Infrastructure recovery curve estimation using Gaussian process regression on expert elicited data
Reliability Engineering & System Safety(2022)
摘要
The U.S. National Institute of Standards and Technology (NIST)’s Community Resilience Planning Guide uses recovery times of infrastructure functions as key metrics for disaster resilience. The existing literature also widely uses the recovery curve and the area under it to measure infrastructure resilience. Therefore, infrastructure recovery curve estimation is critical to understanding and improving disaster resilience. Unfortunately, this process is challenging in the pre-event planning context due to lack of historical data. To bridge this gap, we consider a situation where infrastructure experts are asked to estimate the time for different infrastructure systems to recover to certain functionality levels after a scenario hazard event. We propose a methodological framework to use expert-elicited data to estimate the expected recovery time curve of a particular infrastructure system. This framework uses the Gaussian process regression (GPR) to capture the experts’ estimation-uncertainty and satisfy known physical constraints of recovery processes. The framework is designed to find a balance between the data collection cost of expert elicitation and the prediction accuracy of GPR. We evaluate the framework on simulated expert-elicited data concerning two case study events, the 1995 Great Hanshin-Awaji Earthquake and the 2011 Great East Japan Earthquake. It is shown that the framework is robust against different configurations such as the number of experts, how the quantities of interest are elicited, and uncertainty in the experts’ estimates.
更多查看译文
关键词
Gaussian process regression,Expert elicitation,Infrastructure restoration,Disaster recovery,Resilience planning
AI 理解论文
溯源树
样例
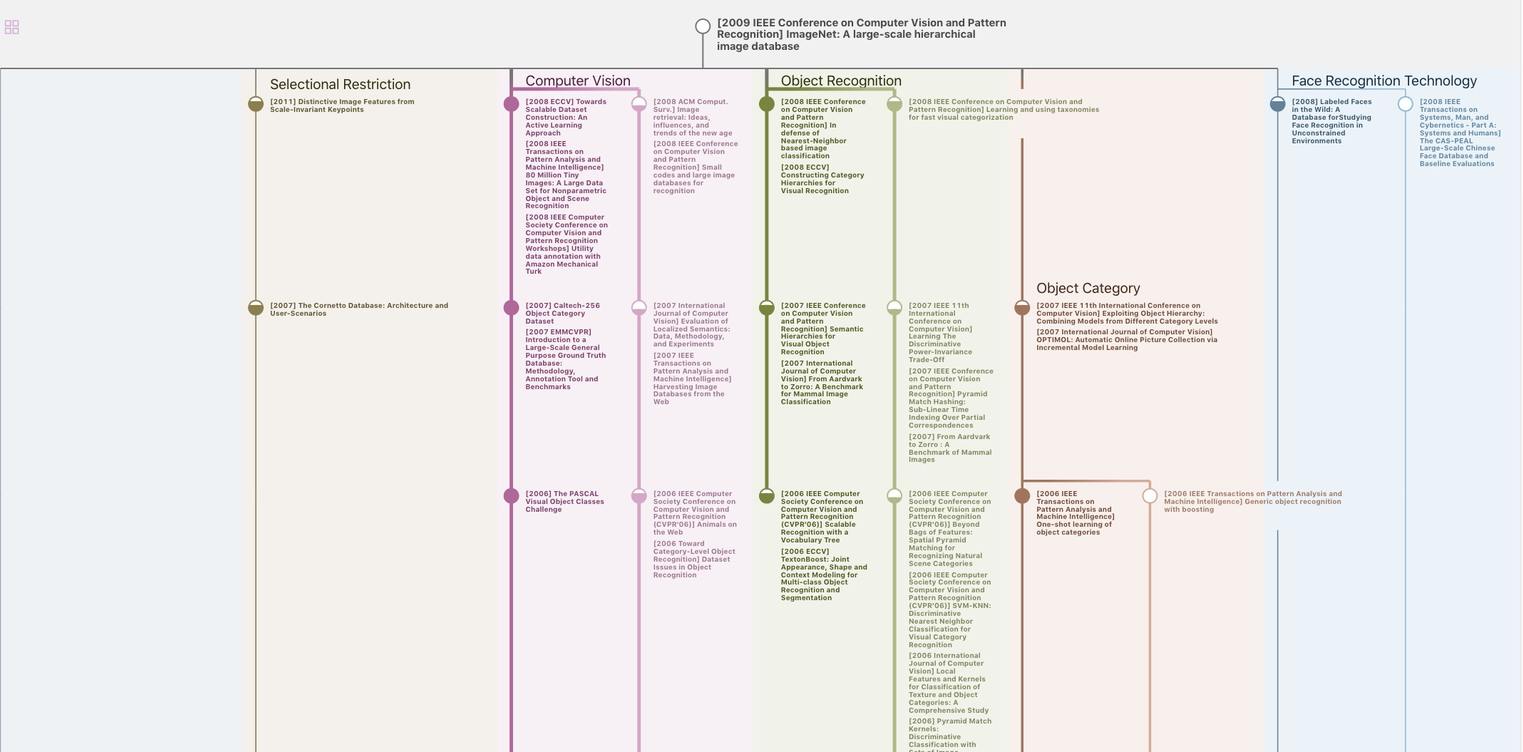
生成溯源树,研究论文发展脉络
Chat Paper
正在生成论文摘要