Local Generalization and Bucketization Technique for Personalized Privacy Preservation
Journal of King Saud University Computer and information sciences/Maǧalaẗ ǧamʼaẗ al-malīk Saud ùlm al-ḥasib wa al-maʼlumat(2023)
摘要
Anonymization technique has been extensively studied and widely applied for privacy-preserving data publishing. In most previous approaches, a microdata table consists of three categories of attributes, namely explicit-identifier, quasi-identifier (QI), and sensitive attribute. In general, individuals may have different views on the sensitivity of different attributes. Therefore, there is another type of attribute that contains both QI values and sensitive values, termed semi-sensitive attribute. In this paper, we propose a new anonymization technique, called Local Generalization and Bucketization, to prevent identity disclosure and protect the sensitive values on each semi-sensitive attribute and sensitive attribute. The rationale is to use local generalization and local bucketization to divide the tuples into local equivalence groups and partition the sensitive values into local buckets, respectively. The protections of local generalization and local bucketization are independent, so that they can be implemented by appropriate algorithms without weakening other protection. Besides, the protection of local bucketization for each semi-sensitive attribute and sensitive attribute is also independent. Consequently, local bucketization can comply with various principles in different attributes according to the actual requirements of anonymization. We conducted extensive experiments to illustrate the effectiveness of the proposed approach.
更多查看译文
关键词
Privacy preservation,Data publication,Local generalization,Local bucketization
AI 理解论文
溯源树
样例
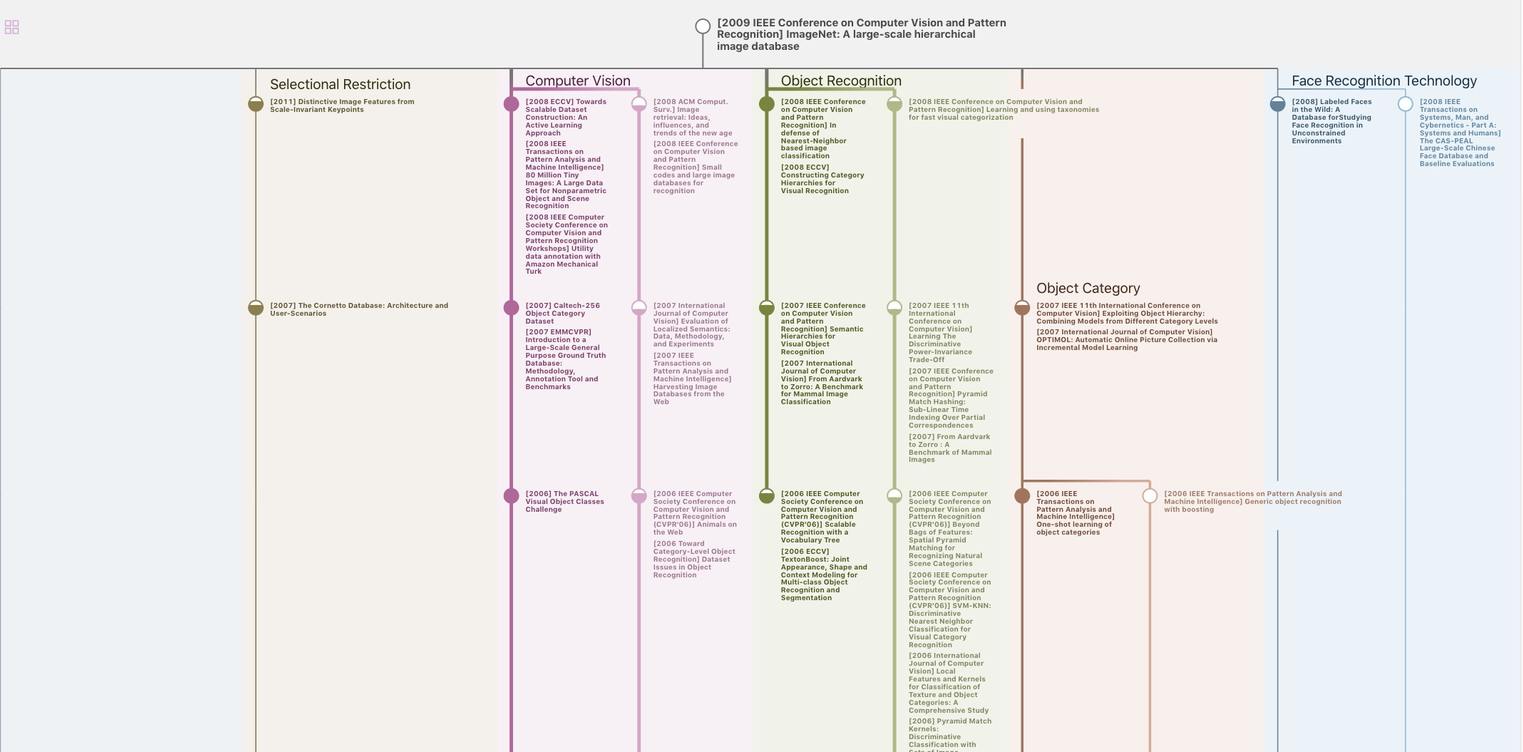
生成溯源树,研究论文发展脉络
Chat Paper
正在生成论文摘要