Efficient Algorithms For Covariate Analysis With Dynamic Data Using Nonlinear Mixed-Effects Model
STATISTICAL METHODS IN MEDICAL RESEARCH(2021)
摘要
Nonlinear mixed-effects modeling is one of the most popular tools for analyzing repeated measurement data, particularly for applications in the biomedical fields. Multiple integration and nonlinear optimization are the two major challenges for likelihood-based methods in nonlinear mixed-effects modeling. To solve these problems, approaches based on empirical Bayesian estimates have been proposed by breaking the problem into a nonlinear mixed-effects model with no covariates and a linear regression model without random effect. This approach is time-efficient as it involves no covariates in the nonlinear optimization. However, covariate effects based on empirical Bayesian estimates are underestimated and the bias depends on the extent of shrinkage. Marginal correction method has been proposed to correct the bias caused by shrinkage to some extent. However, the marginal approach appears to be suboptimal when testing covariate effects on multiple model parameters, a situation that is often encountered in real-world data analysis. In addition, the marginal approach cannot correct the inaccuracy in the associatedp-values. In this paper, we proposed a simultaneous correction method (nSCEBE), which can handle the situation where covariate analysis is performed on multiple model parameters. Simulation studies and real data analysis showed that nSCEBE is accurate and efficient for both effect-size estimation andp-value calculation compared with the existing methods. Importantly, nSCEBE can be >2000 times faster than the standard mixed-effects models, potentially allowing utilization for high-dimension covariate analysis for longitudinal or repeated measured outcomes.
更多查看译文
关键词
Nonlinear mixed-effects model, empirical Bayesian estimates, shrinkage, marginal correction, GALLOP, simultaneous correction
AI 理解论文
溯源树
样例
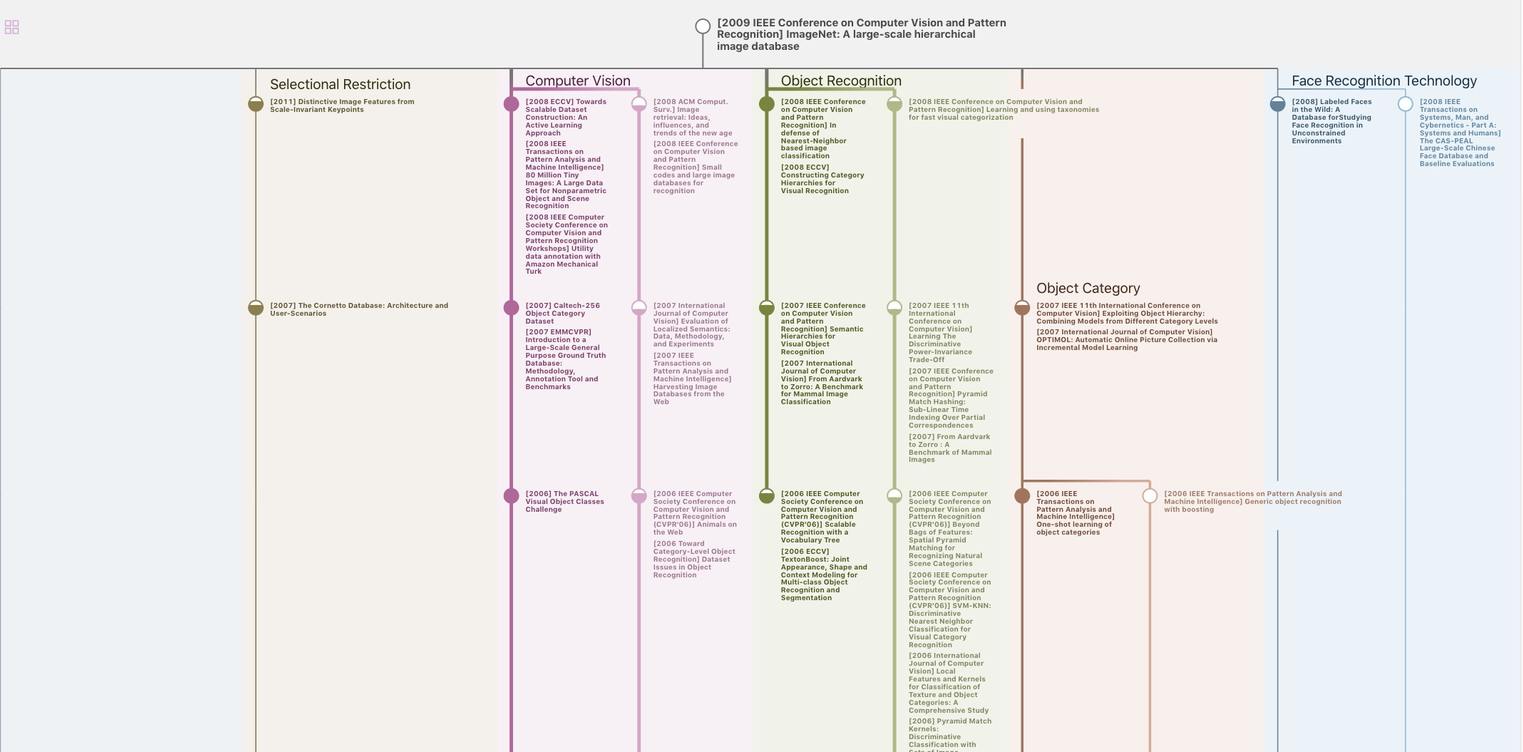
生成溯源树,研究论文发展脉络
Chat Paper
正在生成论文摘要