Experimentally Driven Automated Machine-Learned Interatomic Potential For A Refractory Oxide
arxiv(2021)
摘要
Understanding the structure and properties of refractory oxides is critical for high temperature applications. In this work, a combined experimental and simulation approach uses an automated closed loop via an active learner, which is initialized by x-ray and neutron diffraction measurements, and sequentially improves a machine-learning model until the experimentally predetermined phase space is covered. A multiphase potential is generated for a canonical example of the archetypal refractory oxide, HfO2, by drawing a minimum number of training configurations from room temperature to the liquid state at similar to 2900 degrees C. The method significantly reduces model development time and human effort.
更多查看译文
关键词
refractory oxide,potential,machine-learned
AI 理解论文
溯源树
样例
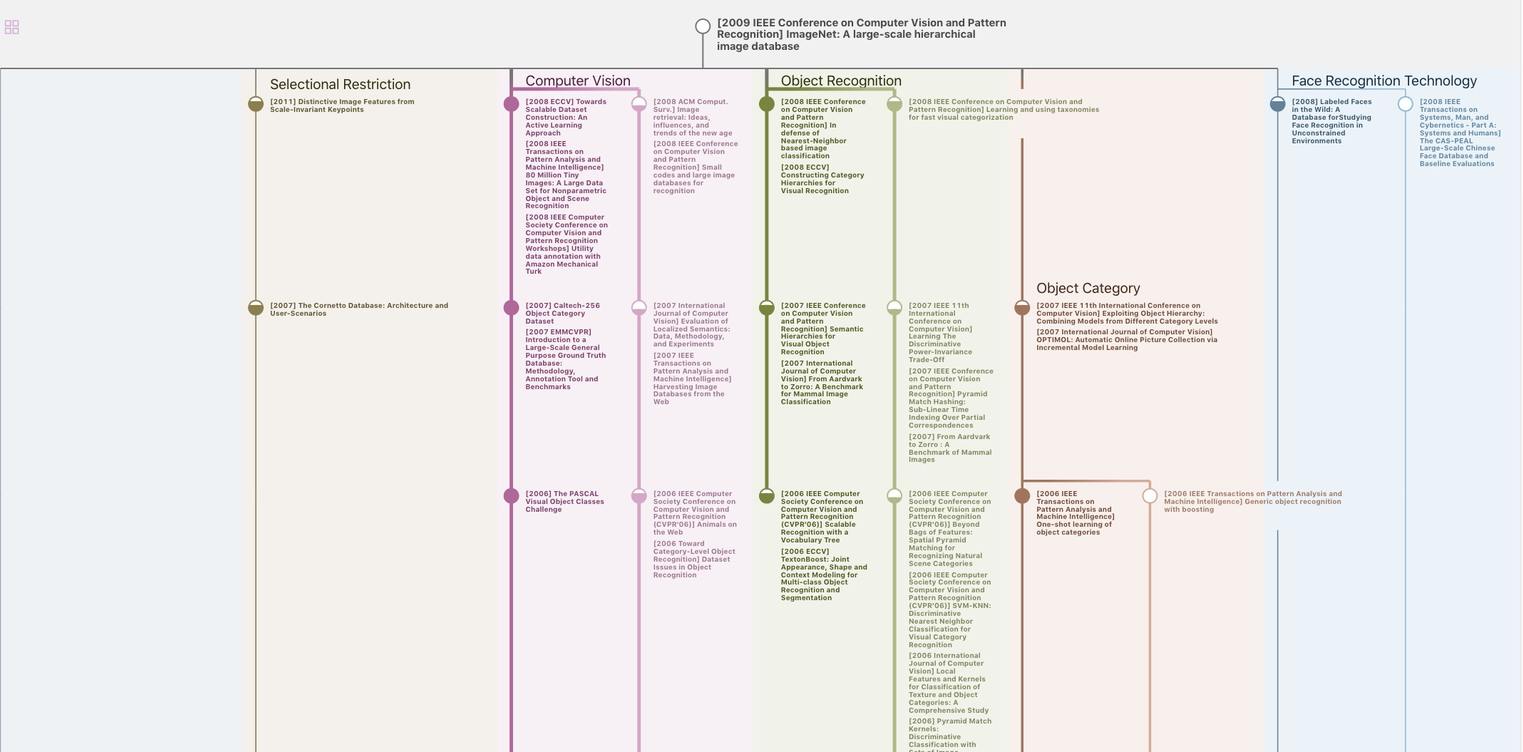
生成溯源树,研究论文发展脉络
Chat Paper
正在生成论文摘要