Augmented pseudo-marginal Metropolis–Hastings for partially observed diffusion processes
STATISTICS AND COMPUTING(2022)
摘要
We consider the problem of inference for nonlinear, multivariate diffusion processes, satisfying Itô stochastic differential equations (SDEs), using data at discrete times that may be incomplete and subject to measurement error. Our starting point is a state-of-the-art correlated pseudo-marginal Metropolis–Hastings algorithm, that uses correlated particle filters to induce strong and positive correlation between successive likelihood estimates. However, unless the measurement error or the dimension of the SDE is small, correlation can be eroded by the resampling steps in the particle filter. We therefore propose a novel augmentation scheme, that allows for conditioning on values of the latent process at the observation times, completely avoiding the need for resampling steps. We integrate over the uncertainty at the observation times with an additional Gibbs step. Connections between the resulting pseudo-marginal scheme and existing inference schemes for diffusion processes are made, giving a unified inference framework that encompasses Gibbs sampling and pseudo marginal schemes. The methodology is applied in three examples of increasing complexity. We find that our approach offers substantial increases in overall efficiency, compared to competing methods
更多查看译文
关键词
Stochastic differential equation,Bayesian inference,Pseudo-marginal Metropolis–Hastings,Data augmentation,Linear noise approximation
AI 理解论文
溯源树
样例
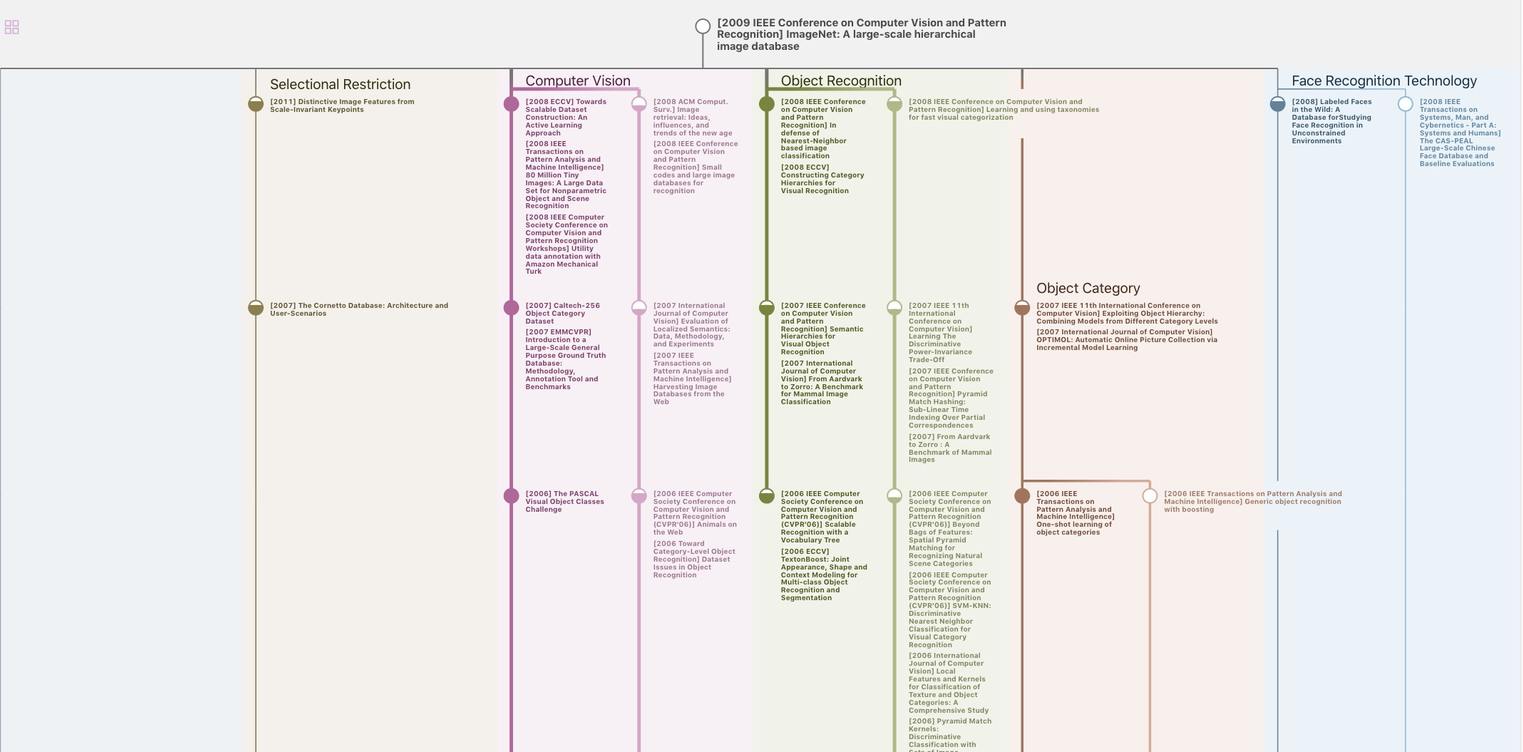
生成溯源树,研究论文发展脉络
Chat Paper
正在生成论文摘要