Data-Driven Advancement of Homogeneous Nickel Catalyst Activity for Aryl Ether Cleavage
ACS CATALYSIS(2020)
摘要
The increasing urgency to make chemical processes more environmentally friendly while continuing to derive the chemicals required for modern society from renewable resources requires the development of a forthcoming generation of synthetic processes and the catalysts needed to facilitate these reactions. Recently, applications of machine-learning (ML) algorithms involving catalysis have begun to appear with increasing frequency, as they constitute an attractive pathway both for discovering prospective species and identifying trends surrounding catalytic behavior, principally because the number of potential catalysts that can be examined greatly exceeds those found in more traditional experimental or theoretical approaches. Here, we harness a data-driven approach powered by ML in tandem with molecular volcano plots to estimate the activity of over 143,000 homogeneous nickel catalysts bearing phosphine and N-heterocyclic carbene ligands for the reductive C(sp(2))-O cleavage reaction in aryl ether compounds, an important step in the degradation of biomass (lignin) into industrially useful feedstock chemicals. Our computational workflow reveals that a vast majority of Ni-phosphine and Ni-carbene catalysts are not ideally tuned to facilitate this reaction. An analysis of those species identified as being the most promising uncovers a clear catalytic design strategy that can be exploited in an experimental setting to enhance the rate of reductive C(sp(2))-O cleavage of aryl ether compounds.
更多查看译文
关键词
homogeneous catalysis,machine-learning,big data,lignin valorization,volcano plots,linear scaling relationships,ligand design
AI 理解论文
溯源树
样例
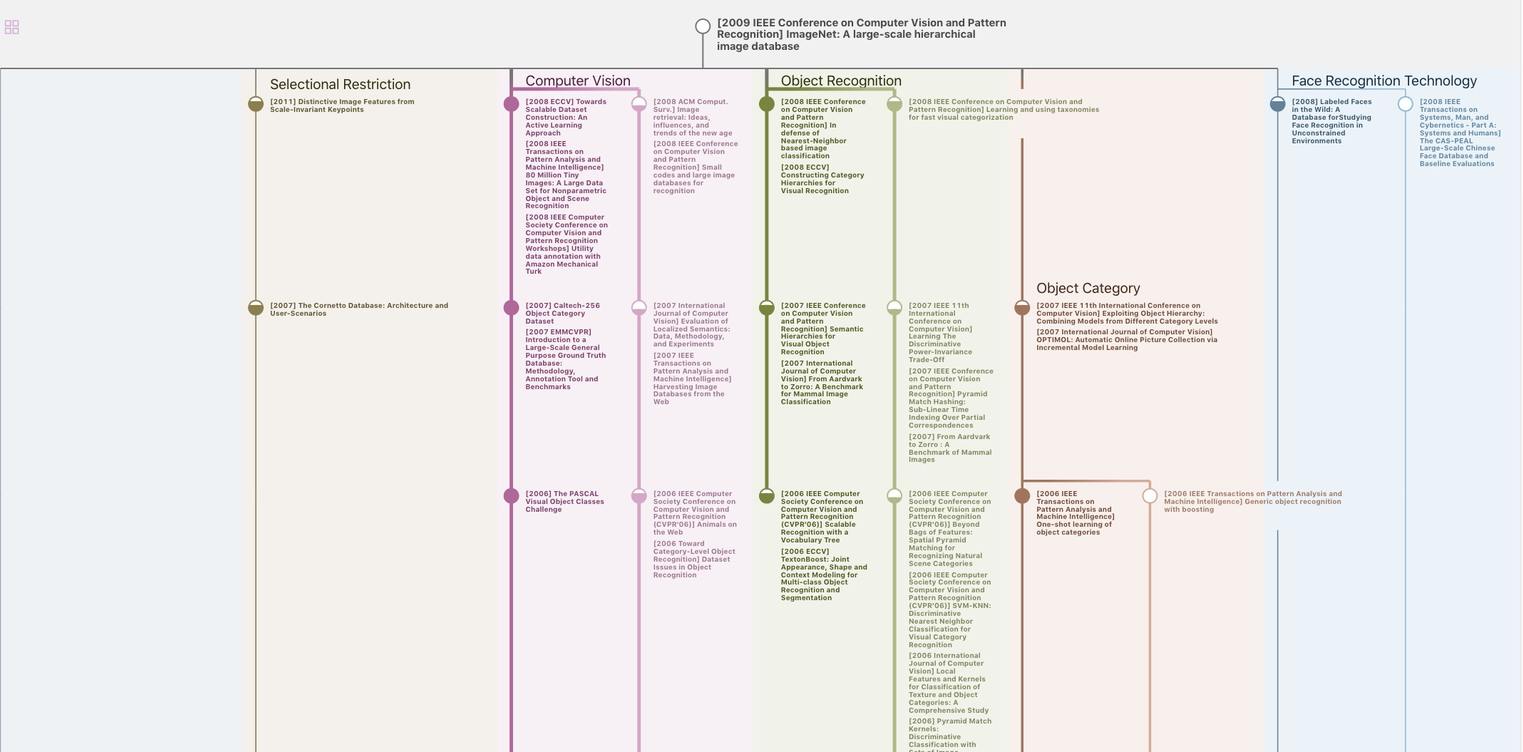
生成溯源树,研究论文发展脉络
Chat Paper
正在生成论文摘要