Lymph Node Gross Tumor Volume Detection and Segmentation via Distance-Based Gating Using 3D CT/PET Imaging in Radiotherapy
medical image computing and computer assisted intervention(2020)
摘要
Finding, identifying and segmenting suspicious cancer metastasized lymph nodes from 3D multi-modality imaging is a clinical task of paramount importance. In radiotherapy, they are referred to as Lymph Node Gross Tumor Volume (GTV\(_{LN}\)). Determining and delineating the spread of GTV\(_{LN}\) is essential in defining the corresponding resection and irradiating regions for the downstream workflows of surgical resection and radiotherapy of various cancers. In this work, we propose an effective distance-based gating approach to simulate and simplify the high-level reasoning protocols conducted by radiation oncologists, in a divide-and-conquer manner. GTV\(_{LN}\) is divided into two subgroups of “tumor-proximal" and “tumor-distal", respectively, by means of binary or soft distance gating. This is motivated by the observation that each category can have distinct though overlapping distributions of appearance, size and other LN characteristics. A novel multi-branch detection-by-segmentation network is trained with each branch specializing on learning one GTV\(_{LN}\) category features, and outputs from multi-branch are fused in inference. The proposed method is evaluated on an in-house dataset of 141 esophageal cancer patients with both PET and CT imaging modalities. Our results validate significant improvements on the mean recall from \(72.5\%\) to \(78.2\%\), as compared to previous state-of-the-art work. The highest achieved GTV\(_{LN}\) recall of \(82.5\%\) at \(20\%\) precision is clinically relevant and valuable since human observers tend to have low sensitivity (\(\sim \)80% for the most experienced radiation oncologists, as reported by literature
[5]).
更多查看译文
关键词
Lymph Node Gross Tumor Volume (GTV$$_{LN}$$LN),CT/PET Imaging,3D Distance Transformation,Distance-based Gating
AI 理解论文
溯源树
样例
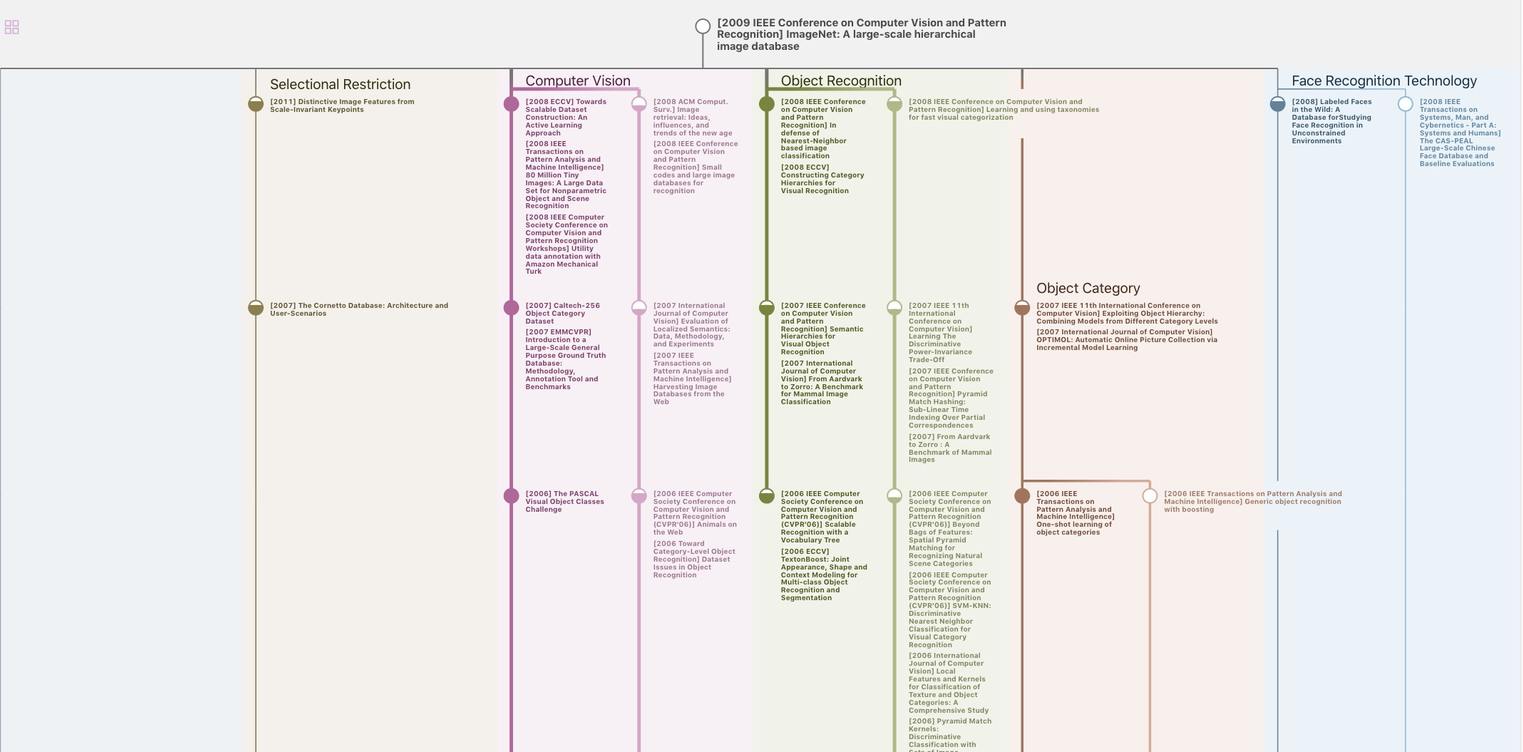
生成溯源树,研究论文发展脉络
Chat Paper
正在生成论文摘要