Interactive Programmatic Labeling for Weak Supervision
user-5ebe28444c775eda72abcdcf(2019)
摘要
The standard supervised machine learning pipeline involves labeling individual training data points, which is often prohibitively slow and expensive. New programmatic or weak supervision approaches expedite this process by having users instead write labeling functions, simple rules or other heuristic functions that label subsets of a dataset. While these types of programmatic labeling approaches can provide significant advantages over labeling training sets by hand, there is usually little formal structure or guidance for how these labeling functions are created by users. We perform an initial exploration of processes through which users can be guided by asking them to write labeling functions over specifically-chosen subsets of the data. This can be viewed as a new form of active learning—a traditional technique wherein data points are intelligently chosen for labeling—applied at the labeling function level. We show in synthetic and real-world experiments how two simple labeling function acquisition strategies outperform a random baseline. In our real-world experiment we observe a 1-2% increase in accuracy after the first 100 labeling functions when using our acquisition strategies, which corresponds to a 2× reduction in the amount of data required to achieve a fixed accuracy.
更多查看译文
AI 理解论文
溯源树
样例
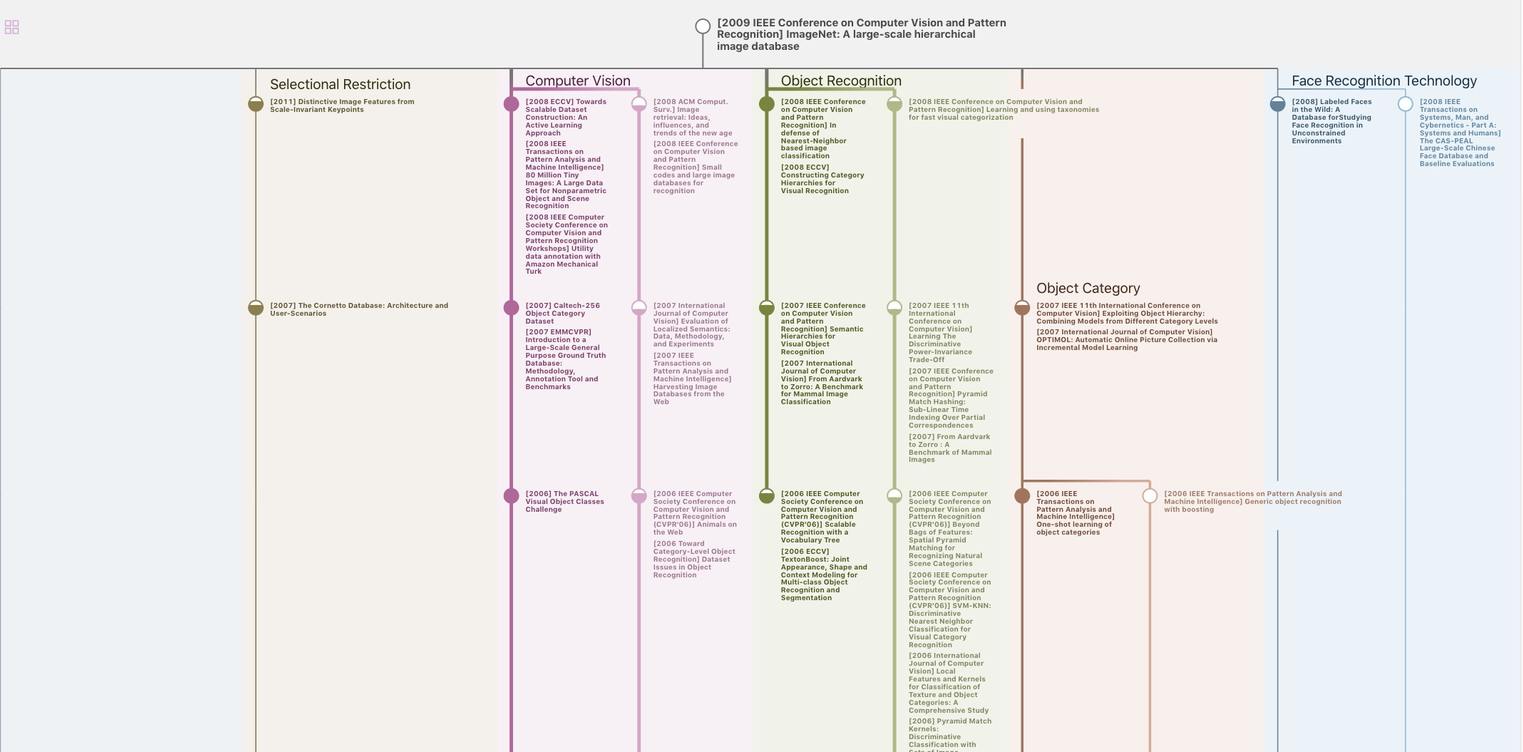
生成溯源树,研究论文发展脉络
Chat Paper
正在生成论文摘要