Eleven Routine Clinical Features Predict COVID 19 Severity
medRxiv(2020)
摘要
Severity prediction of COVID-19 remains one of the major clinical challenges for the ongoing pandemic. Here, we have recruited a 144 COVID-19 patient cohort, resulting in a data matrix containing 3,065 readings for 124 types of measurements over 52 days. A machine learning model was established to predict the disease progression based on the cohort consisting of training, validation, and internal test sets. A panel of eleven routine clinical factors constructed a classifier for COVID-19 severity prediction, achieving accuracy of over 98% in the discovery set. Validation of the model in an independent cohort containing 25 patients achieved accuracy of 80%. The overall sensitivity, specificity, positive predictive value (PPV), and negative predictive value (NPV) were 0.70, 0.99, 0.93, and 0.93, respectively. Our model captured predictive dynamics of lactate dehydrogenase (LDH) and creatine kinase (CK) while their levels were in the normal range. This model is accessible at https://www.guomics.com/covidAI/ for research purpose. (C) 2021 The Author(s). Published by Elsevier B.V. on behalf of Research Network of Computational and Structural Biotechnology.
更多查看译文
关键词
COVID-19, SARS-CoV-2, Severity prediction, Machine learning, Routine clinical test, Longitudinal dynamics
AI 理解论文
溯源树
样例
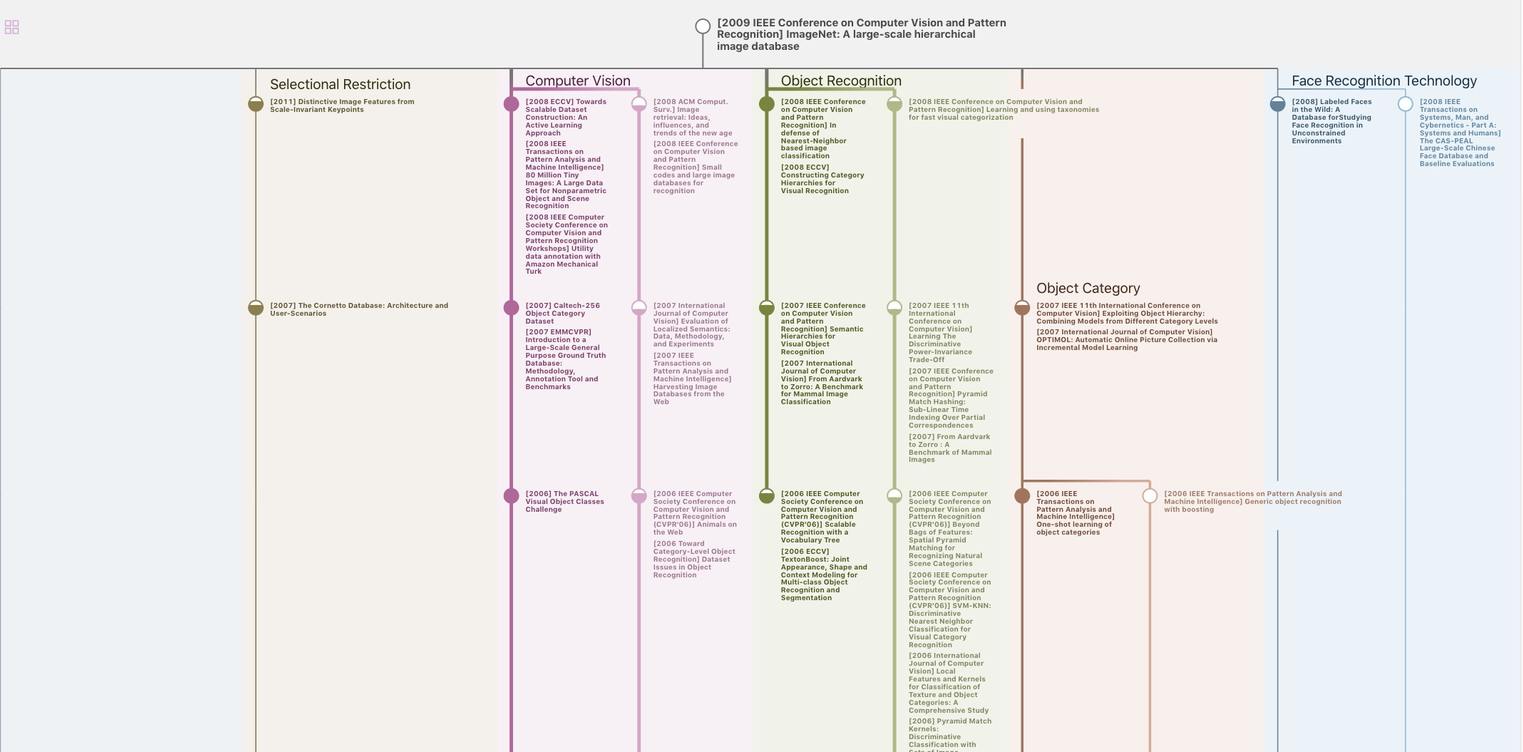
生成溯源树,研究论文发展脉络
Chat Paper
正在生成论文摘要