Pay Attention when Required
arxiv(2020)
摘要
Transformer-based models consist of interleaved feed-forward blocks - that capture content meaning, and relatively more expensive self-attention blocks - that capture context meaning. In this paper, we explored trade-offs and ordering of the blocks to improve upon the current Transformer architecture and proposed PAR Transformer. It needs 35% lower compute time than Transformer-XL achieved by replacing ~63% of the self-attention blocks with feed-forward blocks, and retains the perplexity on WikiText-103 language modelling benchmark. We further validated our results on text8 and enwiki8 datasets, as well as on the BERT model.
更多查看译文
关键词
attention
AI 理解论文
溯源树
样例
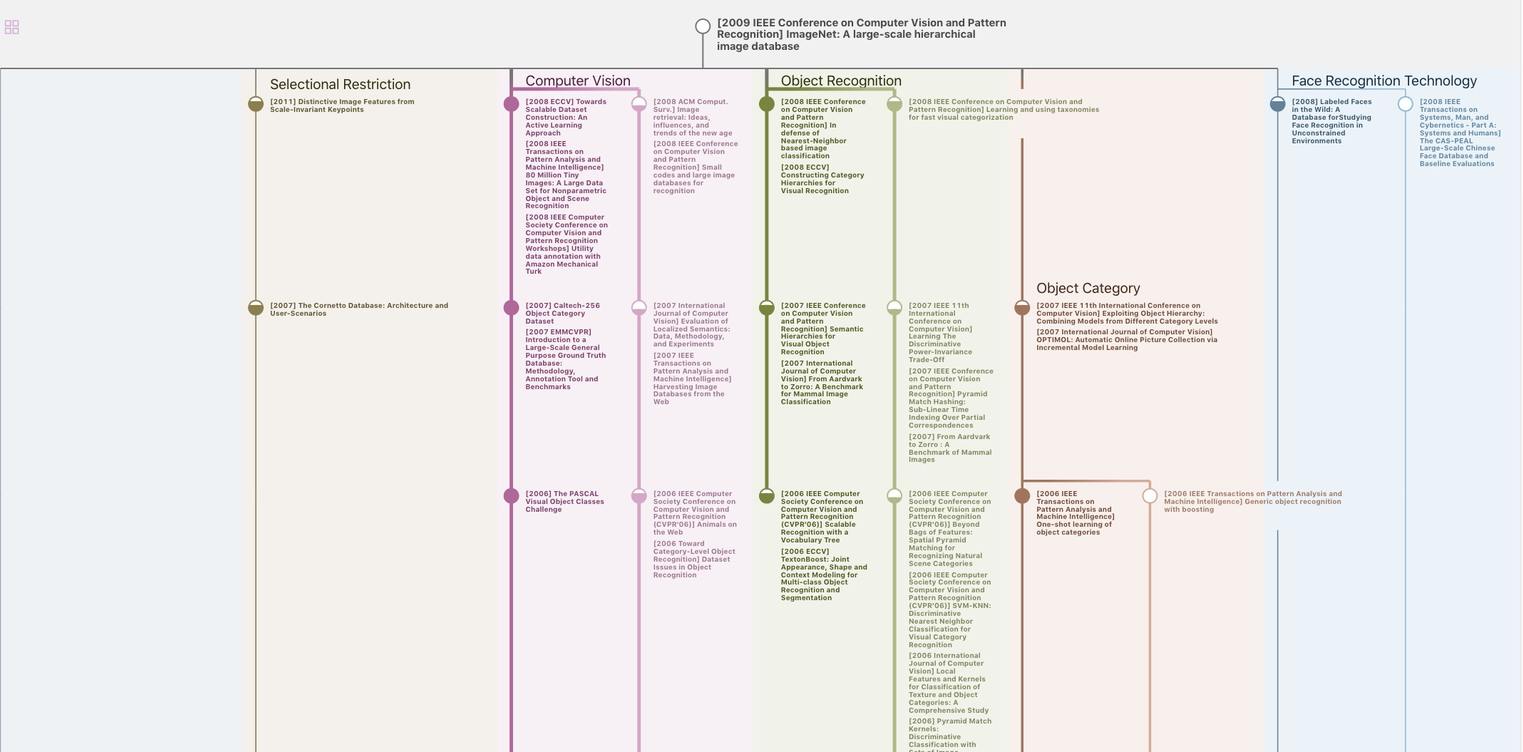
生成溯源树,研究论文发展脉络
Chat Paper
正在生成论文摘要