Analysis Of Kohn-Sham Eigenfunctions Using A Convolutional Neural Network In Simulations Of The Metal-Insulator Transition In Doped Semiconductors
JOURNAL OF THE PHYSICAL SOCIETY OF JAPAN(2021)
摘要
Machine learning has recently been applied to many problems in condensed matter physics. A common point of many proposals is to save computational cost by training the machine with data from a simple example and then using the machine to make predictions for a more complicated example. Convolutional neural networks (CNN), which are one of the tools of machine learning, have proved to work well for assessing eigenfunctions in disordered systems. Here we apply a CNN to assess Kohn-Sham eigenfunctions obtained in density functional theory (DFT) simulations of the metal-insulator transition of a doped semiconductor. We demonstrate that a CNN that has been trained using eigenfunctions from a simulation of a doped semiconductor that neglects electron spin successfully predicts the critical concentration when presented with eigenfunctions from simulations that include spin.
更多查看译文
关键词
doped semiconductors,convolutional neural network,kohn-sham,metal-insulator
AI 理解论文
溯源树
样例
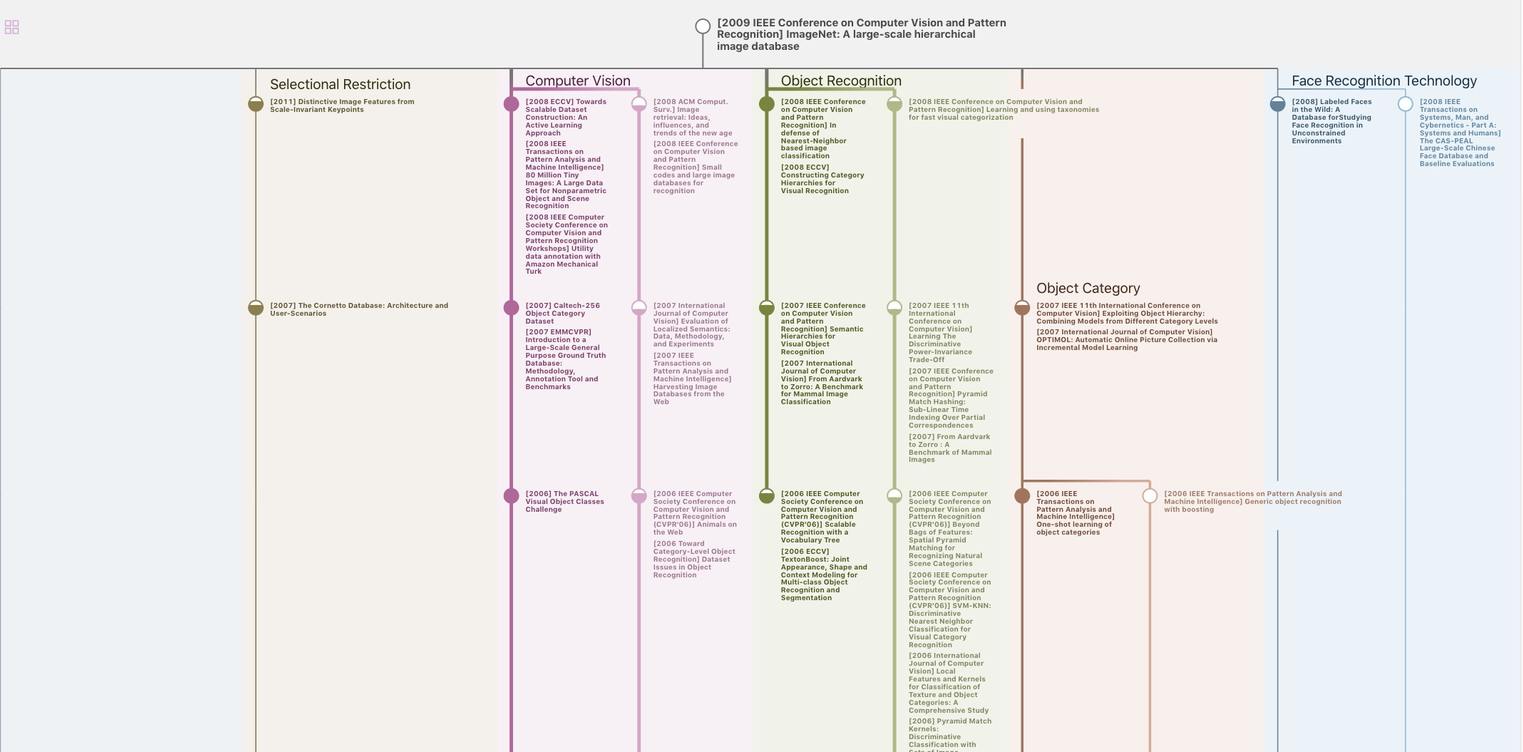
生成溯源树,研究论文发展脉络
Chat Paper
正在生成论文摘要