Approximating ( K,ℓ )-Median Clustering for Polygonal Curves
ACM TRANSACTIONS ON ALGORITHMS(2023)
摘要
In 2015, Driemel, Krivošija, and Sohler introduced the k,ℓ -median clustering problem for polygonal curves under the Fréchet distance. Given a set of input curves, the problem asks to find k median curves of at most ℓ vertices each that minimize the sum of Fréchet distances over all input curves to their closest median curve. A major shortcoming of their algorithm is that the input curves are restricted to lie on the real line. In this article, we present a randomized bicriteria-approximation algorithm that works for polygonal curves in ℝ d and achieves approximation factor (1+ɛ) with respect to the clustering costs. The algorithm has worst-case running time linear in the number of curves, polynomial in the maximum number of vertices per curve (i.e., their complexity), and exponential in d , ℓ, 1/ɛ and 1/δ (i.e., the failure probability). We achieve this result through a shortcutting lemma, which guarantees the existence of a polygonal curve with similar cost as an optimal median curve of complexity ℓ, but of complexity at most 2ℓ -2, and whose vertices can be computed efficiently. We combine this lemma with the superset sampling technique by Kumar et al. to derive our clustering result. In doing so, we describe and analyze a generalization of the algorithm by Ackermann et al., which may be of independent interest.
更多查看译文
关键词
Approximation Algorithms,Clustering Algorithms,Geometric Optimization,Top-k Query Processing
AI 理解论文
溯源树
样例
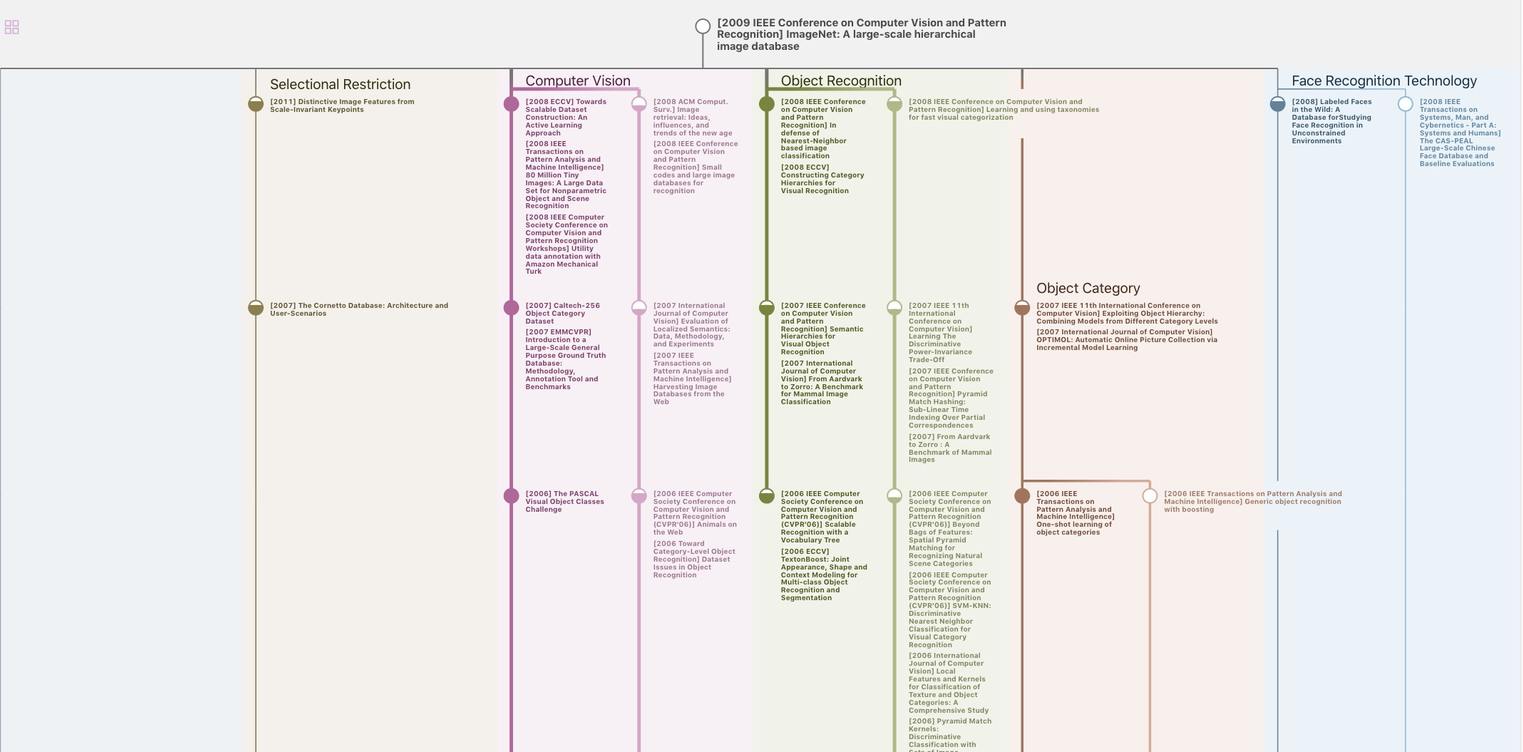
生成溯源树,研究论文发展脉络
Chat Paper
正在生成论文摘要