Macro neural architecture search revisited
2nd Workshop on Meta-Learning at NeurIPS(2018)
摘要
Neural architecture search (NAS) has recently shown promising results for automatically finding cost-efficient and accurate predictors. However, most recent work exclusively study the small search space of repeatable network modules (cells), instead of the more general overall network (macro), partially because the models found by macro-search typically require an order of magnitude more parameters compared to cell-search to have the same accuracy. In this work, we show through ablation study that this gap mainly exists due to the difference in initial models. In fact, by starting with the same condition as cell-search, the proposed macro-search can find a CIFAR-10 model that has 2.93% test error rate and uses 3.1 million parameters. Our macro-search algorithm has the advantage of being simple and fast. The search procedure randomly and incrementally grows the most cost-efficient models on the Pareto frontier. The proposed search takes only 6 GPU-days, which is much smaller than those of many existing methods, while achieving comparable or better results.
更多查看译文
AI 理解论文
溯源树
样例
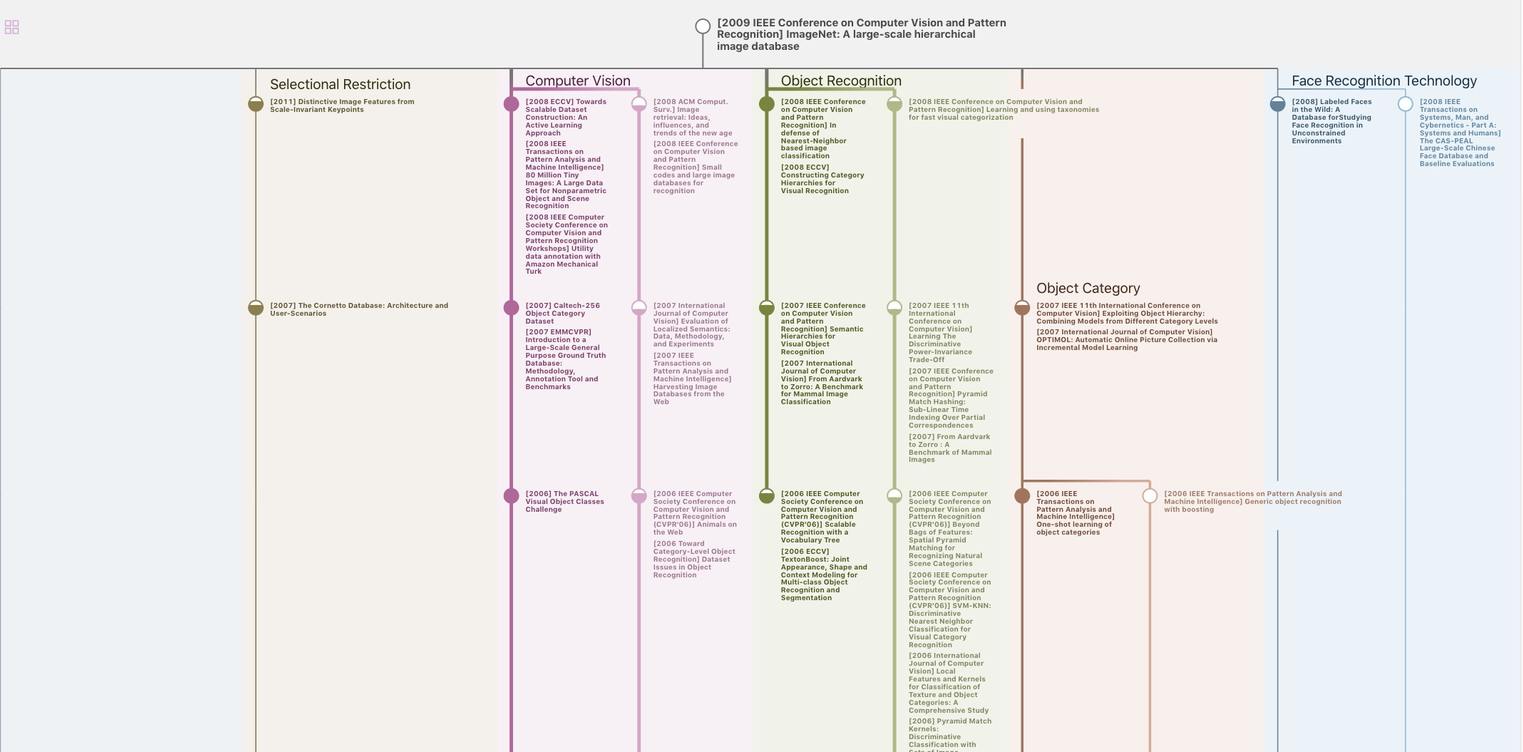
生成溯源树,研究论文发展脉络
Chat Paper
正在生成论文摘要