Learning Topological Motion Primitives for Knot Planning
IROS(2020)
摘要
In this paper, we approach the challenging problem of motion planning for knot tying. We propose a hierarchical approach in which the top layer produces a topological plan and the bottom layer translates this plan into continuous robot motion. The top layer decomposes a knotting task into sequences of abstract topological actions based on knot theory. The bottom layer translates each of these abstract actions into robot motion trajectories through learned topological motion primitives. To adapt each topological action to the specific rope geometry, the motion primitives take the observed rope configuration as input. We train the motion primitives by imitating human demonstrations and reinforcement learning in simulation. To generalize human demonstrations of simple knots into more complex knots, we observe similarities in the motion strategies of different topological actions and design the neural network structure to exploit such similarities. We demonstrate that our learned motion primitives can be used to efficiently generate motion plans for tying the overhand knot. The motion plan can then be executed on a real robot using visual tracking and Model Predictive Control. We also demonstrate that our learned motion primitives can be composed to tie a more complex pentagram-like knot despite being only trained on human demonstrations of simpler knots.
更多查看译文
关键词
robot motion trajectories,learned topological motion primitives,motion strategies,learned motion primitives,motion plan,complex pentagram-like knot,knot planning,motion planning,knot tying,topological plan,continuous robot motion,knotting task,topological actions,knot theory,visual tracking,model predictive control,neural network structure
AI 理解论文
溯源树
样例
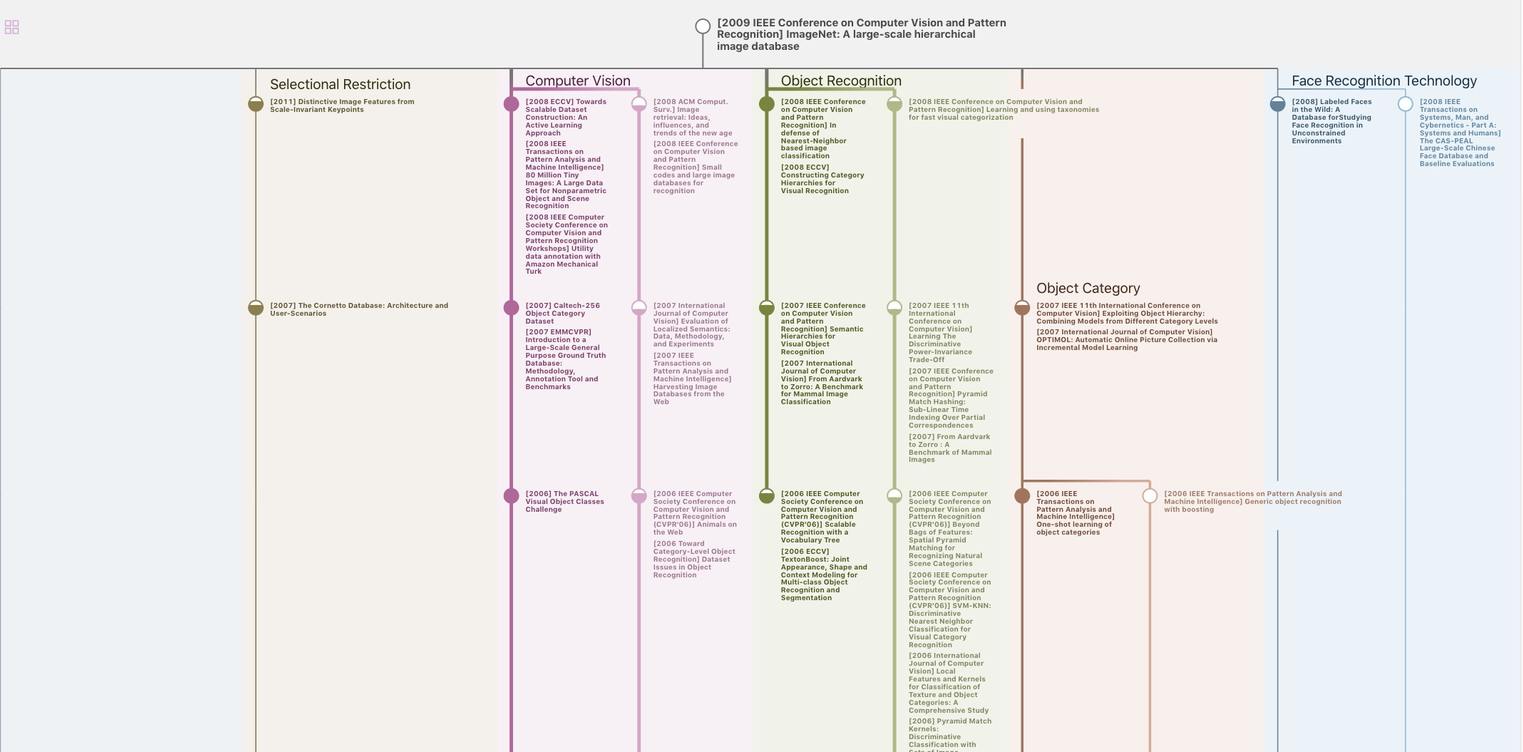
生成溯源树,研究论文发展脉络
Chat Paper
正在生成论文摘要