Tackling the SHL recognition challenge with phone position detection and nearest neighbour smoothing
UbiComp/ISWC '20: 2020 ACM International Joint Conference on Pervasive and Ubiquitous Computing and 2020 ACM International Symposium on Wearable Computers Virtual Event Mexico September, 2020(2020)
摘要
We present the solution of team MDCA to the Sussex-Huawei Locomotion-Transportation (SHL) recognition challenge 2020. The task is to recognize the mode of transportation from 5-second frames of smartphone sensor data from two users, who wore the phone in a constant but unknown position. The training data were collected by a different user with four phones simultaneously worn at four different positions. Only a small labelled dataset from the two "target" users was provided. Our solution consists of three steps: 1) detecting the phone wearing position, 2) selecting training data to create a user and position-specific classification model, and 3) "smoothing" the predictions by identifying groups of similar data frames in the test set, which probably belong to the same class. We demonstrate the effectiveness of the processing pipeline by comparison to baseline models. Using 4-fold cross-validation our approach achieves an average F1 score of 75.3%.
更多查看译文
关键词
activity recognition, transport mode recognition, signal processing, neural networks
AI 理解论文
溯源树
样例
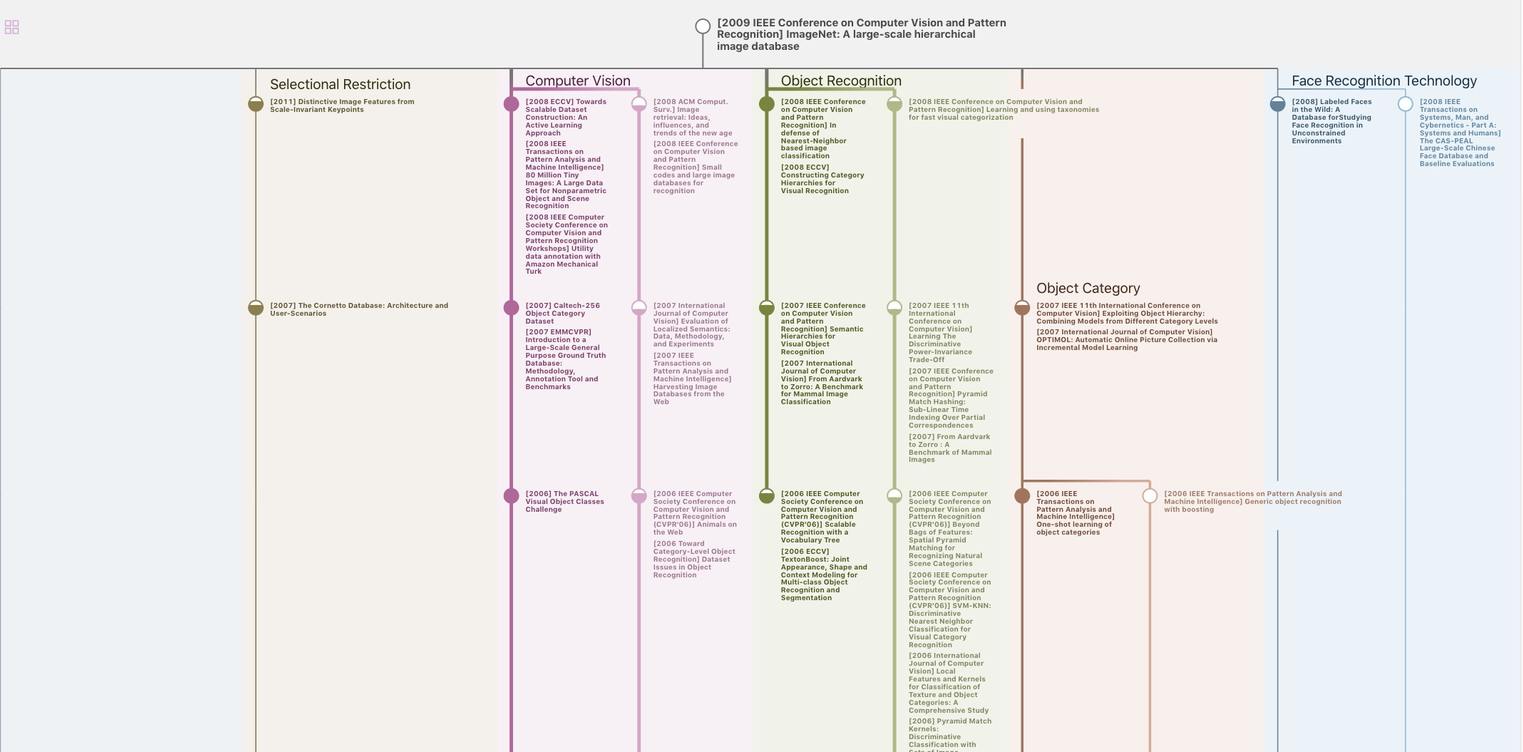
生成溯源树,研究论文发展脉络
Chat Paper
正在生成论文摘要