A Tutorial of Ultra-Reliable and Low-Latency Communications in 6G: Integrating Theoretical Knowledge into Deep Learning
arxiv(2020)
摘要
As one of the key communication scenarios in the 5th and also the 6th generation (6G) cellular networks, ultra-reliable and low-latency communications (URLLC) will be central for the development of various emerging mission-critical applications. The state-of-the-art mobile communication systems do not fulfill the end-to-end delay and overall reliability requirements of URLLC. A holistic framework that takes into account latency, reliability, availability, scalability, and decision-making under uncertainty is lacking. Driven by recent breakthroughs in deep neural networks, deep learning algorithms have been considered as promising ways of developing enabling technologies for URLLC in future 6G networks. This tutorial illustrates how to integrate theoretical knowledge (models, analysis tools, and optimization frameworks) of wireless communications into different kinds of deep learning algorithms for URLLC. We first introduce the background of URLLC and review promising network architectures and deep learning frameworks in 6G. To better illustrate how to improve learning algorithms with theoretical knowledge, we revisit model-based analysis tools and cross-layer optimization frameworks for URLLC. Following that, we examine the potential of applying supervised/unsupervised deep learning and deep reinforcement learning in URLLC and summarize related open problems. Finally, we provide simulation and experimental results to validate the effectiveness of different learning algorithms and discuss future directions.
更多查看译文
AI 理解论文
溯源树
样例
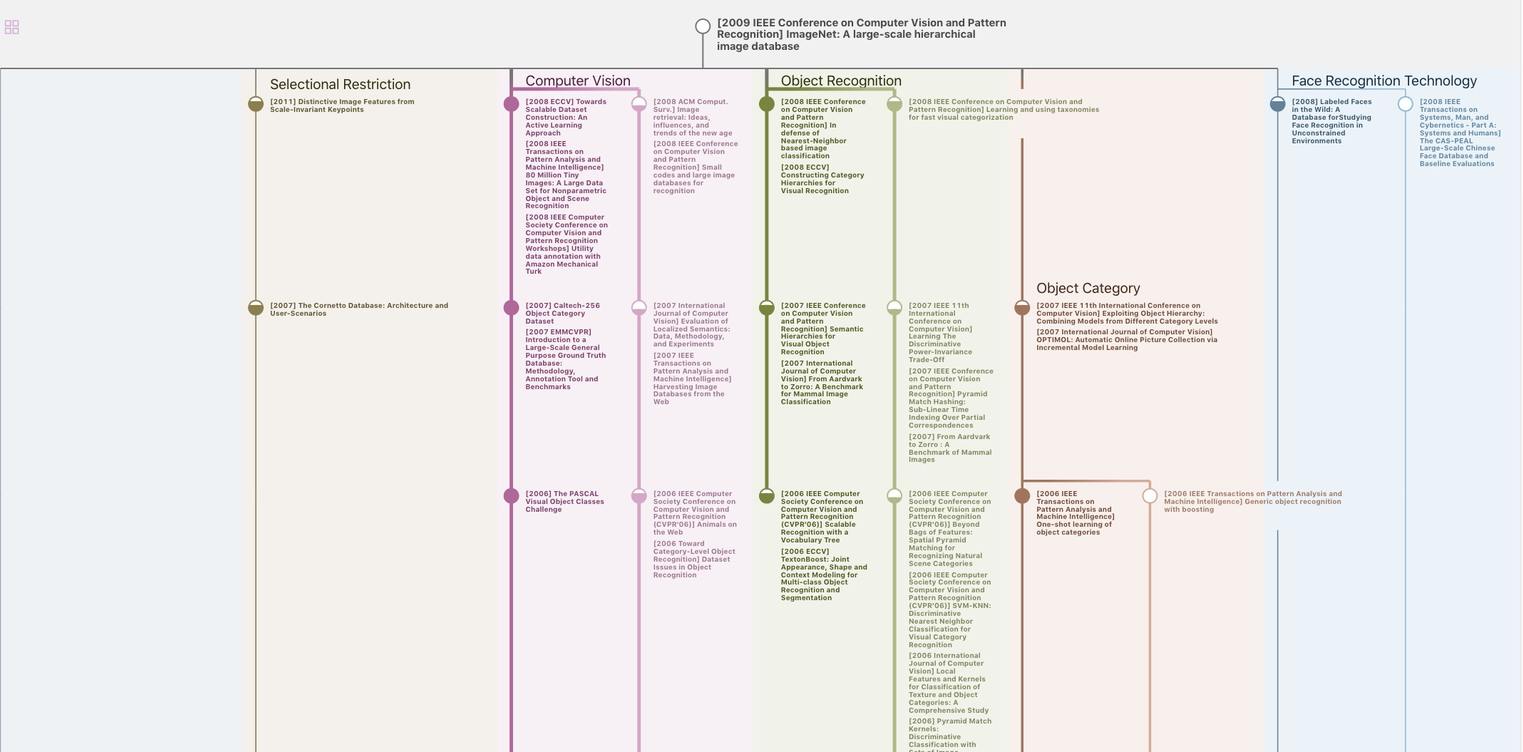
生成溯源树,研究论文发展脉络
Chat Paper
正在生成论文摘要