Traffic Dynamics Exploration and Incident Detection Using Spatiotemporal Graphical Modeling
Journal of Big Data Analytics in Transportation(2019)
摘要
To discover the spatial and temporal traffic patterns, this paper proposes a spatiotemporal graphical modeling approach, spatiotemporal pattern network (STPN), to explore traffic dynamics in large traffic networks. A measurement based on Granger causality is used to identify the characteristics of spatial and temporal traffic patterns. An anomaly score is estimated to detect and locate traffic incidents in diverse types and severities, and also to quantify the influence of incidents on traffic flow fluctuations. Built upon symbolic dynamics filtering, the proposed approach implements spatial and temporal feature extraction via discovering causal dependencies among road segments using STPN, system-wide pattern learning through an energy-based model, restricted Boltzmann machine, and inference using a newly developed root-cause analysis algorithm. Case studies are carried out using the probe vehicle data collected on Interstate Highway 80 in Iowa and the results show that the proposed approach is capable of (1) discovering and representing causal interactions among sub-systems (e.g., road segment) of a traffic network that provide valuable information for developing and applying customized traffic management strategies, (2) adaptively handling multiple nominal patterns mixed with anomalous data for effectively differentiating abnormal traffic system status and locating traffic incidents, and (3) quantifying the fluctuation of traffic flow and the severity of the detected incident via anomaly scores estimated from traffic speed behaviors. The findings from the case studies reiterate the importance of incorporating both temporal and spatial features for pattern analysis and incident detection. The proposed approach is built for real-time application and can be utilized for on-line incident detection.
更多查看译文
关键词
Traffic pattern analysis,Anomaly detection,Incident detection,Spatiotemporal graphical modeling,Root-cause analysis
AI 理解论文
溯源树
样例
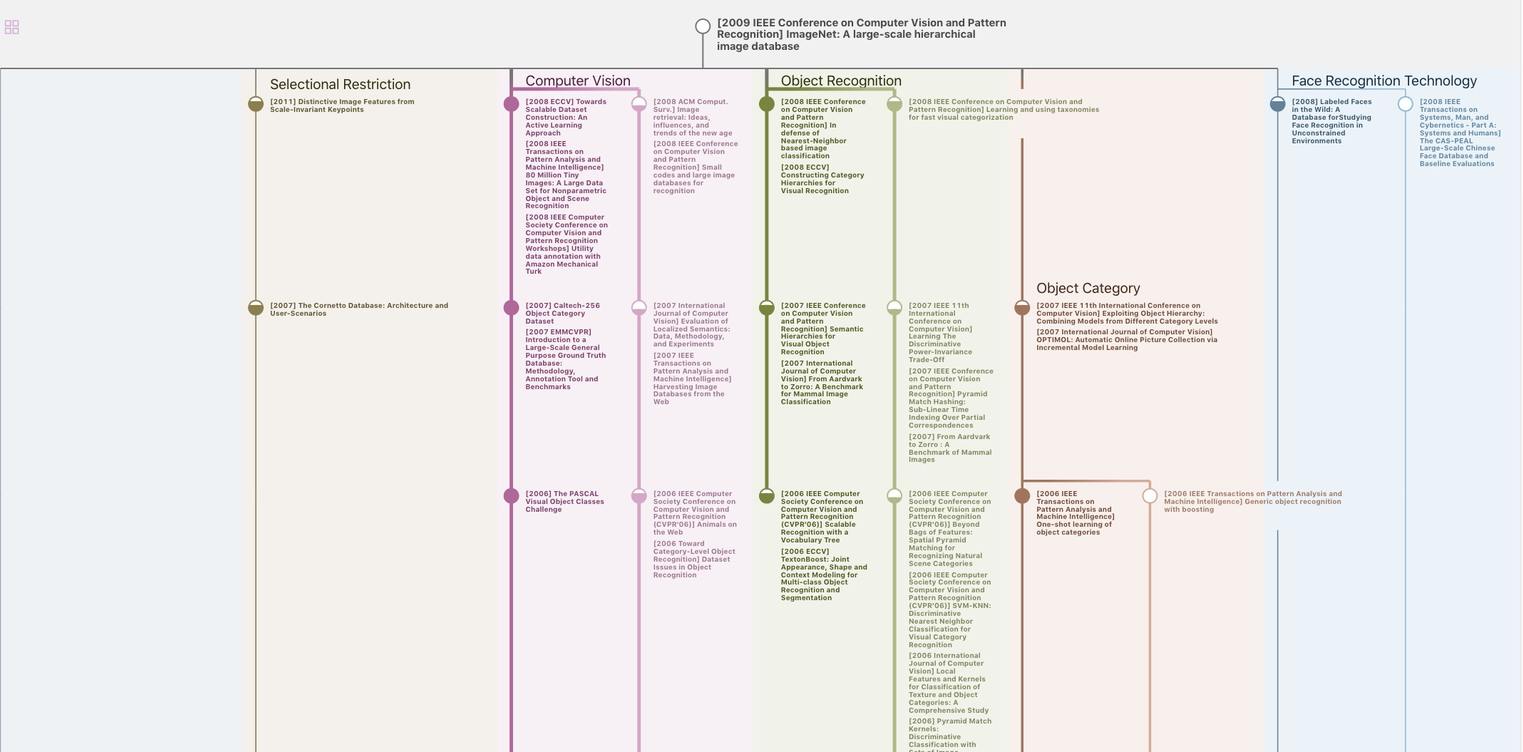
生成溯源树,研究论文发展脉络
Chat Paper
正在生成论文摘要