Estimating the Changing Infection Rate of COVID 19 Using Bayesian Models of Mobility
medRxiv(2020)
摘要
In order to prepare for and control the continued spread of the COVID-19 pandemic while minimizing its economic impact, the world needs to be able to estimate and predict COVID-199s spread. Unfortunately, we cannot directly observe the prevalence or growth rate of COVID-19; these must be inferred using some kind of model. We propose a hierarchical Bayesian extension to the classic susceptible-exposed-infected-removed (SEIR) compartmental model that adds compartments to account for isolation and death and allows the infection rate to vary as a function of both mobility data collected from mobile phones and a latent time-varying factor that accounts for changes in behavior not captured by mobility data. Since confirmed-case data is unreliable, we infer the model9s parameters conditioned on deaths data. We replace the exponential-waiting-time assumption of classic compartmental models with Erlang distributions, which allows for a more realistic model of the long lag between exposure and death. The mobility data gives us a leading indicator that can quickly detect changes in the pandemic9s local growth rate and forecast changes in death rates weeks ahead of time. This is an analysis of observational data, so any causal interpretations of the model9s inferences should be treated as suggestive at best; nonetheless, the model9s inferred relationship between different kinds of trips and the infection rate do suggest some possible hypotheses about what kinds of activities might contribute most to COVID-199s spread.
更多查看译文
关键词
infection rate,bayesian models,mobility
AI 理解论文
溯源树
样例
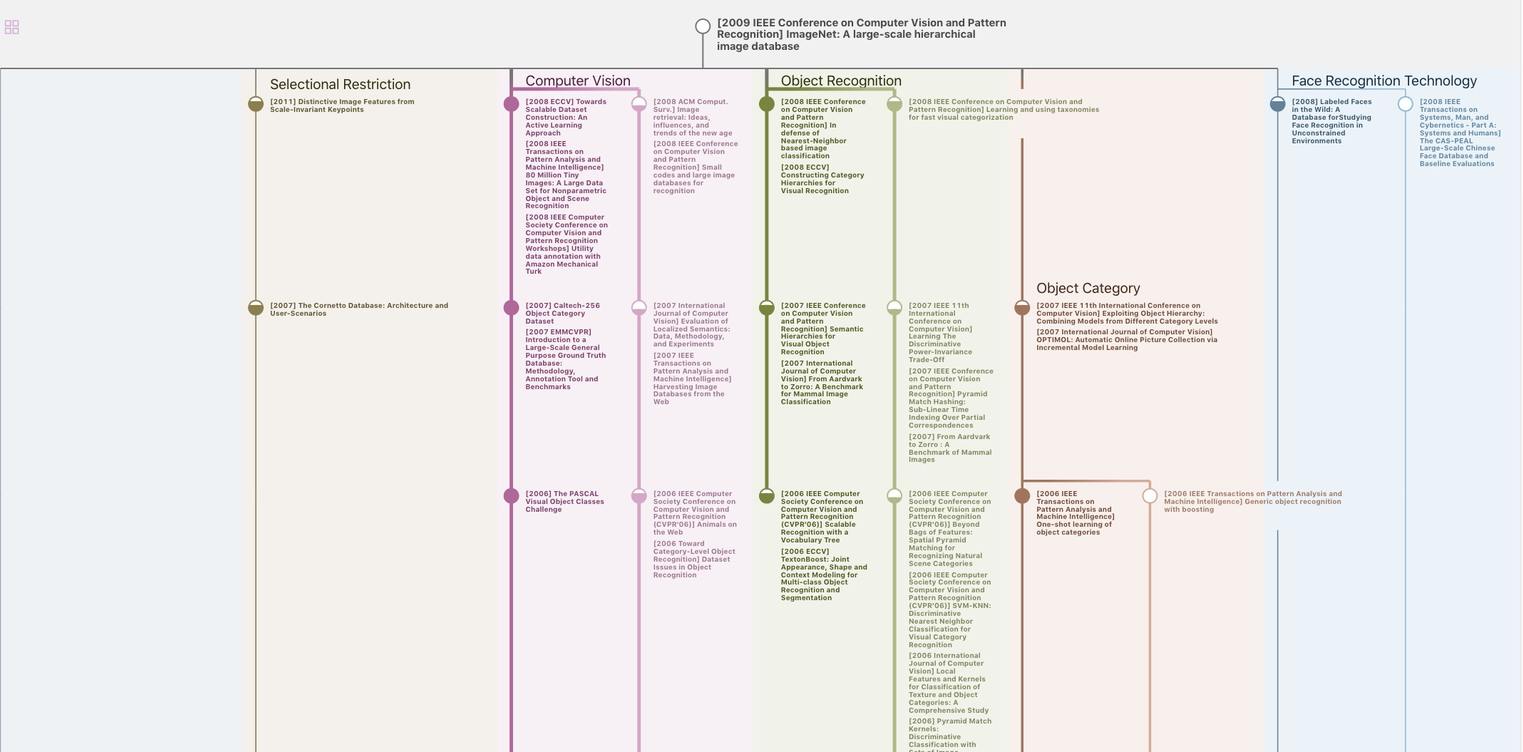
生成溯源树,研究论文发展脉络
Chat Paper
正在生成论文摘要