Information Theoretic Meta Learning with Gaussian Processes
arxiv(2020)
摘要
We formulate meta learning using information theoretic concepts such as mutual information and the information bottleneck. The idea is to learn a stochastic representation or encoding of the task description, given by a training or support set, that is highly informative about predicting the validation set. By making use of variational approximations to the mutual information we derive a general and tractable framework for meta learning. We particularly develop new memory-based meta learning algorithms based on Gaussian processes and derive extensions that combine memory and gradient based meta learning. We demonstrate our method on few-shot regression and classification by using standard benchmarks such as Omniglot, mini-Imagenet and Augmented Omniglot.
更多查看译文
关键词
meta learning,information,processes
AI 理解论文
溯源树
样例
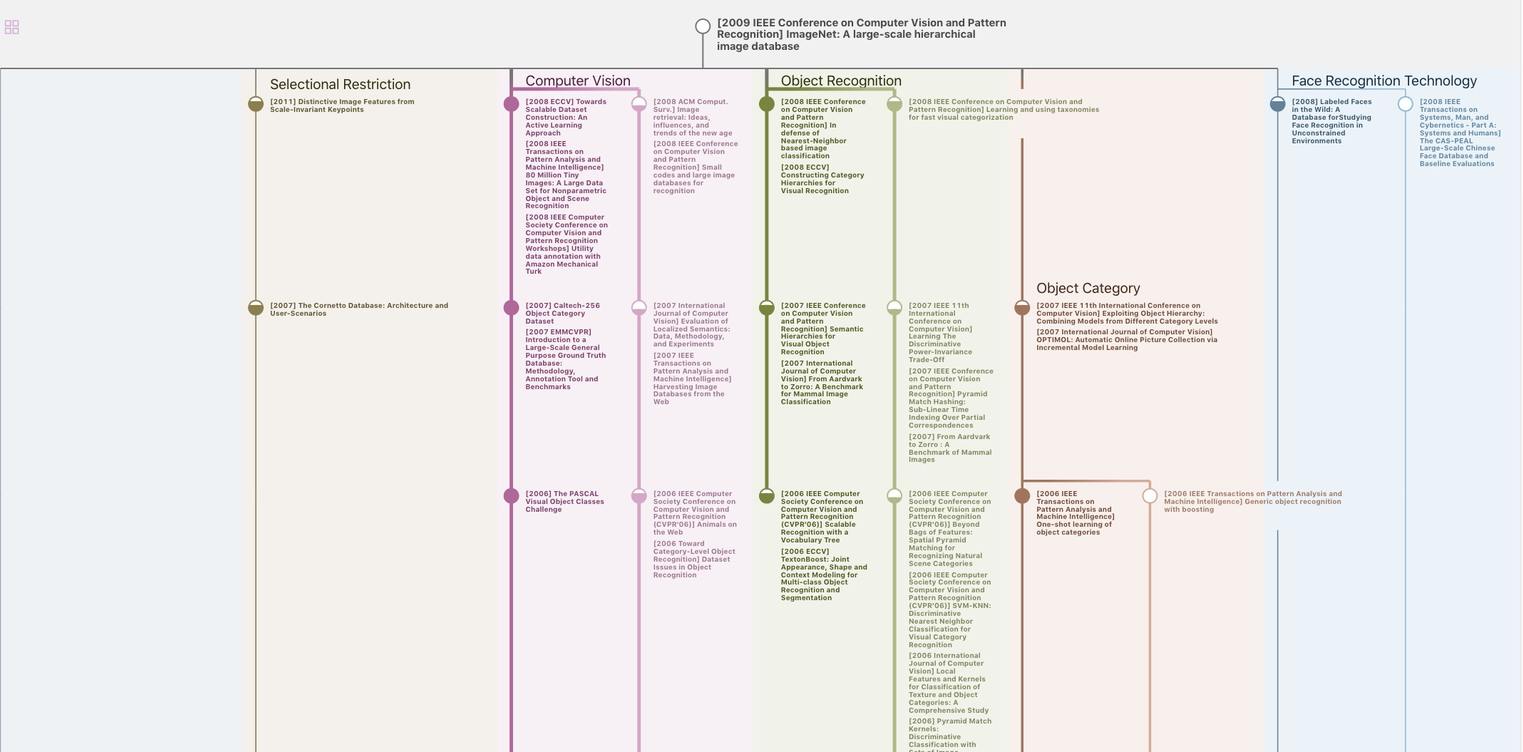
生成溯源树,研究论文发展脉络
Chat Paper
正在生成论文摘要