Application of hierarchical clustering to multi-parametric MR in prostate: Differentiation of tumor and normal tissue with high accuracy
Magnetic Resonance Imaging(2020)
摘要
Purpose: Hierarchical clustering (HC), an unsupervised machine learning (ML) technique, was applied to multi-parametric MR (mp-MR) for prostate cancer (PCa). The aim of this study is to demonstrate HC can diagnose PCa in a straightforward interpretable way, in contrast to deep learning (DL) techniques.Methods: HC was constructed using mp-MR including intravoxel incoherent motion, diffusion kurtosis imaging, and dynamic contrast-enhanced MRI from 40 tumor and normal tissues in peripheral zone (PZ) and 23 tumor and normal tissues in transition zone (TZ). HC model was optimized by assessing the combinations of several dissimilarity and linkage methods. Goodness of HC model was validated by internal methods.Results: Accuracy for differentiating tumor and normal tissue by optimal HC model was 96.3% in PZ and 97.8% in TZ, comparable to current clinical standards. Relationship between input (DWI and permeability parameters) and output (tumor and normal tissue cluster) was shown by heat maps, consistent with literature.Conclusion: HC can accurately differentiate PCa and normal tissue, comparable to state-of-the-art diffusion based parameters. Contrary to DL techniques, HC is an operator-independent ML technique producing results that can be interpreted such that the results can be knowledgeably judged.
更多查看译文
关键词
Hierarchical clustering,Cluster analysis,Machine learning,Interpretability,Prostate cancer,Multi-parametric magnetic resonance imaging
AI 理解论文
溯源树
样例
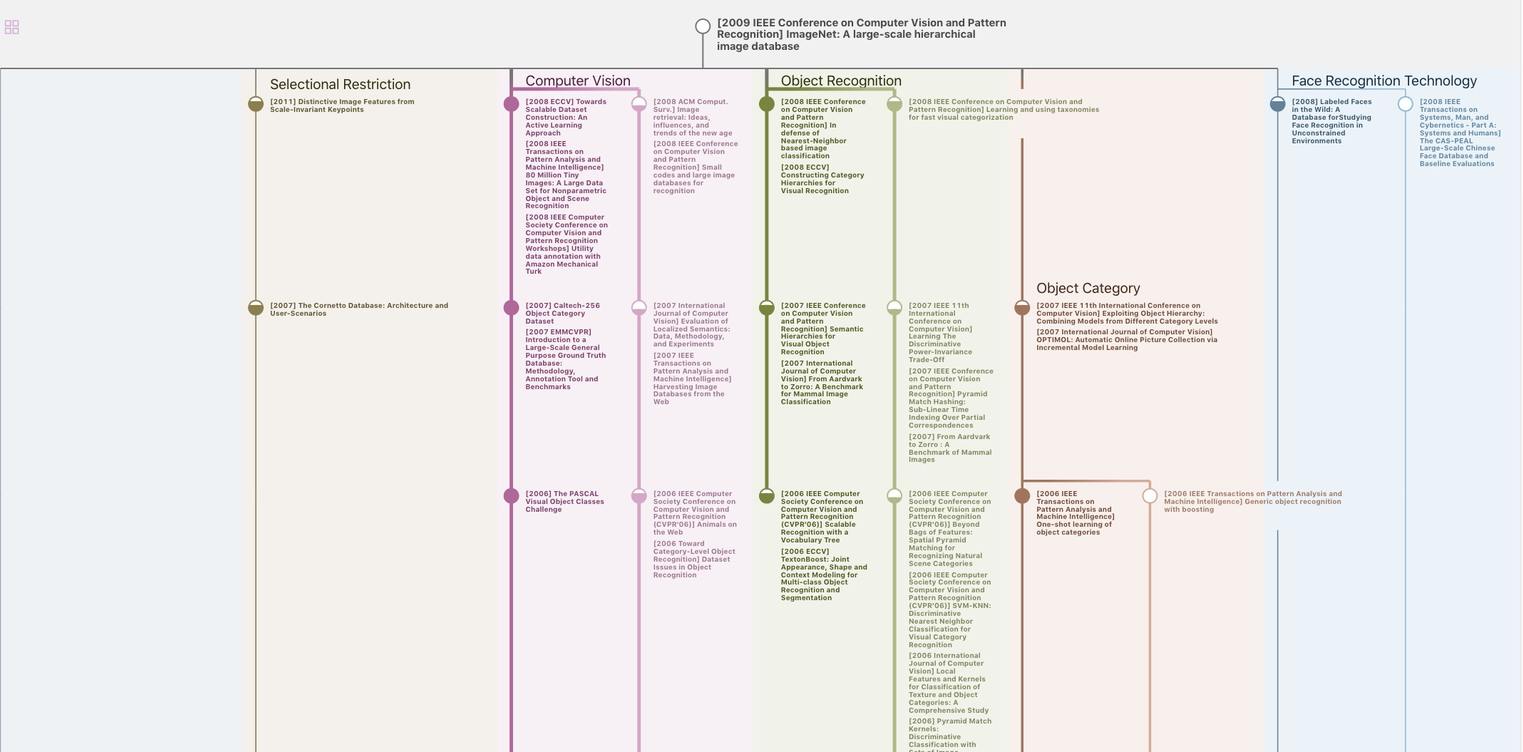
生成溯源树,研究论文发展脉络
Chat Paper
正在生成论文摘要