Fairness in the Eyes of the Data: Certifying Machine-Learning Models
AIES '21: PROCEEDINGS OF THE 2021 AAAI/ACM CONFERENCE ON AI, ETHICS, AND SOCIETY(2021)
摘要
We present a framework that allows to certify the fairness degree of a model based on an interactive and privacy-preserving test. The framework verifies any trained model, regardless of its training process and architecture. Thus, it allows us to evaluate any deep learning model on multiple fairness definitions empirically. We tackle two scenarios, where either the test data is privately available only to the tester or is publicly known in advance, even to the model creator. We investigate the soundness of the proposed approach using theoretical analysis and present statistical guarantees for the interactive test. Finally, we provide a cryptographic technique to automate fairness testing and certified inference with only blackbox access to the model at hand while hiding the participants' sensitive data.
更多查看译文
关键词
Fairness, Privacy
AI 理解论文
溯源树
样例
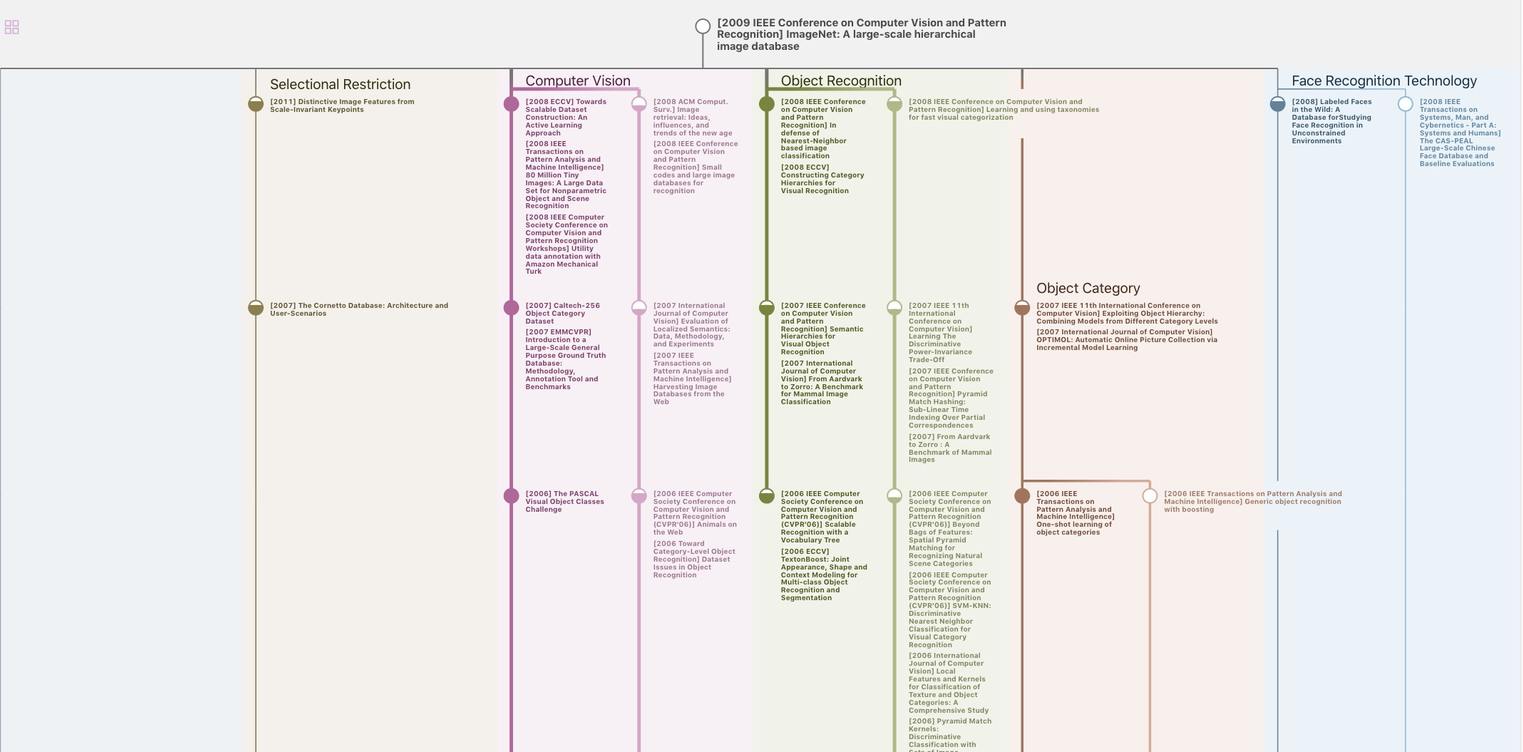
生成溯源树,研究论文发展脉络
Chat Paper
正在生成论文摘要