Training And Validation Of A Deep Learning Architecture For The Automatic Analysis Of Coronary Angiography
EUROINTERVENTION(2021)
摘要
Background: In recent years, the use of deep learning has become more commonplace in the biomedical field and its development will greatly assist clinical and imaging data interpretation. Most existing machine learning methods for coronary angiography analysis are limited to a single aspect.Aims: We aimed to achieve an automatic and multimodal analysis to recognise and quantify coronary angiography, integrating multiple aspects, including the identification of coronary artery segments and the recognition of lesion morphology.Methods: A data set of 20,612 angiograms was retrospectively collected, among which 13,373 angiograms were labelled with coronary artery segments, and 7,239 were labelled with special lesion morphology. Trained and optimised by these labelled data, one network recognised 20 different segments of coronary arteries, while the other detected lesion morphology, including measures of lesion diameter stenosis as well as calcification, thrombosis, total occlusion, and dissection detections in an input angiogram.Results: For segment prediction, the recognition accuracy was 98.4%, and the recognition sensitivity was 85.2%. For detecting lesion morphologies including stenotic lesion, total occlusion, calcification, thrombosis, and dissection, the F1 scores were 0.829, 0.810, 0.802, 0.823, and 0.854, respectively. Only two seconds were needed for the automatic recognition.Conclusions: Our deep learning architecture automatically provides a coronary diagnostic map by integrating multiple aspects. This helps cardiologists to flag and diagnose lesion severity and morphology during the intervention.
更多查看译文
关键词
artificial intelligence, coronary artery disease, imaging modalities, multiple vessel disease
AI 理解论文
溯源树
样例
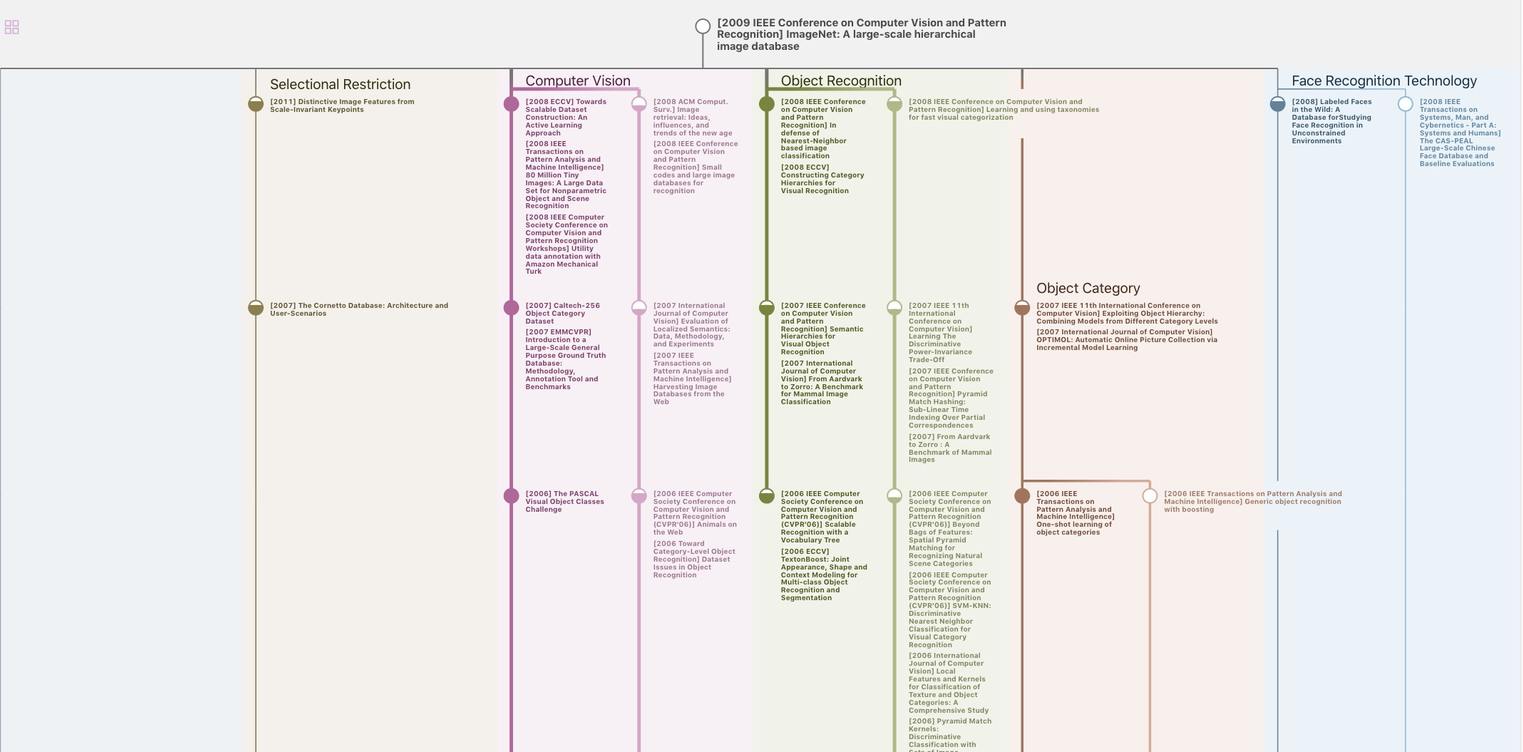
生成溯源树,研究论文发展脉络
Chat Paper
正在生成论文摘要