Multi kernel based deep residual networks for image super resolution
International Workshop on Advanced Image Technology (IWAIT) 2019(2019)
摘要
Single image super-resolution (SISR) is a classical problem in low-level vision task, which aims to find a mapping function from a single low-resolution (LR) input to corresponding high-resolution (HR) output. Recently, deep networks have achieved great success in SISR task. Recent successful deep models mostly consist of stacked the same size convolution filters whose size is mostly 3 by 3. To cope with more variations in both local dependencies and global contexts between LR and HR images, we propose multi-kernel based deep residual networks for SISR. Since larger kernel requires more parameters, we adopt dilated convolution for increasing the size of the receptive field. Also, we adopt local feature fusion, global feature fusion and local residual learning for controlling the multi-scale features and hence for the better performance by accelerating the convergence. Experimental results show that our …
更多查看译文
关键词
Single image super-resolution (SISR), convolutional neural networks (CNN), dilated convolution, residual learning
AI 理解论文
溯源树
样例
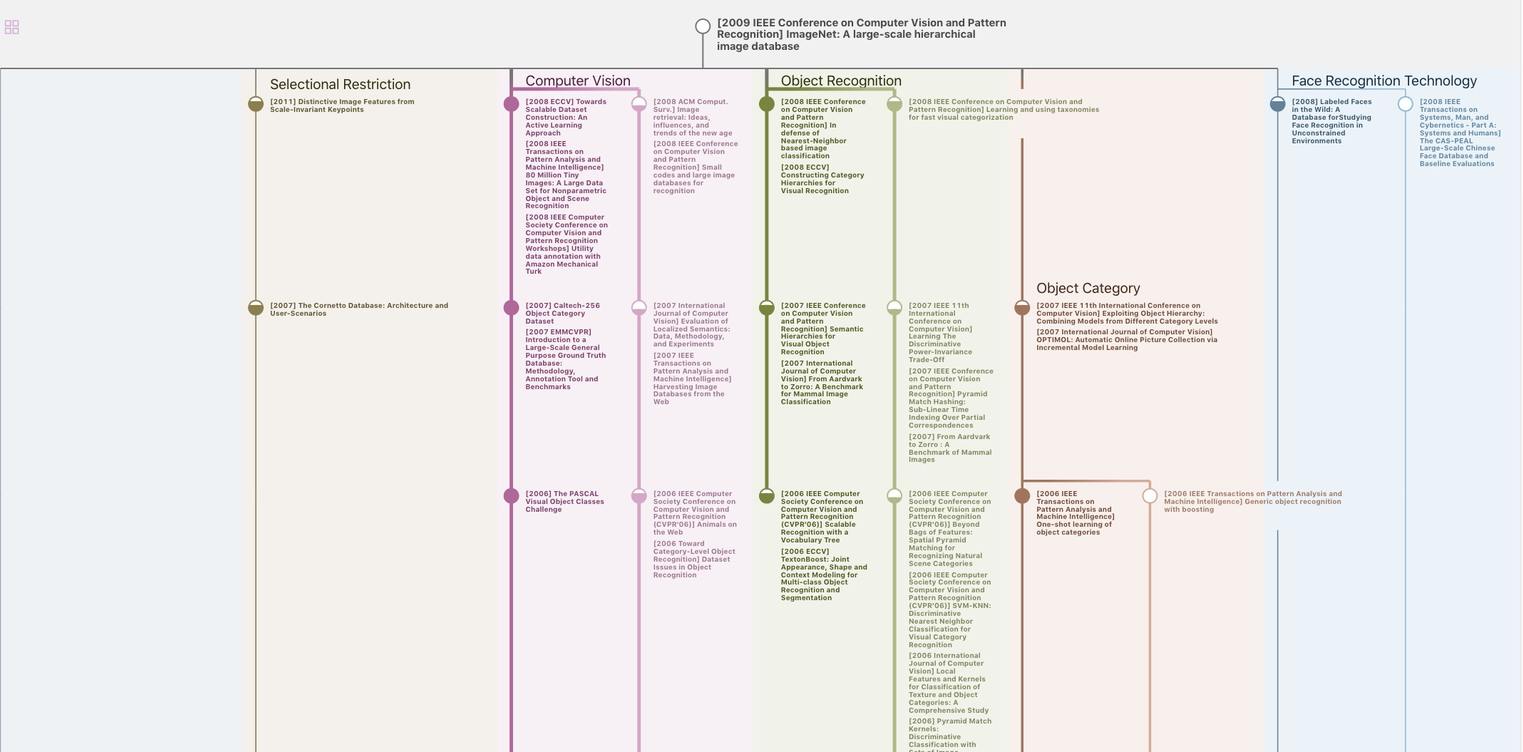
生成溯源树,研究论文发展脉络
Chat Paper
正在生成论文摘要