A window-based sequence-to-one approach with dynamic voting for nurse care activity recognition using acceleration-based wearable sensor
UbiComp/ISWC '20: 2020 ACM International Joint Conference on Pervasive and Ubiquitous Computing and 2020 ACM International Symposium on Wearable Computers Virtual Event Mexico September, 2020(2020)
摘要
This paper introduces a window-based sequence-to-one approach with dynamic voting for nurse care activity recognition using acceleration-based wearable sensors. Nurse care activity recognition is an essential part of ensuring high quality patient care and providing constructive and concrete feedback to the care team. Some of the current sensing approaches for activity recognition include vision-based sensing and non-wearable RF sensing. However, their application is limited in real-life scenarios due to restrictive factors such as perceived privacy and sensitivity to specific occupant paths. To overcome these limitations, acceleration-based wearable sensing have been introduced in recent works. However, the duration distribution of nursing activity instances are biased and skewed. This skewness leads to imbalanced datasets which will result in low performance for the common predictive models. Further, uncertainties such as ambient noise and environmental factors affect the signals and thus can potentially reduce the activity recognition performance. To overcome the first challenge, we separate the signals into short windows with adaptive overlapping ratios for activity instances having different lengths, which balances the label distribution due to event length variations. Further, we use a multi-layer Long Short-Term Memory (LSTM) model to predict nursing activities of each sliding window and introduce a voting-based scheme for complementing the predictions across the signal windows and addressing the uncertainty challenge. We validate our approach through participation in "The 2nd Nurse Care Activity Recognition Challenge Using Lab and Field Data" as team HealthyVibes. On the challenge dataset our model achieves 97.4% and 43.9% accuracy for training and validation, respectively.
更多查看译文
关键词
Wearable Sensors, Activity Recognition, Sequence Model, Nurse Care
AI 理解论文
溯源树
样例
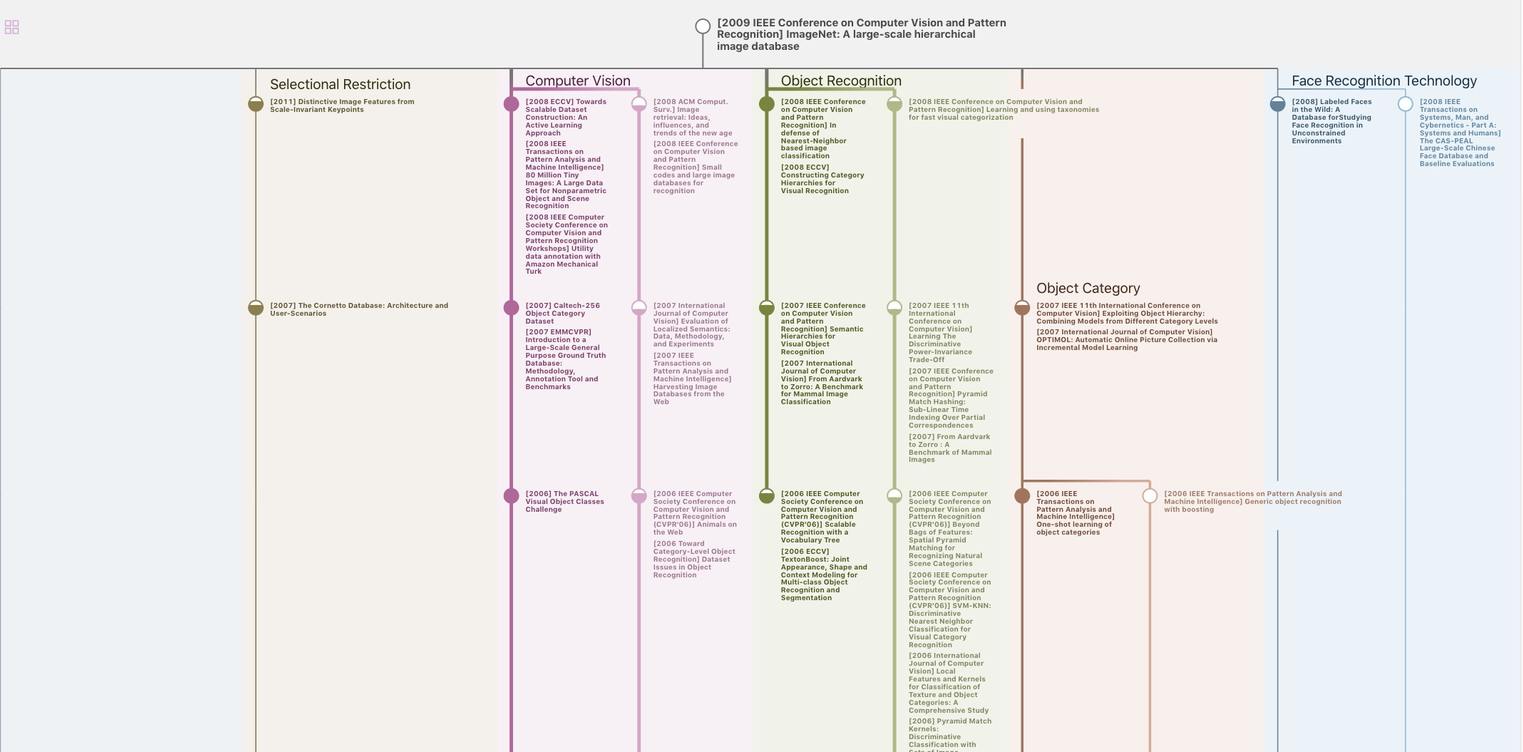
生成溯源树,研究论文发展脉络
Chat Paper
正在生成论文摘要