Risk of relapse after anti-PD1 discontinuation in patients with Hodgkin lymphoma
EUROPEAN JOURNAL OF NUCLEAR MEDICINE AND MOLECULAR IMAGING(2020)
摘要
Introduction Patients with relapsed/refractory Hodgkin lymphoma (R/R HL) experience high response rates upon anti-PD1 therapy. In these patients, the optimal duration of treatment and the risk of relapse after anti-PD1 discontinuation are unknown. Methods We retrospectively analyzed patients with R/R HL who responded to anti-PD1 monotherapy and discontinued the treatment either because of unacceptable toxicity or prolonged remission. A machine learning algorithm based on 17 candidate variables was trained and validated to predict progression-free survival (PFS) landmarked at the time of discontinuation of anti-PD1 therapy. Results Forty patients from 14 centers were randomly assigned to training (n = 25) and validation (n = 15) sets. At the time of anti-PD1 discontinuation, patients had received treatment for a median duration of 11.2 (range, 0—time to best response was not statistically significant in discriminating patients with PFS lesser or greater than 12 months). Considering PFS status as a binary variable (alive or dead) at a specific time point (12 months) is convenient, intuitive and allows for comparing the value of potential predicting variables in these two groups of patients. Nonetheless, this approach has two drawbacks: first, it binarizes outcome; second, it excludes patients alive with a time to last follow up lesser 12 months. Therefore, it is less powerful to demonstrate statistically significant association with PFS even if it exists 5 months. Patients discontinued anti-PD1 treatment either because of prolonged remission (N = 27, 67.5%) or unacceptable toxicity (N = 13, 32.5%). Most patients were in CR (N = 35, 87.5%) at the time of anti-PD1 discontinuation. In the training set, the machine learning algorithm identified that the most important variables to predict PFS were patients’ age, time to best response, and presence or absence of CR. The performance observed in the training set was validated in the validation set. Conclusion In this pilot, proof of concept study using a machine learning algorithm, we identified biomarkers capable of predicting the risk of relapse after anti-PD1 discontinuation (age, time to best response, quality of response). Once confirmed, these simple biomarkers will represent useful tools to guide the management of these patients.
更多查看译文
关键词
Immunotherapy, Biomarkers, Hodgkin lymphoma, Checkpoint inhibitors
AI 理解论文
溯源树
样例
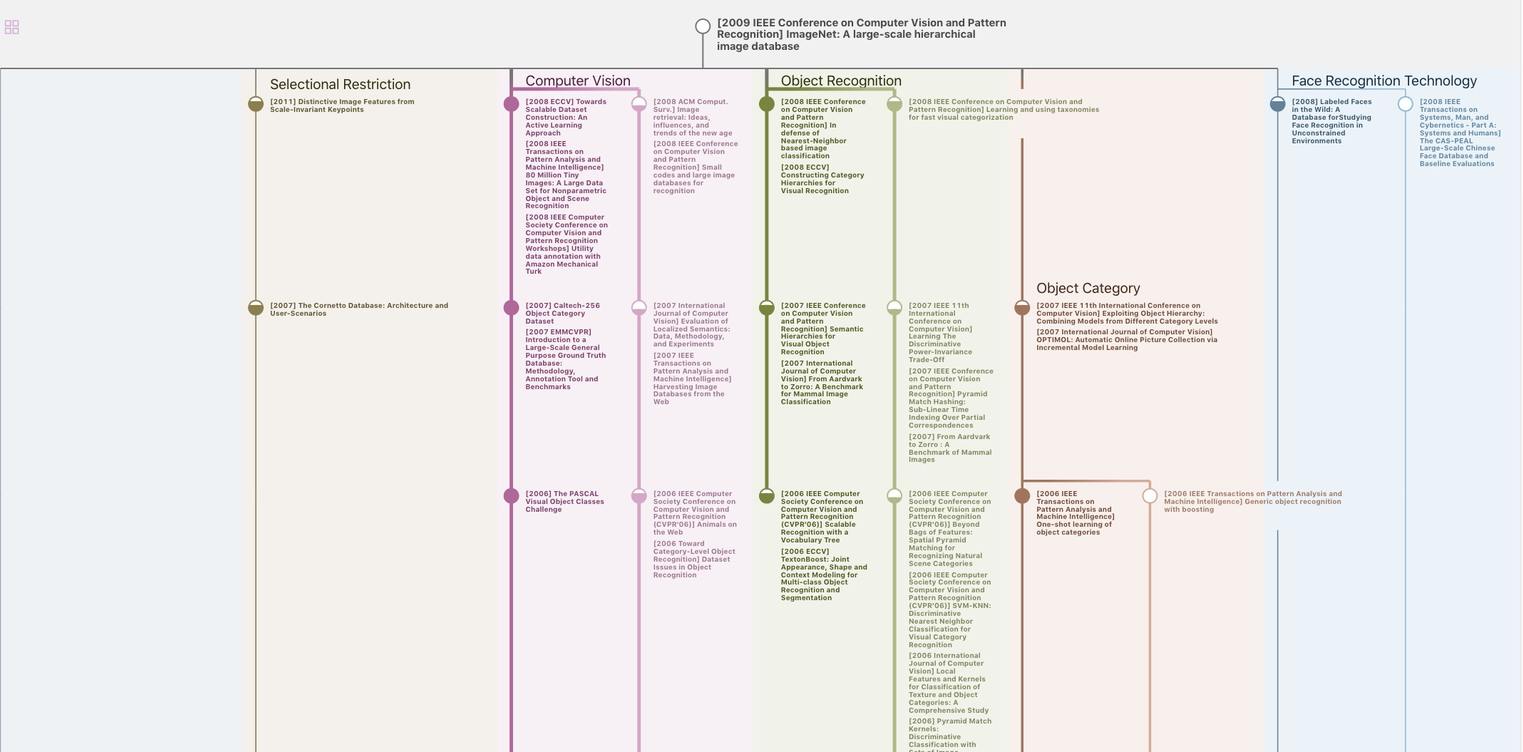
生成溯源树,研究论文发展脉络
Chat Paper
正在生成论文摘要