Bayesian Efficient Coding
biorxiv(2024)
摘要
The efficient coding hypothesis, which proposes that neurons are optimized to maximize information about the environment, has provided a guiding theoretical framework for sensory and systems neuroscience. More recently, a theory known as the Bayesian Brain hypothesis has focused on the brain’s ability to integrate sensory and prior sources of information in order to perform Bayesian inference. However, there is as yet no comprehensive theory connecting these two theoretical frameworks. We bridge this gap by formalizing a Bayesian theory of efficient coding. We define Bayesian efficient codes in terms of four basic ingredients: (1) a stimulus prior distribution; (2) an encoding model; (3) a capacity constraint, specifying a neural resource limit; and (4) a loss function, quantifying the desirability or undesirability of various posterior distributions. Classic efficient codes can be seen as a special case in which the loss function is the posterior entropy, leading to a code that maximizes mutual information, but alternate loss functions give solutions that differ dramatically from information-maximizing codes. In particular, we show that decorrelation of sensory inputs, which is optimal under classic efficient codes in low-noise settings, can be disadvantageous for loss functions that penalize large errors. Bayesian efficient coding therefore enlarges the family of normatively optimal codes and provides a more general framework for understanding the design principles of sensory systems. We examine Bayesian efficient codes for linear receptive fields and nonlinear input-output functions, and show that our theory invites reinterpretation of Laughlin’s seminal analysis of efficient coding in the blowfly visual system.
### Competing Interest Statement
The authors have declared no competing interest.
更多查看译文
AI 理解论文
溯源树
样例
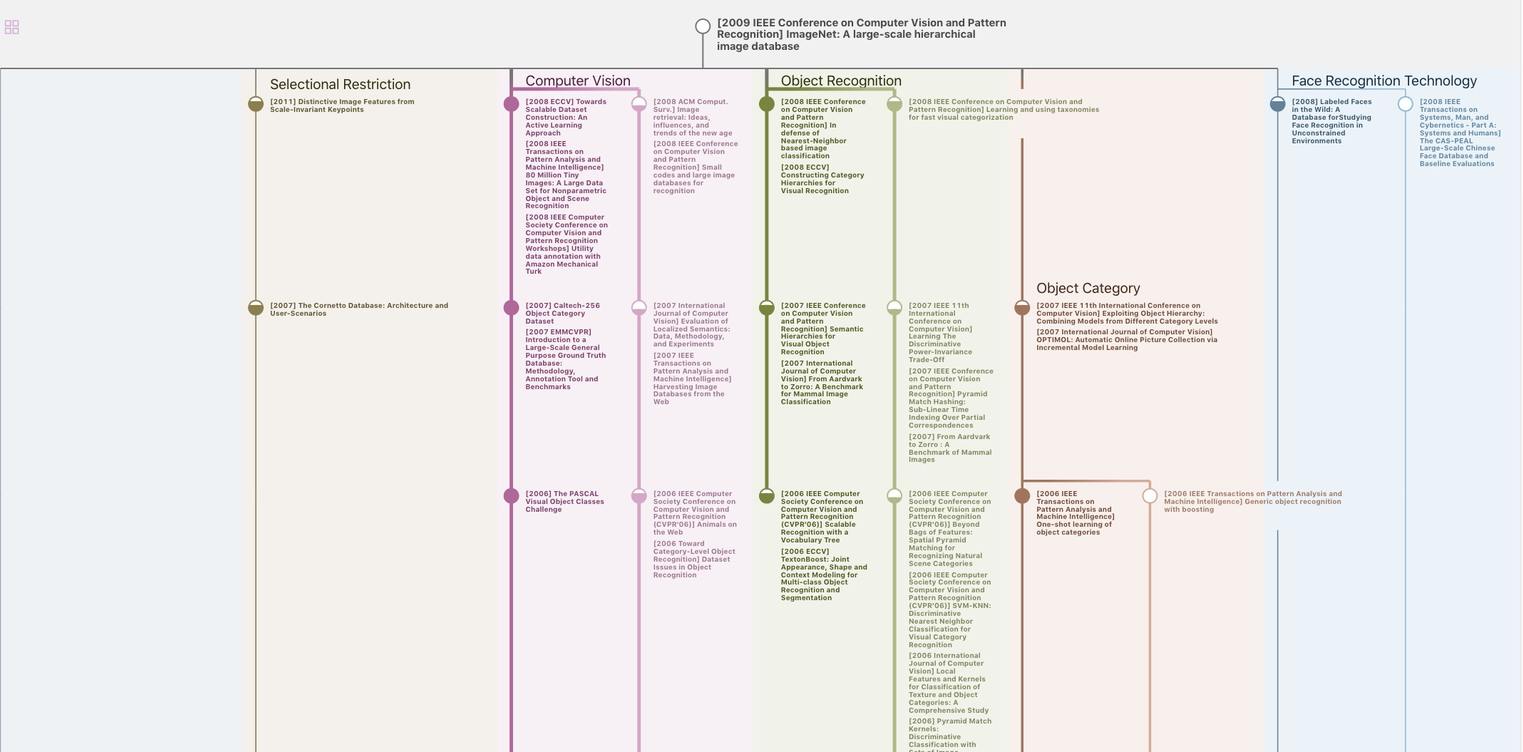
生成溯源树,研究论文发展脉络
Chat Paper
正在生成论文摘要