Safe Model-Based Meta-Reinforcement Learning: A Sequential Exploration-Exploitation Framework
arxiv(2020)
摘要
Safe deployment of autonomous robots in diverse environments requires agents that are capable of safe and efficient adaptation to new scenarios. Indeed, achieving both data efficiency and well-calibrated safety has been a central problem in robotic learning and adaptive control due in part to the tension between these objectives. In this work, we develop a framework for probabilistically safe operation with uncertain dynamics. This framework relies on Bayesian meta-learning for efficient inference of system dynamics with calibrated uncertainty. We leverage the model structure to construct confidence bounds which hold throughout the learning process, and factor this uncertainty into a model-based planning framework. By decomposing the problem of control under uncertainty into discrete exploration and exploitation phases, our framework extends to problems with high initial uncertainty while maintaining probabilistic safety and persistent feasibility guarantees during every phase of operation. We validate our approach on the problem of a nonlinear free flying space robot manipulating a payload in cluttered environments, and show it can safely learn and reach a goal.
更多查看译文
关键词
Chance-constrained planning,dynamics,meta-learning,reachability analysis,robotics,system identification
AI 理解论文
溯源树
样例
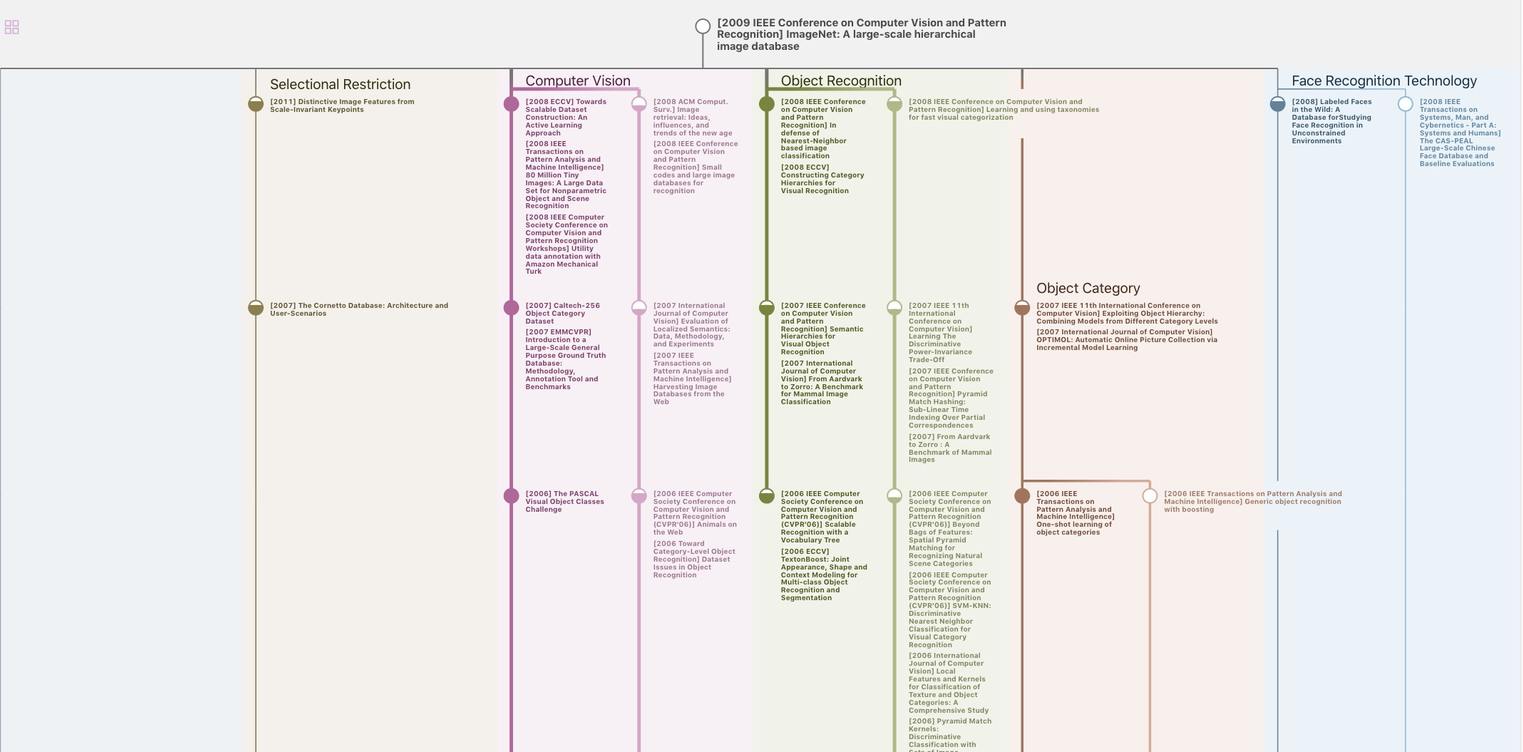
生成溯源树,研究论文发展脉络
Chat Paper
正在生成论文摘要