Process Mining Meets Causal Machine Learning: Discovering Causal Rules from Event Logs
2020 2nd International Conference on Process Mining (ICPM)(2020)
摘要
This paper proposes an approach to analyze an event log of a business process in order to generate case-level recommendations of treatments that maximize the probability of a given outcome. Users classify the attributes in the event log into controllable and non-controllable, where the former correspond to attributes that can be altered during an execution of the process (the possible treatments). We use an action rule mining technique to identify treatments that co-occur with the outcome under some conditions. Since action rules are generated based on correlation rather than causation, we then use a causal machine learning technique, specifically uplift trees, to discover subgroups of cases for which a treatment has a high causal effect on the outcome after adjusting for confounding variables. We test the relevance of this approach using an event log of a loan application process and compare our findings with recommendations manually produced by process mining experts.
更多查看译文
关键词
process mining,causal ML,uplift modeling
AI 理解论文
溯源树
样例
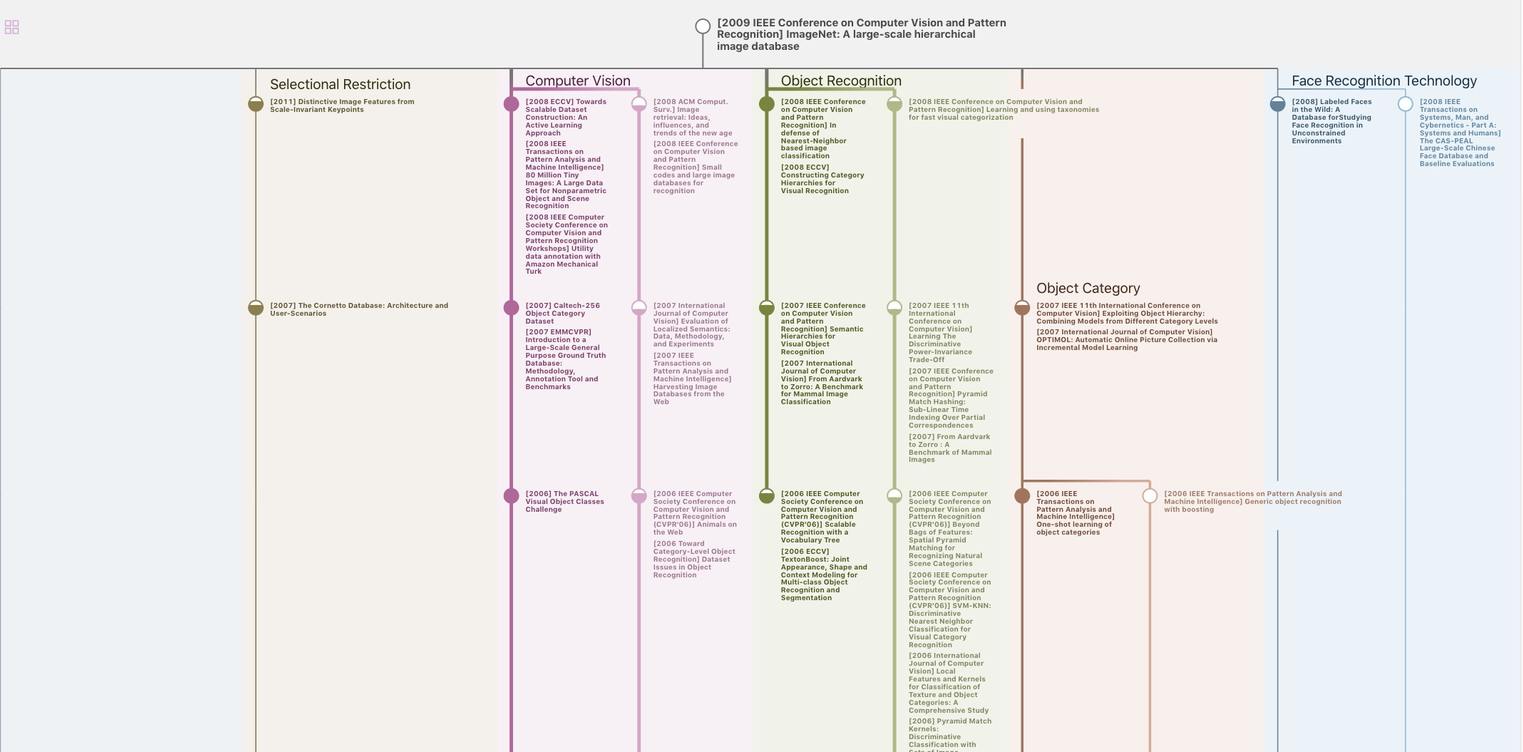
生成溯源树,研究论文发展脉络
Chat Paper
正在生成论文摘要