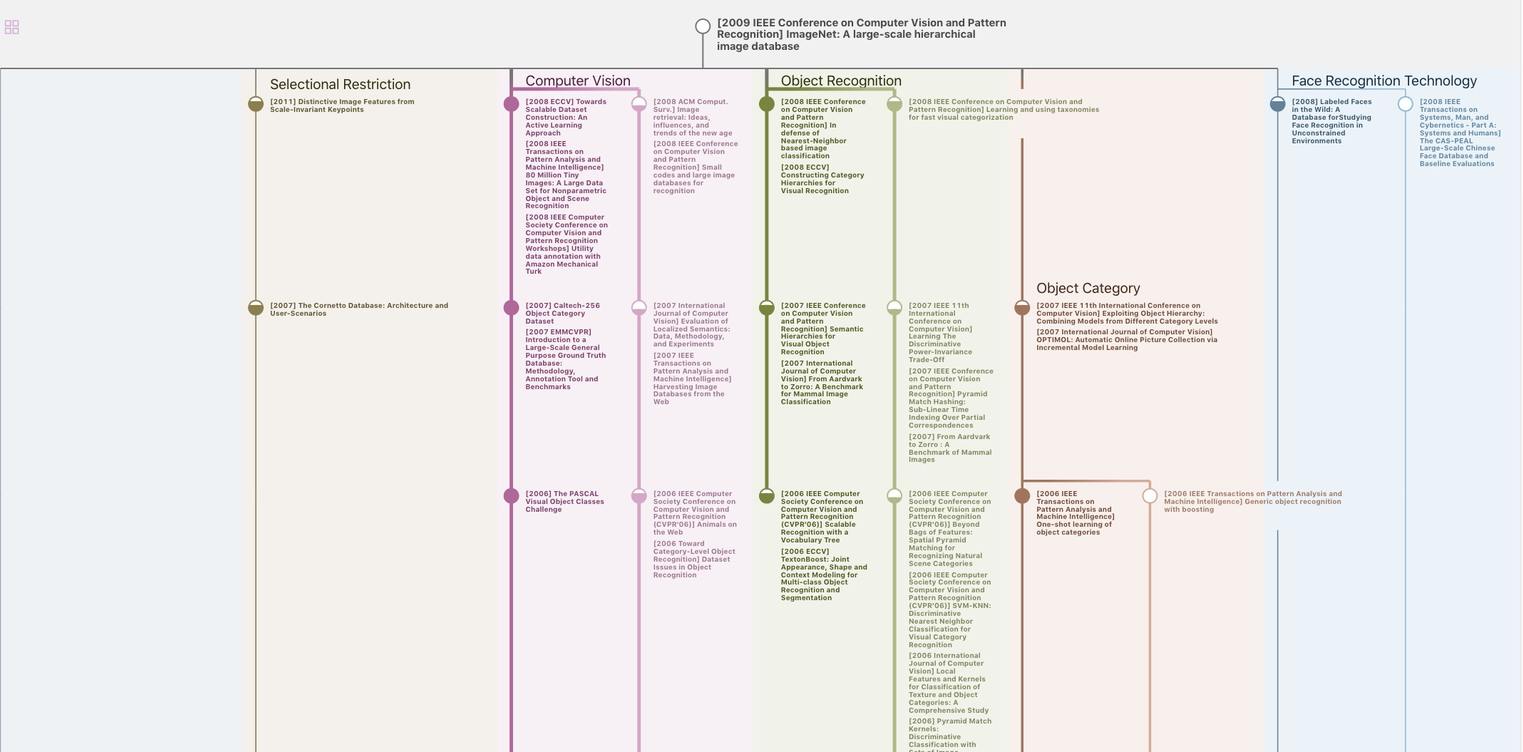
生成溯源树,研究论文发展脉络
Chat Paper
正在生成论文摘要