Disentangled Adversarial Autoencoder For Subject-Invariant Physiological Feature Extraction
IEEE SIGNAL PROCESSING LETTERS(2020)
摘要
Recent developments in biosignal processing have enabled users to exploit their physiological status for manipulating devices in a reliable and safe manner. One major challenge of physiological sensing lies in the variability of biosignals across different users and tasks. To address this issue, we propose an adversarial feature extractor for transfer learning to exploit disentangled universal representations. We consider the trade-off between task-relevant features and user-discriminative information by introducing additional adversary and nuisance networks in order to manipulate the latent representations such that the learned feature extractor is applicable to unknown users and various tasks. Results on cross-subject transfer evaluations exhibit the benefits of the proposed framework, with up to 8.8% improvement in average accuracy of classification, and demonstrate adaptability to a broader range of subjects.
更多查看译文
关键词
Adversarial deep learning, stress assessment
AI 理解论文
溯源树
样例
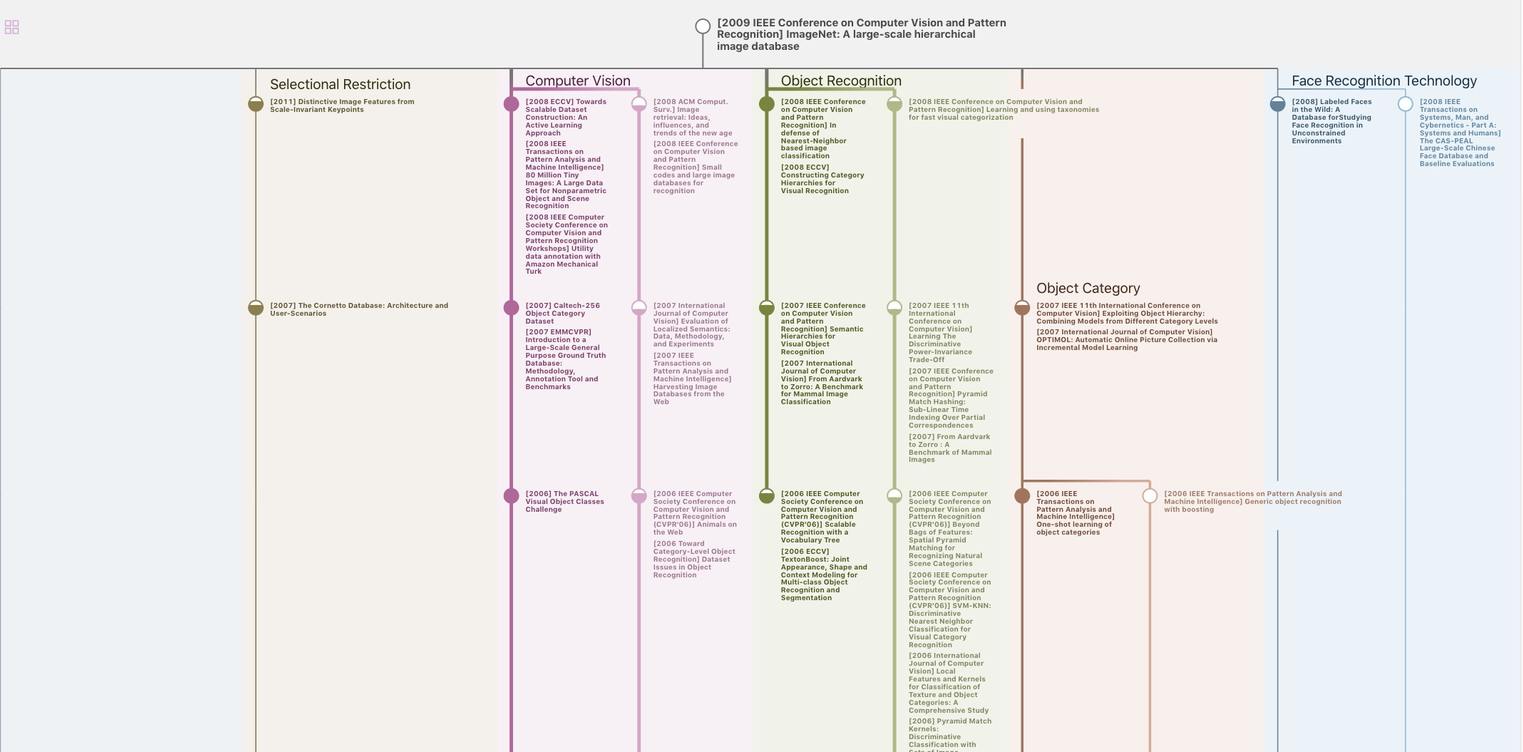
生成溯源树,研究论文发展脉络
Chat Paper
正在生成论文摘要