Unsupervised satellite image classification based on partial transfer learning
Aerospace Systems(2019)
摘要
Satellite image classification plays an important role in many fields. It can be divided into two groups: supervised and unsupervised classification. The former requires a large number of labeled data, while, in practice, satellite images usually lack of sufficient ones. How to achieve high accuracy on unsupervised satellite image classification is a key problem. For tackling this problem, based on the idea of partial transfer learning, we propose an novel end-to-end unsupervised classification method called coordinate partial adversarial domain adaptation (CPADA) for satellite image classification. Under the aid of a novel coordinate loss, our framework transfers relevant examples in the shared classes to promote performance, and ignore irrelevant ones in the specific classes to mitigate negative transfer. Experiments show that our CPADA exceeds state-of-the-art results for unsupervised satellite image classification task.
更多查看译文
关键词
Unsupervised satellite image classification,Partial domain adaptation,Transfer learning
AI 理解论文
溯源树
样例
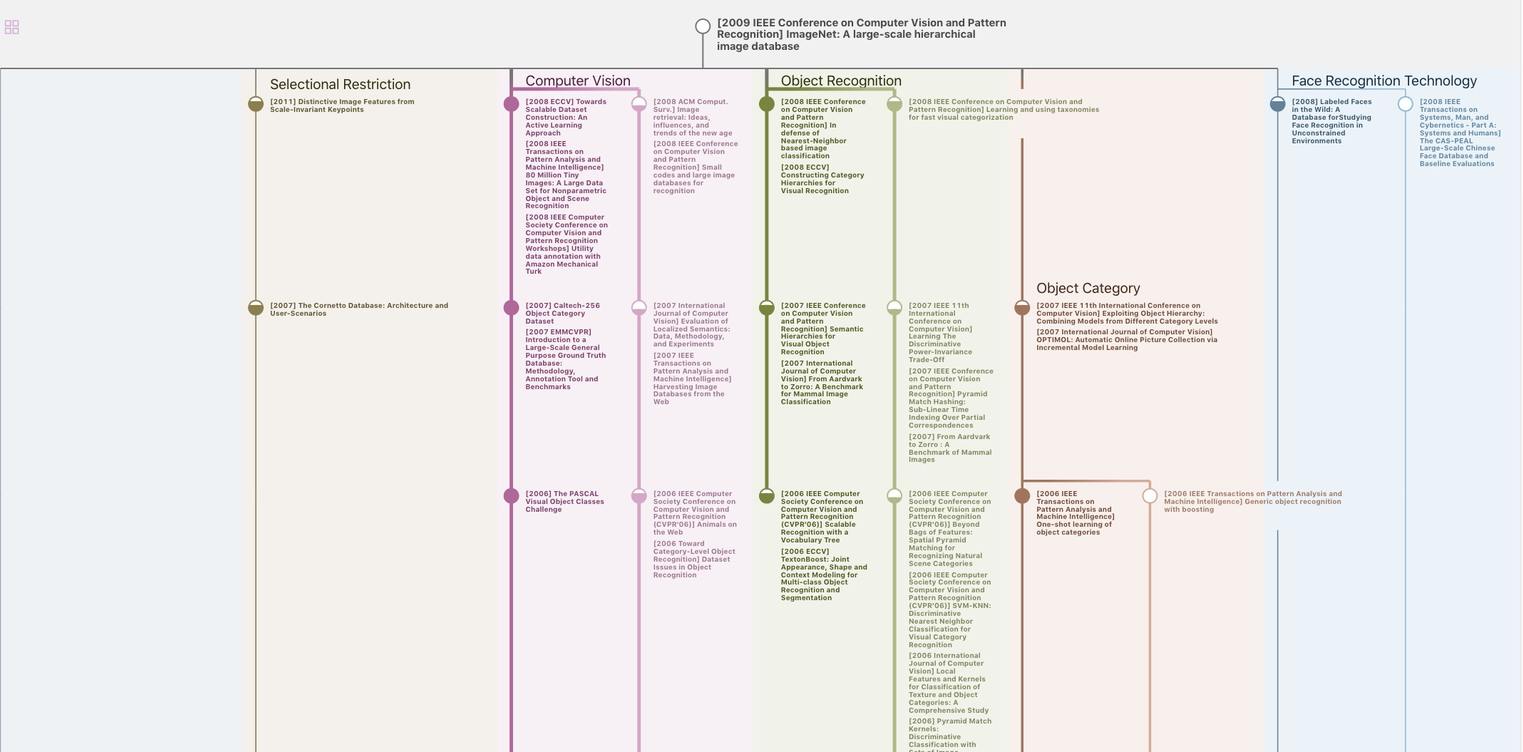
生成溯源树,研究论文发展脉络
Chat Paper
正在生成论文摘要