Energy-Efficient Hardware for Language Guided Reinforcement Learning
IEEE Design & Test(2022)
摘要
Reinforcement learning (RL) has shown great performance in solving sequential decision-making problems. While a lot of works have done on processing state information such as images, there has been some effort towards integrating natural language instructions into RL. In this paper, we propose an energy-efficient architecture which is designed to receive both images and text inputs as a step towards designing RL agents that can understand human language and act in real-world environments. Different configurations are proposed to illustrate the trade off between the number of parameters and the model accuracy, and a custom low power hardware is designed and implemented on FPGA based on the best configuration. The hardware designed to be configurable with different parameters such as number of processing elements, so that it can easily balance power and performance. The high throughput configuration achieves 217 frames per second throughput with 1.2 mJ energy consumption per classification on Xilinx Artix-7 FPGA, while the low power configuration consumes less than 139 mW for 30 frames per second classification. Compared to the similar works using FPGA for hardware implementation, our design is more energy efficient and need less energy for generating each output.
更多查看译文
关键词
Reinforcement Learning,Power Optimization,FPGA,Dynamic Load Balancing
AI 理解论文
溯源树
样例
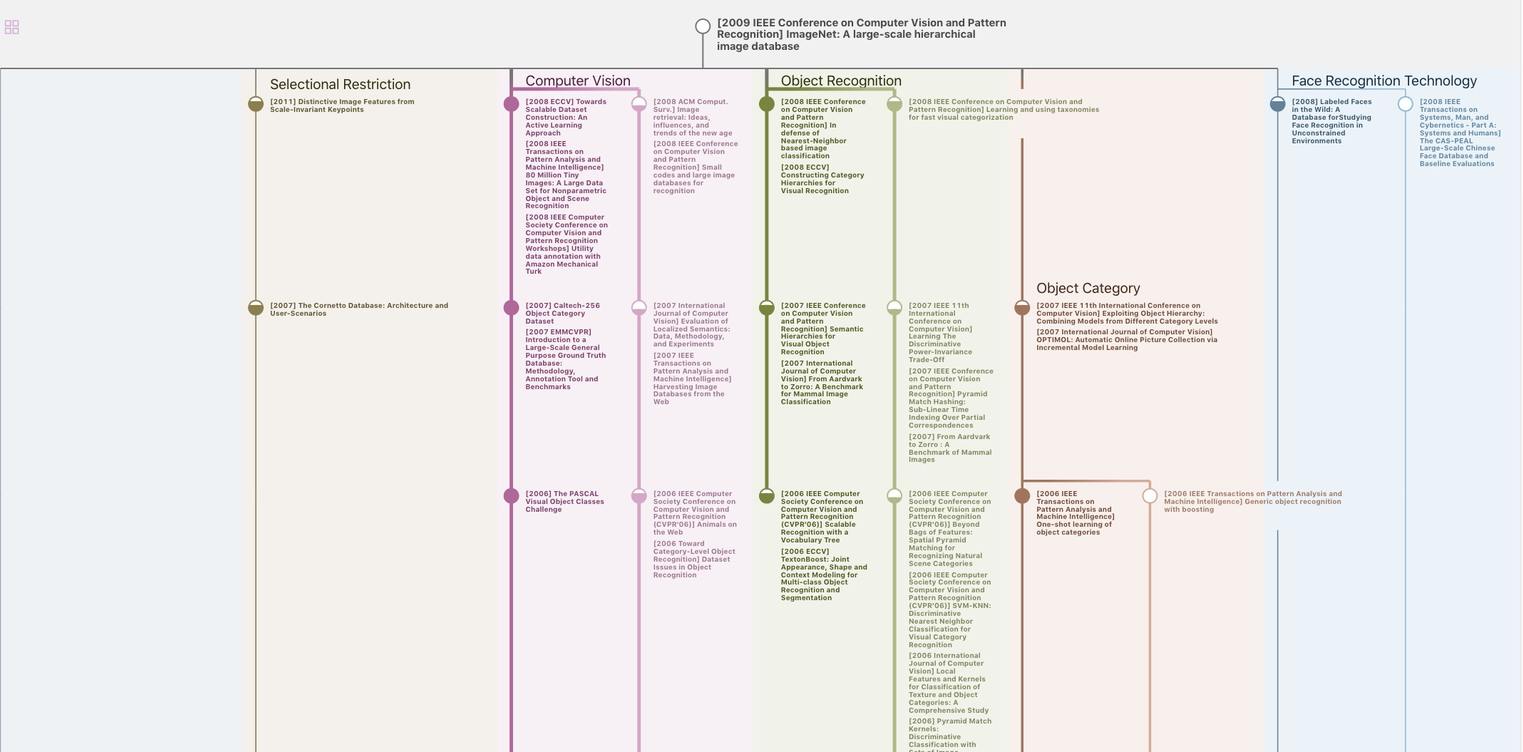
生成溯源树,研究论文发展脉络
Chat Paper
正在生成论文摘要