Efficient Hierarchical Clustering for Classification and Anomaly Detection
arxiv(2020)
摘要
We address the problem of large scale real-time classification of content posted on social networks, along with the need to rapidly identify novel spam types. Obtaining manual labels for user-generated content using editorial labeling and taxonomy development lags compared to the rate at which new content type needs to be classified. We propose a class of hierarchical clustering algorithms that can be used both for efficient and scalable real-time multiclass classification as well as in detecting new anomalies in user-generated content. Our methods have low query time, linear space usage, and come with theoretical guarantees with respect to a specific hierarchical clustering cost function (Dasgupta, 2016). We compare our solutions against a range of classification techniques and demonstrate excellent empirical performance.
更多查看译文
关键词
efficient hierarchical clustering,anomaly detection,classification
AI 理解论文
溯源树
样例
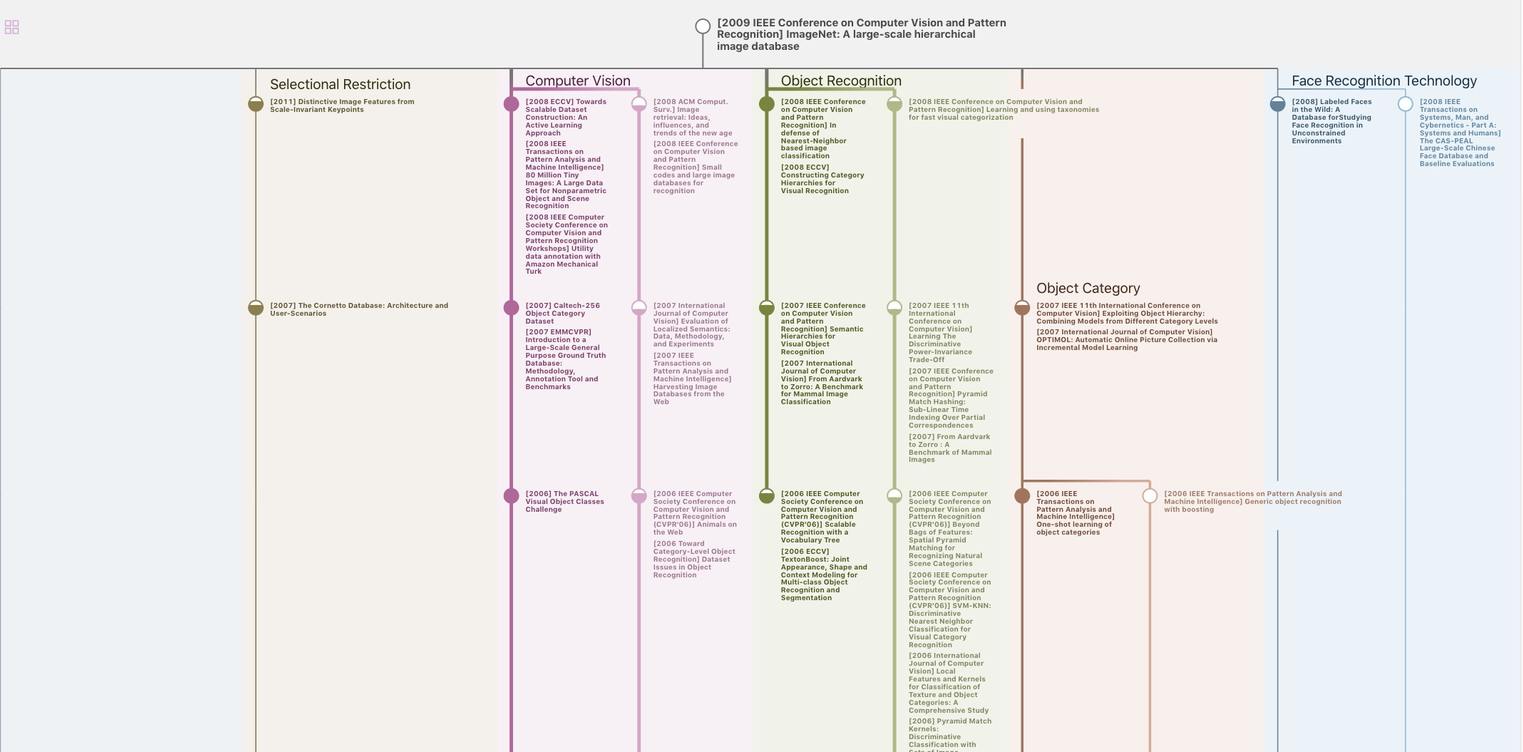
生成溯源树,研究论文发展脉络
Chat Paper
正在生成论文摘要