Unsupervised Feature Learning by Autoencoder and Prototypical Contrastive Learning for Hyperspectral Classification
NEUROCOMPUTING(2021)
Abstract
Hyperspectral classification is a fundamental problem for applying hyperspectral technology, and unsupervised learning is a promising direction to address the issue. Unsupervised feature learning algorithm extracts representative features, which help efficiently classify the hyperspectral pixels. This paper combines the popular contrastive learning method (prototypical contrastive learning) and the classic representation learning method (autoencoder) to design an unsupervised feature learning network for hyperspectral classification. First, two different encoders are used to extract different features as augmentation function in a contrastive network (ContrastNet). Second, the prototypical contrastive learning method is adopted to train a contrastive network. Third, features extracted by the contrastive network are used for classification. Experiments have proved that our two proposed autoencoder networks show good feature learning capabilities by themselves and the designed contrastive learning network can further learn better representative features from the two modules. The three modules compose an efficient framework for unsupervised feature learning. Our method also performs reasonably fast in the testing phase, which implies that it is applicable in practice under specific conditions. (C) 2021 Elsevier B.V. All rights reserved.
MoreTranslated text
Key words
Unsupervised learning,Autoencoder,Contrastive learning,Hyperspectral imagery classification
AI Read Science
Must-Reading Tree
Example
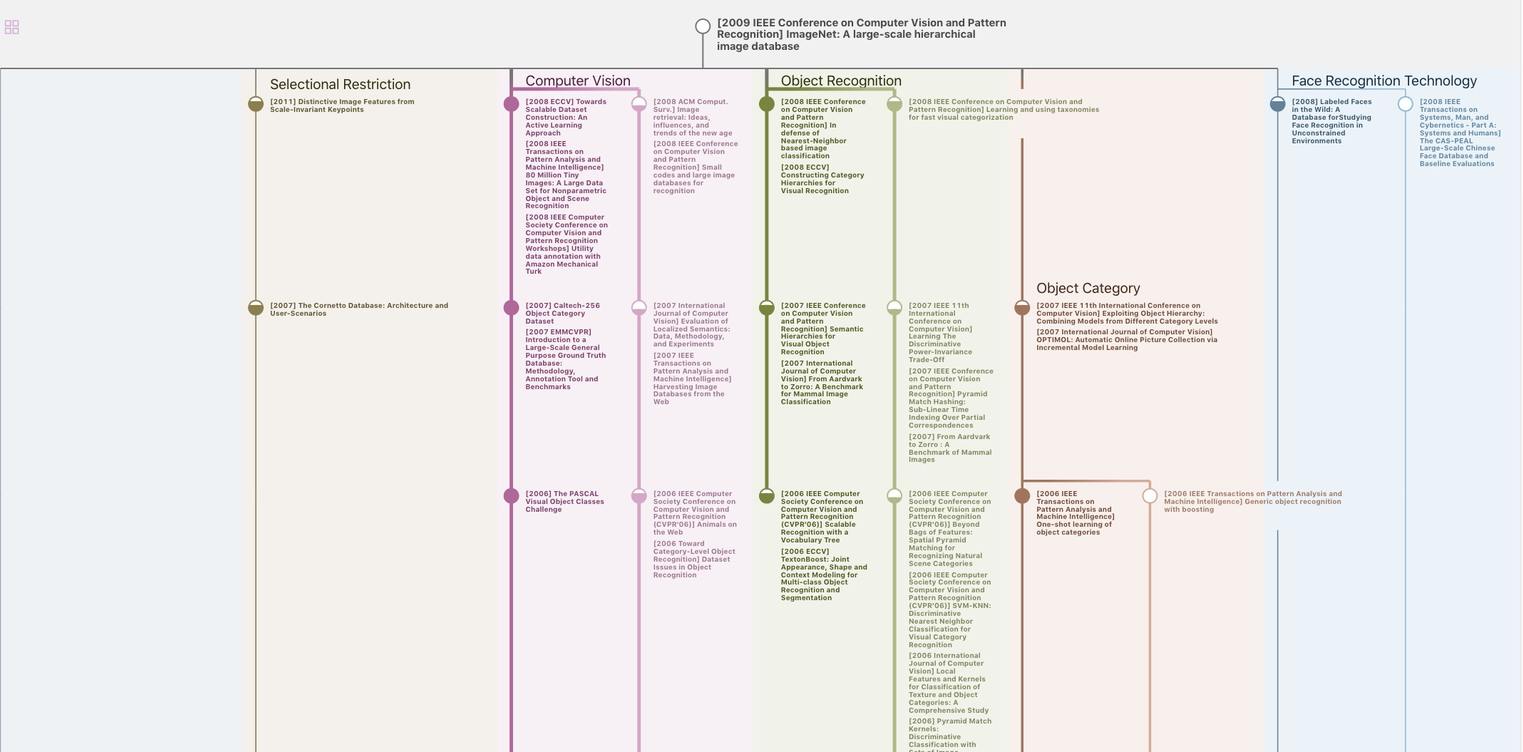
Generate MRT to find the research sequence of this paper
Chat Paper
Summary is being generated by the instructions you defined