A Comprehensive Analysis of Information Leakage in Deep Transfer Learning
arxiv(2020)
摘要
Transfer learning is widely used for transferring knowledge from a source domain to the target domain where the labeled data is scarce. Recently, deep transfer learning has achieved remarkable progress in various applications. However, the source and target datasets usually belong to two different organizations in many real-world scenarios, potential privacy issues in deep transfer learning are posed. In this study, to thoroughly analyze the potential privacy leakage in deep transfer learning, we first divide previous methods into three categories. Based on that, we demonstrate specific threats that lead to unintentional privacy leakage in each category. Additionally, we also provide some solutions to prevent these threats. To the best of our knowledge, our study is the first to provide a thorough analysis of the information leakage issues in deep transfer learning methods and provide potential solutions to the issue. Extensive experiments on two public datasets and an industry dataset are conducted to show the privacy leakage under different deep transfer learning settings and defense solution effectiveness.
更多查看译文
关键词
deep transfer
AI 理解论文
溯源树
样例
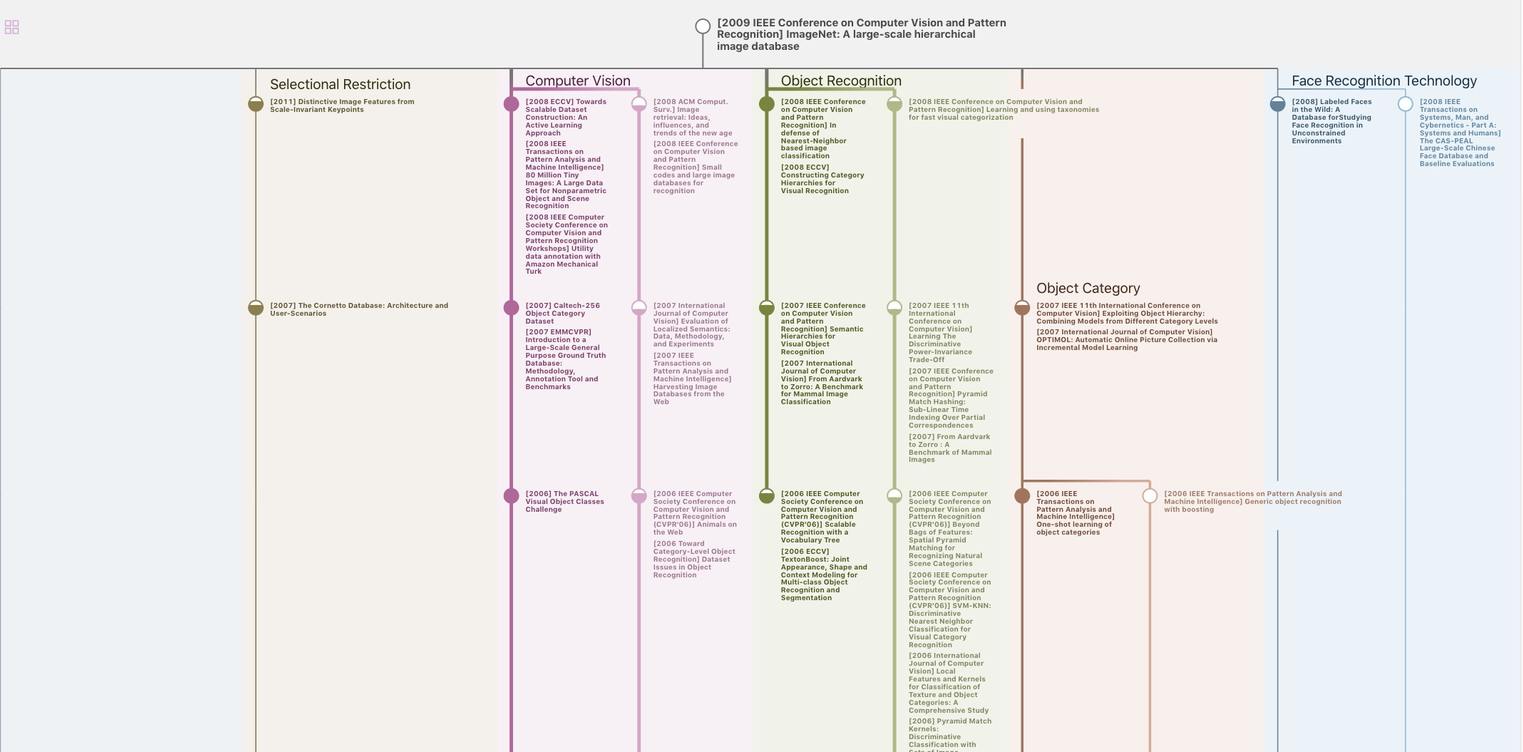
生成溯源树,研究论文发展脉络
Chat Paper
正在生成论文摘要