Adversarial Domain Adaptation for Analyzing Low Resolution Images
user-5f03edee4c775ed682ef5237(2020)
摘要
Domain adaptation has recently been explored for transferring knowledge from a source domain, where there exists ground truth, to a target domain without ground truth. It can also be applied to paired datasets where the task is to learn from a source domain that contains more informative features. This stands to benefit domains, ie remote sensing, where it is desirable to analyze images in low resolution because they are acquired more frequently. In this direction, we adopt an adversarial domain adaptation method to transfer knowledge from a source domain with high resolution images to a target domain with low resolution images. Additionally, we propose a sequential domain adaptation method to stabilize training when analyzing very low resolution images. Finally, we empirically find that models trained across different resolution images can complement each other, and we ensemble adapted and original target networks to utilize adapted high and low resolution features. Our final approach yields state-of-the-art results for low resolution image classification on a set of diverse benchmarks, including fMoW, Stanford Cars, and Caltech-USCD Birds.
更多查看译文
AI 理解论文
溯源树
样例
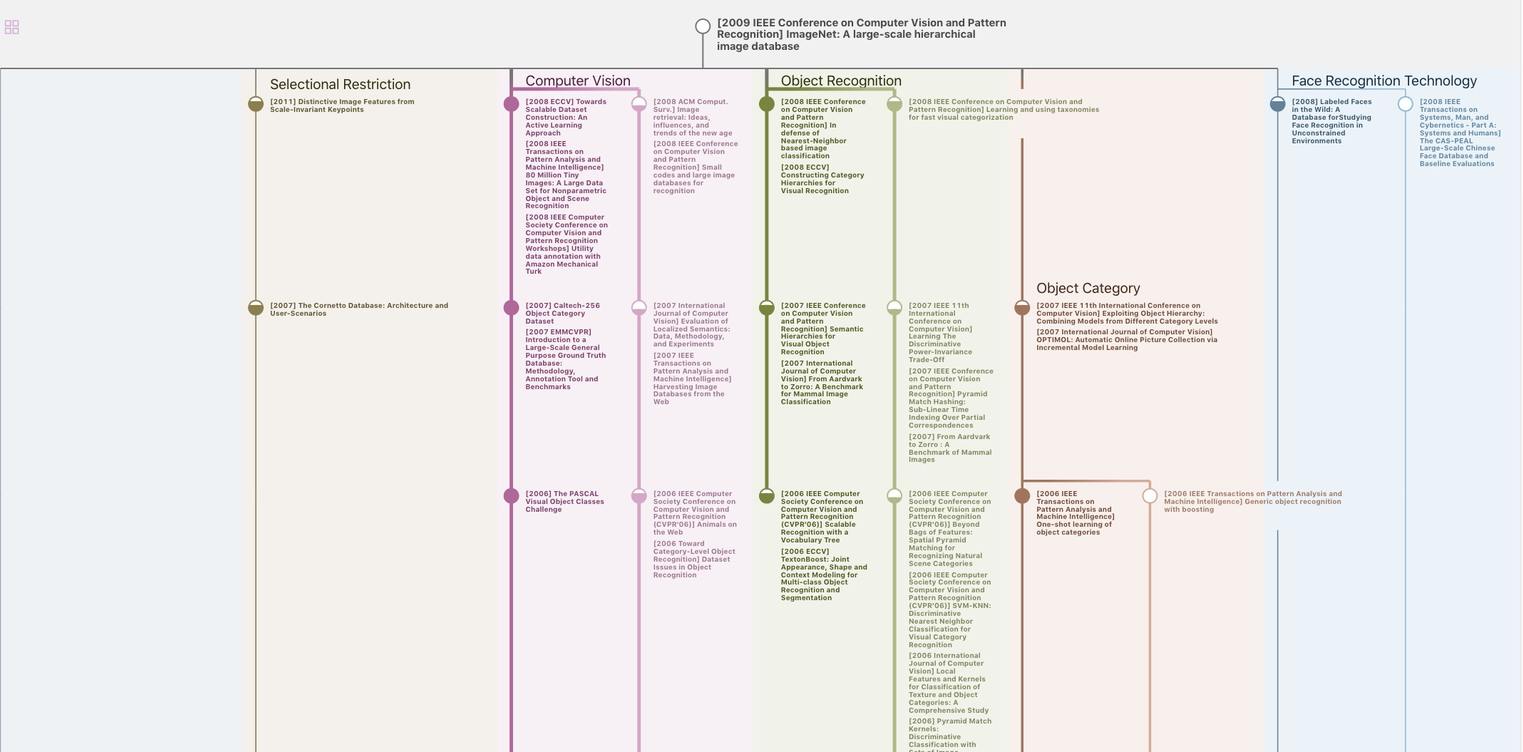
生成溯源树,研究论文发展脉络
Chat Paper
正在生成论文摘要