Langevin Cooling for Domain Translation
arxiv(2020)
摘要
Domain translation is the task of finding correspondence between two domains. Several Deep Neural Network (DNN) models, e.g., CycleGAN and cross-lingual language models, have shown remarkable successes on this task under the unsupervised setting---the mappings between the domains are learned from two independent sets of training data in both domains (without paired samples). However, those methods typically do not perform well on a significant proportion of test samples. In this paper, we hypothesize that many of such unsuccessful samples lie at the fringe---relatively low-density areas---of data distribution, where the DNN was not trained very well, and propose to perform Langevin dynamics to bring such fringe samples towards high density areas. We demonstrate qualitatively and quantitatively that our strategy, called Langevin Cooling (L-Cool), enhances state-of-the-art methods in image translation and language translation tasks.
更多查看译文
关键词
langevin cooling,translation
AI 理解论文
溯源树
样例
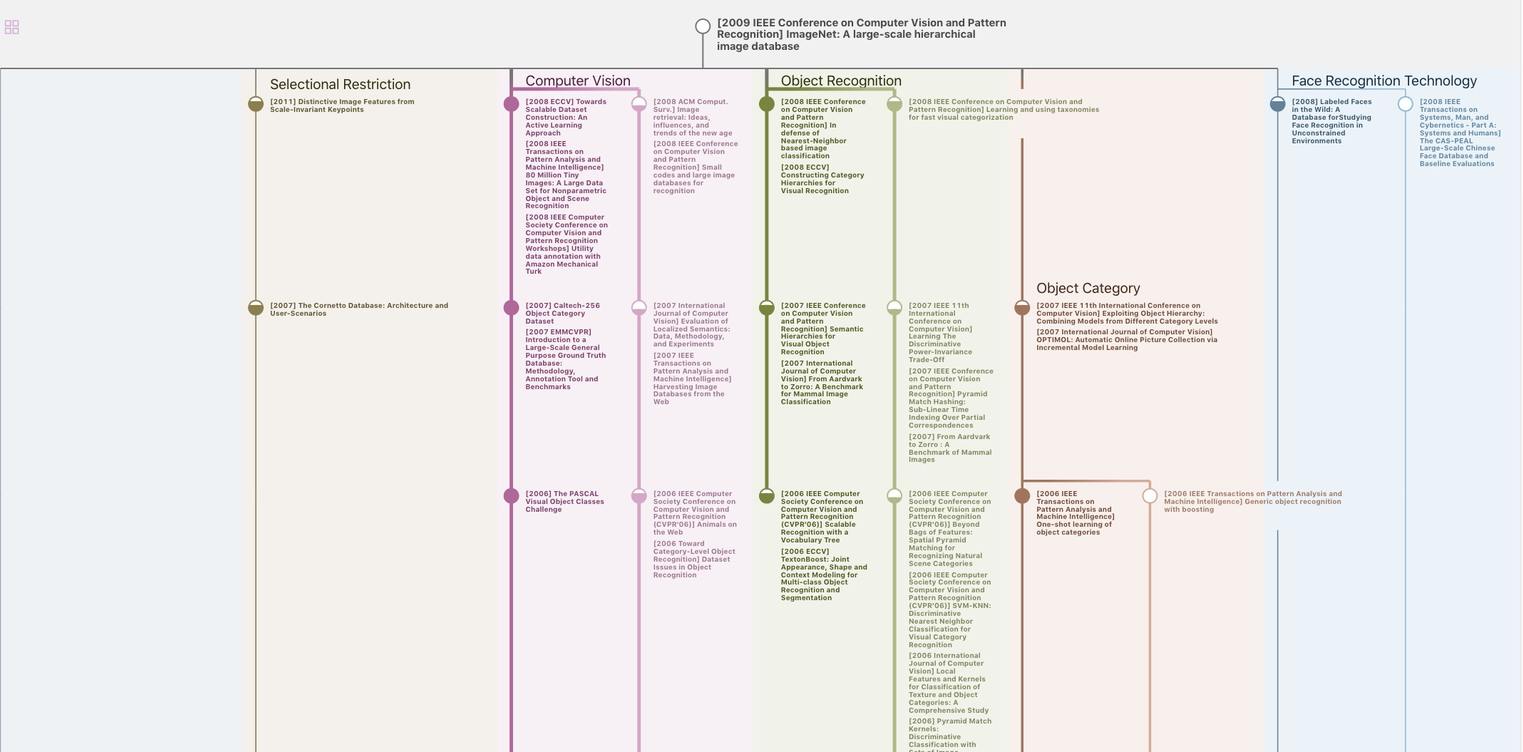
生成溯源树,研究论文发展脉络
Chat Paper
正在生成论文摘要