Linear predictive coding distinguishes spectral EEG features of Parkinson's disease.
PARKINSONISM & RELATED DISORDERS(2020)
摘要
Objective: We have developed and validated a novel EEG-based signal processing approach to distinguish PD and control patients: Linear-predictive-coding EEG Algorithm for PD (LEAPD). This method efficiently encodes EEG time series into features that can detect PD in a computationally fast manner amenable to real time applications. Methods: We included a total of 41 PD patients and 41 demographically-matched controls from New Mexico and Iowa. Data for all participants from New Mexico (27 PD patients and 27 controls) were used to evaluate in-sample LEAPD performance, with extensive cross-validation. Participants from Iowa (14 PD patients and 14 controls) were used for out-of-sample tests. Our method utilized data from six EEG leads which were as little as 2 min long. Results: For the in-sample dataset, LEAPD differentiated PD patients from controls with 85.3 +/- 0.1% diagnostic accuracy, 93.3 +/- 0.5% area under the receiver operating characteristics curve (AUC), 87.9 +/- 0.9% sensitivity, and 82.7 +/- 1.1% specificity, with multiple cross-validations. After head-to-head comparison with state-of-the-art methods using our dataset, LEAPD showed a 13% increase in accuracy and a 15.5% increase in AUC. When the trained classifier was applied to a distinct out-of-sample dataset, LEAPD showed reliable performance with 85.7% diagnostic accuracy, 85.2% AUC, 85.7% sensitivity, and 85.7% specificity. No statistically significant effect of levodopa-ON and levodopa-OFF sessions were found. Conclusion: We describe LEAPD, an efficient algorithm that is suitable for real time application and captures spectral EEG features using few parameters and reliably differentiates PD patients from demographically-matched controls.
更多查看译文
关键词
EEG,Parkinson's disease,Diagnosis,Classifier
AI 理解论文
溯源树
样例
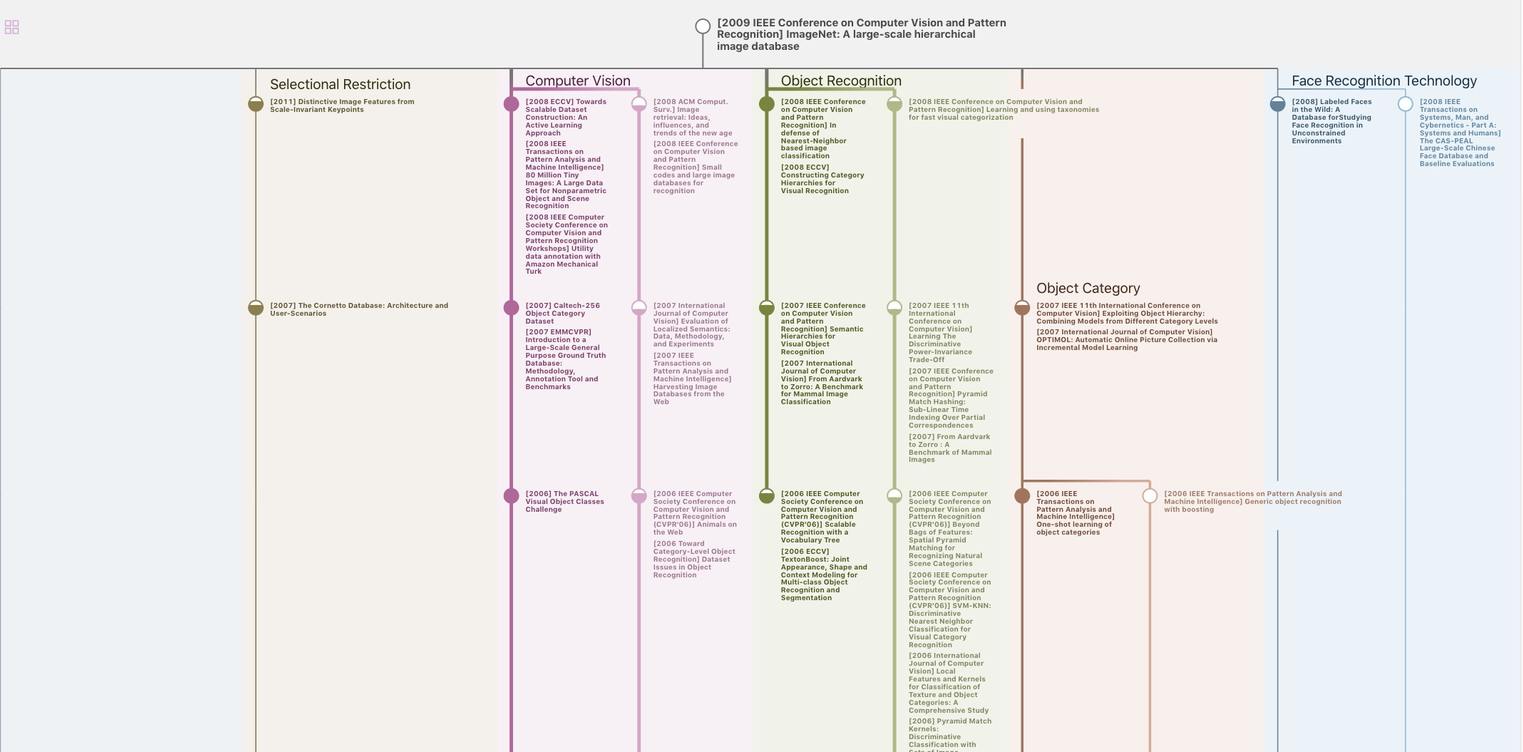
生成溯源树,研究论文发展脉络
Chat Paper
正在生成论文摘要