Dynamic Future Net: Diversified Human Motion Generation
MM '20: The 28th ACM International Conference on Multimedia Seattle WA USA October, 2020(2020)
摘要
Human motion modelling is crucial in many areas such as computergraphics, vision and virtual reality. Acquiring high-quality skele-tal motions is difficult due to the need for specialized equipmentand laborious manual post-posting, which necessitates maximiz-ing the use of existing data to synthesize new data. However, it is a challenge due to the intrinsic motion stochasticity of humanmotion dynamics, manifested in the short and long terms. In theshort term, there is strong randomness within a couple frames, e.g.one frame followed by multiple possible frames leading to differentmotion styles; while in the long term, there are non-deterministicaction transitions. In this paper, we present Dynamic Future Net,a new deep learning model where we explicitly focuses on the aforementioned motion stochasticity by constructing a generative model with non-trivial modelling capacity in temporal stochas-ticity. Given limited amounts of data, our model can generate a large number of high-quality motions with arbitrary duration, andvisually-convincing variations in both space and time. We evaluateour model on a wide range of motions and compare it with the state-of-the-art methods. Both qualitative and quantitative results show the superiority of our method, for its robustness, versatility and high-quality.
更多查看译文
关键词
human motion, neural networks, generative models
AI 理解论文
溯源树
样例
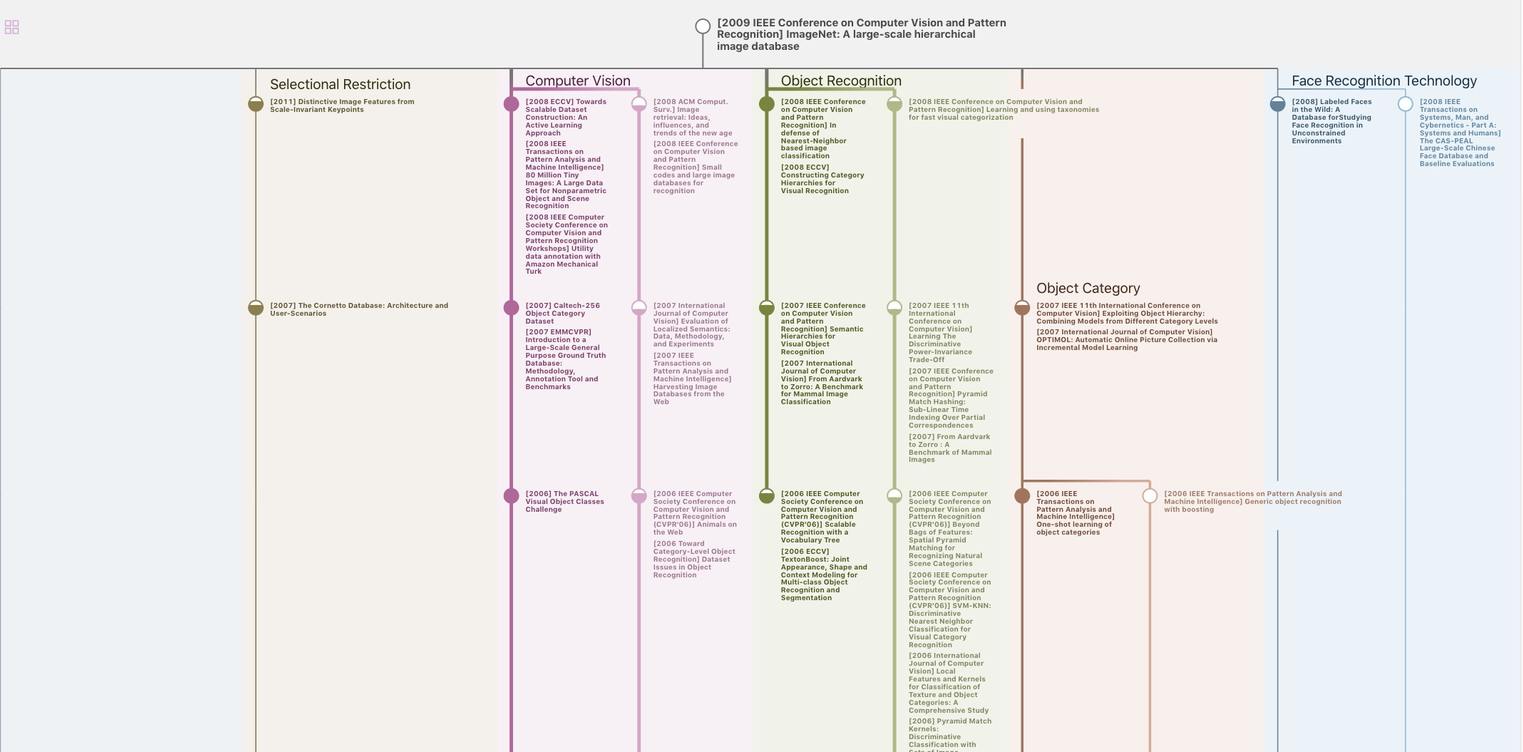
生成溯源树,研究论文发展脉络
Chat Paper
正在生成论文摘要