Locally Connected Network for Monocular 3D Human Pose Estimation
IEEE Transactions on Pattern Analysis and Machine Intelligence(2022)
摘要
We present an approach for 3D human pose estimation from monocular images. The approach consists of two steps: it first estimates a 2D pose from an image and then estimates the corresponding 3D pose. This paper focuses on the second step. Graph convolutional network (GCN) has recently become the de facto standard for human pose related tasks such as action recognition. However, in this work, we show that GCN has critical limitations when it is used for 3D pose estimation due to the inherent weight sharing scheme. The limitations are clearly exposed through a novel reformulation of GCN, in which both GCN and Fully Connected Network (FCN) are its special cases. In addition, on top of the formulation, we present
locally connected network
(LCN) to overcome the limitations of GCN by allocating dedicated rather than shared filters for different joints. We jointly train the LCN network with a 2D pose estimator such that it can handle inaccurate 2D poses. We evaluate our approach on two benchmark datasets and observe that LCN outperforms GCN, FCN, and the state-of-the-art methods by a large margin. More importantly, it demonstrates strong cross-dataset generalization ability because of sparse connections among body joints.
更多查看译文
关键词
3D human pose estimation,locally connected network,graph convolution
AI 理解论文
溯源树
样例
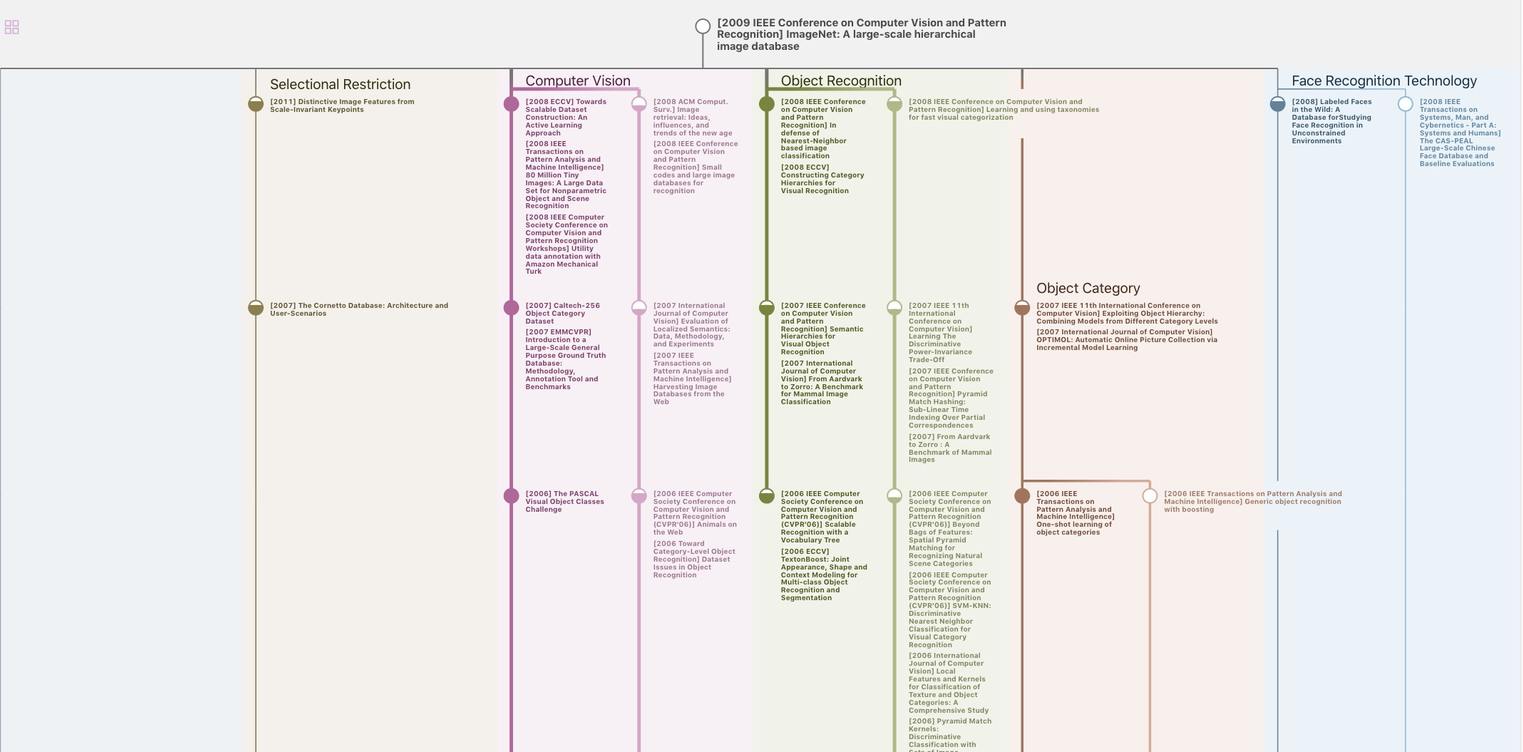
生成溯源树,研究论文发展脉络
Chat Paper
正在生成论文摘要